Prediction of sugar content of fresh peaches based on LDBN model using NIR spectroscopy
Journal of Food Measurement and Characterization(2024)
摘要
Sugar content is a critical factor in evaluating the quality of fresh peaches. This paper aims to develop a rapid and accurate prediction method for the sugar content of fresh peaches using Deep Learning combined with near-infrared spectroscopy (NIRS). The proposed model consists of three main steps. Firstly, the Multivariate Scattering Correction (MSC) is employed as a preprocessing technique to remove noise and redundant information from the raw NIR images of peaches which ensures the reliability of the subsequent analysis. Secondly, the Competitive Adaptive Reweighted Sampling (CARS) is utilized for bands selection which can effectively eliminate redundant information from the NIR spectral data and reduces computational complexity. Finally, a novel Linear Deep Belief Network (LDBN) model is constructed to predict the sugar content of fresh peaches. To validate the performance of the proposed method, a Fresh Peaches Near-infrared spectroscopy dataset (FPNIRS) containing sugar content information is constructed. The maximum value for the sugar content of fresh peaches was 13.4 and the minimum value was 7.5 in the collection of 180 fresh peach samples. The experimental results demonstrate that the proposed LDBN achieves the best prediction performance, with the determination coefficient of prediction set ( R_P^2 ) value of 0.9346 and the root mean square error of prediction set (RMSEP) value of 0.4409. This study suggests that the combination of Near-infrared spectroscopy technology and the LDBN provides an effective method for accurately predicting the sugar content of fresh peaches.
更多查看译文
关键词
Sugar content,Deep learning,Near-infrared spectroscopy,Linear deep belief network
AI 理解论文
溯源树
样例
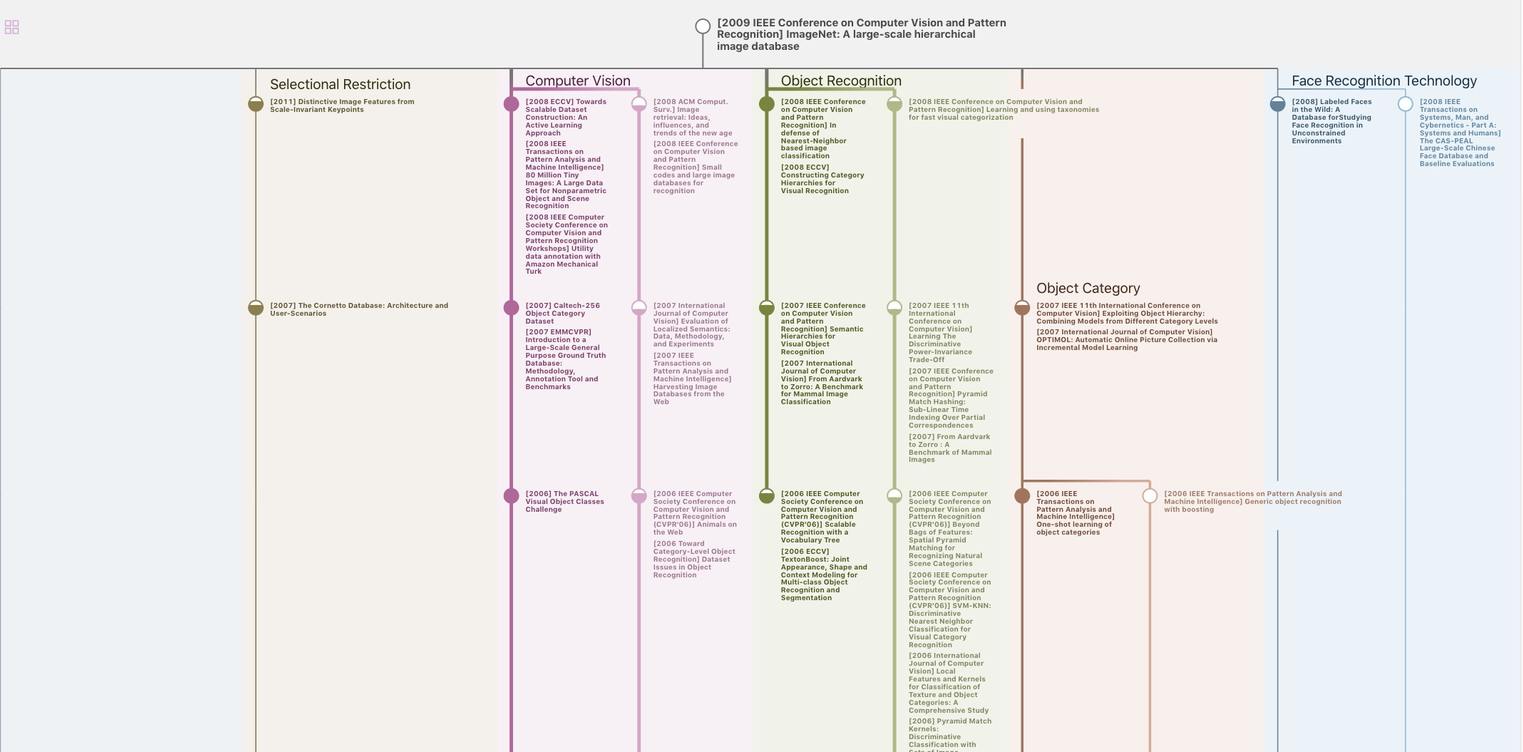
生成溯源树,研究论文发展脉络
Chat Paper
正在生成论文摘要