Wi-Prox: Proximity Estimation of Non-Directly Connected Devices via Sim2Real Transfer Learning.
Global Communications Conference(2023)
摘要
Recent years have witnessed an increasing number of mobile devices, posing a more diversified demand for device localization solutions. While existing wireless localization solutions can obtain the relative locations of connected devices, they fall short in estimating the spatial relationships between devices that are not directly connected. To address this technical gap, we propose Wi-Prox, the first proximity estimation system for non-directly connected devices. Wi-Prox evaluates the spatial proximity of two devices by analyzing their received wireless signals. It integrates a novel multi-resolution spatial encoder that extracts multi-scale spatial features from complex-valued wireless signals, which are then analyzed and transformed into a domain-adaptive proximity metric. To enhance the general-izability of Wi-Prox, we adopt a simulation-to-reality transfer learning framework. Wi-Prox is pre-trained with a large amount of simulated data and then fine-tuned for real-world deployment, significantly reducing the need for real-world data collection. We implement Wi-Prox and evaluate its performance in both simulated and real environments. Our results indicate that a fine-tuned Wi-Prox achieves an average accuracy of 97.2% in selecting the most proximate device. Even without fine-tuning, a pre-trained Wi-Prox still manages an average accuracy of 93.8%, thereby demonstrating impressive performance in terms of both proximity estimation accuracy and domain generalizability.
更多查看译文
关键词
Transfer Learning,Sim2real Transfer,Simulated Data,Large Amount Of Data,Spatial Features,Real-world Data,Simulation Environment,Relative Location,Radio Waves,Need For Collection,Need For Data Collection,Transfer Learning Framework,Optimization Process,Indoor Environments,Generalization Capability,Residual Block,Building Structures,Complex Domain,Small Amount Of Data,Domain Adaptation,User Equipment,Pre-training Process,Fine-tuned Model,Wireless Devices,Latent Representation,Top-1 Accuracy,Real-world Environments,Fine-tuning Process,Minimum Amount Of Data,Spatial Feature Extraction
AI 理解论文
溯源树
样例
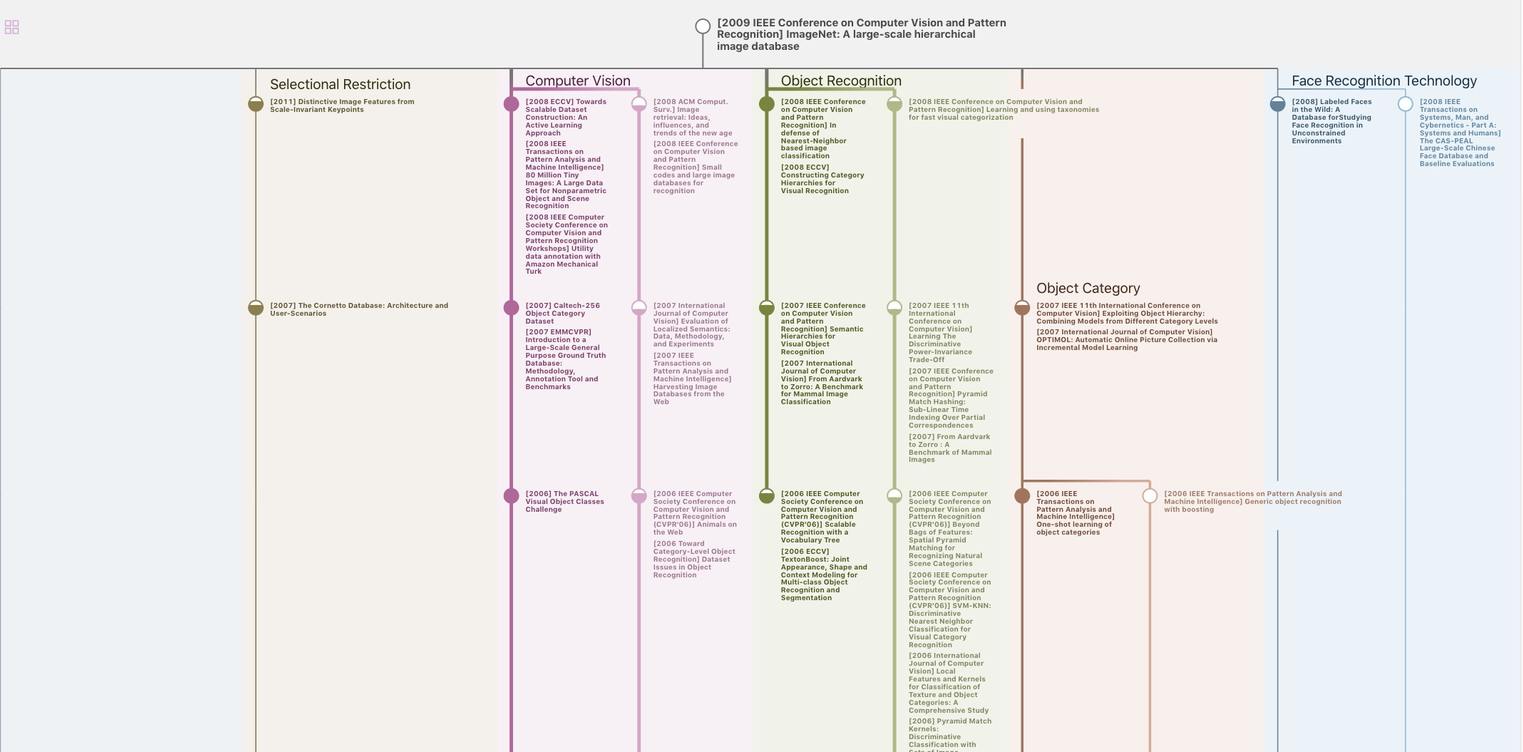
生成溯源树,研究论文发展脉络
Chat Paper
正在生成论文摘要