A Deep Reinforcement Learning Approach for the Placement of Scalable Microservices in the Edge-to-Cloud Continuum.
Global Communications Conference(2023)
摘要
The recent proliferation of computing paradigms, and among them the more prominent ones at the two endpoints of the network infrastructure, i.e. the edge and the cloud, paves the way for an edge-to-cloud continuum of resources and services that is able to meet the stringent Quality of Service (QoS) requirements of emerging applications. However, deploying modern microservice-based applications on such highly distributed and heterogeneous edge-to-cloud infrastructure is a complex challenge to be addressed. To overcome this challenge, we jointly investigate the placement and load distribution problems for applications composed of dependent microservices that can be deployed and scaled across the continuum. Furthermore, we propose a Deep Reinforcement Learning (DRL) based solution for solving this joint problem and we demonstrate through simulations that our proposal outperforms baseline methods in terms of QoS satisfaction and deployment cost.
更多查看译文
关键词
edge-to-cloud continuum,microservice placement,load distribution,deep reinforcement learning
AI 理解论文
溯源树
样例
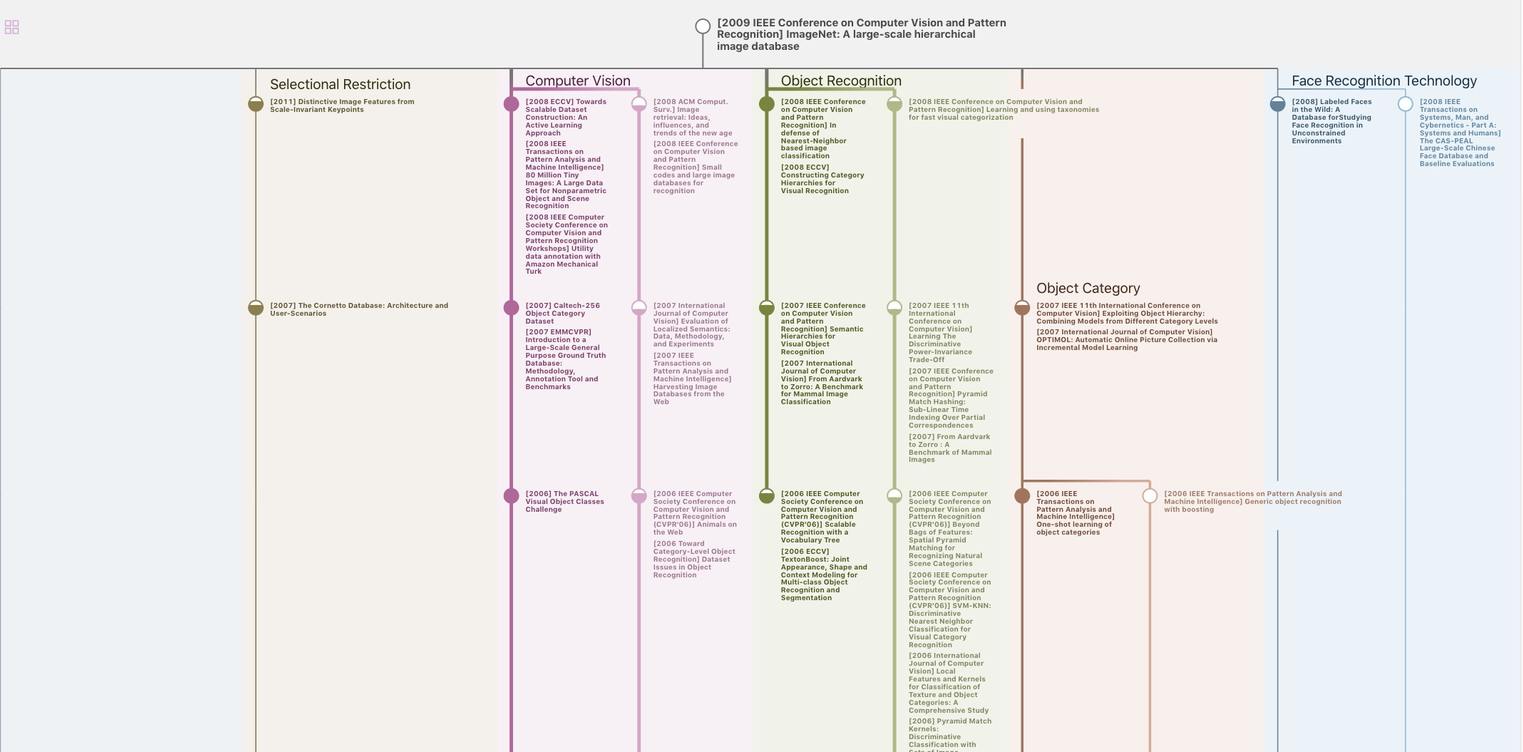
生成溯源树,研究论文发展脉络
Chat Paper
正在生成论文摘要