Towards Efficient and Privacy-Preserving Federated Learning for HMM Training.
Global Communications Conference(2023)
摘要
The hidden Markov model (HMM) has played a pivotal role in various IoT applications due to its ability to model time-varying sequences. Since the datasets usually live in isolated islands and their privacy naturally demands to be seriously considered, the HMM should be trained in a privacy-preserving manner. A typical HMM training framework is federated learning, in which a federated server and many data owners collaboratively train an HMM without revealing data owners' data to the federated server and the trained model to data owners. Since existing HMM training schemes are computationally intensive, we propose an efficient and privacy-preserving federated learning scheme for HMM training to address the efficiency issue in this paper. First, we transform all HMM training computations into matrices- and vectors-based computations over real domains. Then, we introduce our federated HMM training scheme by applying matrix encryption to protect the HMM training privacy. After that, we show that our scheme is privacy-preserving through a rigorous analysis on the security of our scheme. We illustrate that our scheme is efficient through extensive experimental evaluation on the performance of our scheme.
更多查看译文
关键词
Federated learning,Hidden Markov model,Baum-Welch algorithm,Matrix encryption
AI 理解论文
溯源树
样例
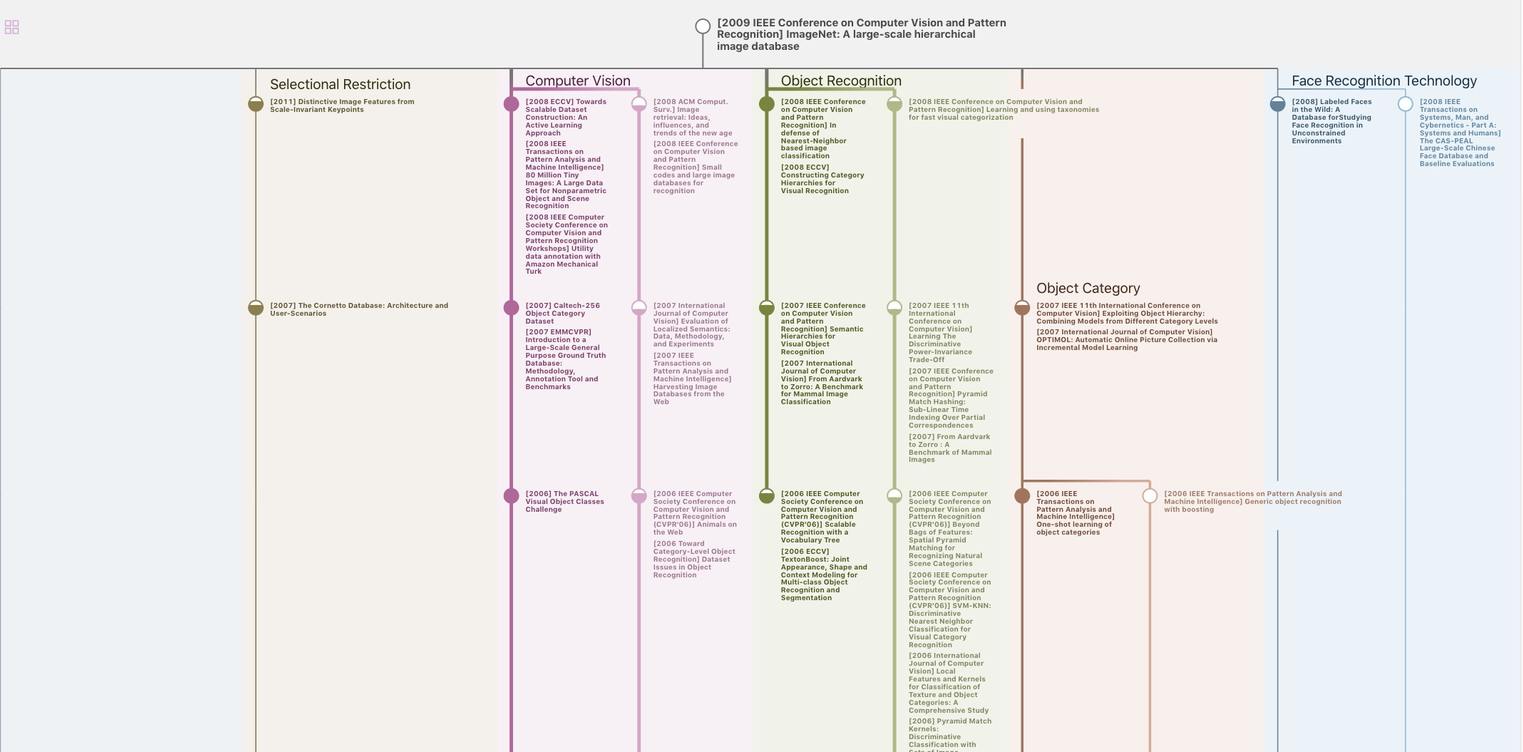
生成溯源树,研究论文发展脉络
Chat Paper
正在生成论文摘要