Predicting Risk of Heroin Overdose, Remission, Use, and Mortality Using Ensemble Learning Methods in a Cohort of People with Heroin Dependence
International Journal of Mental Health and Addiction(2024)
摘要
Despite decades of research demonstrating the effectiveness of treatments for heroin dependence, rates of heroin use, dependence, and death have dramatically increased over the past decade. While evidence has highlighted a range of risk and protective factors for relapse, remission, and other outcomes, this presents clinicians with the challenge as to how to synthesise and integrate the evolving evidence-base to guide clinical decision-making and facilitate the provision of personalised healthcare. Using data from the 11-year follow-up of the Australian Treatment Outcome Study (ATOS), we aimed to develop a clinical risk prediction model to assist clinicians calculate the risk of a range of heroin-related outcomes at varying follow-up intervals for their clients based on known risk factors. Between 2001 and 2002, 615 people with heroin dependence were recruited as part of a prospective longitudinal cohort study. An ensemble machine learning approach was applied to predict risk of heroin use, remission, overdose, and mortality at 1-, 5-, and 10 + year post-study entry. Variables most consistently ranked in the top 10 in terms of their level of importance across outcomes included age; age first got high, used heroin, or injected; sexual trauma; years of school completed; prison history; severe mental health disability; past month criminal involvement; and past month benzodiazepine use. This study provides clinically relevant information on key risk factors associated with heroin use, remission, non-fatal overdose, and mortality among people with heroin dependence, to help guide clinical decision-making in the selection and tailoring of interventions to ensure that the ‘ right treatment ’ is delivered to the ‘ right person ’ at the ‘ right time ’ .
更多查看译文
关键词
Heroin dependence,Risk prediction,Outcome,Remission,Relapse,Mortality
AI 理解论文
溯源树
样例
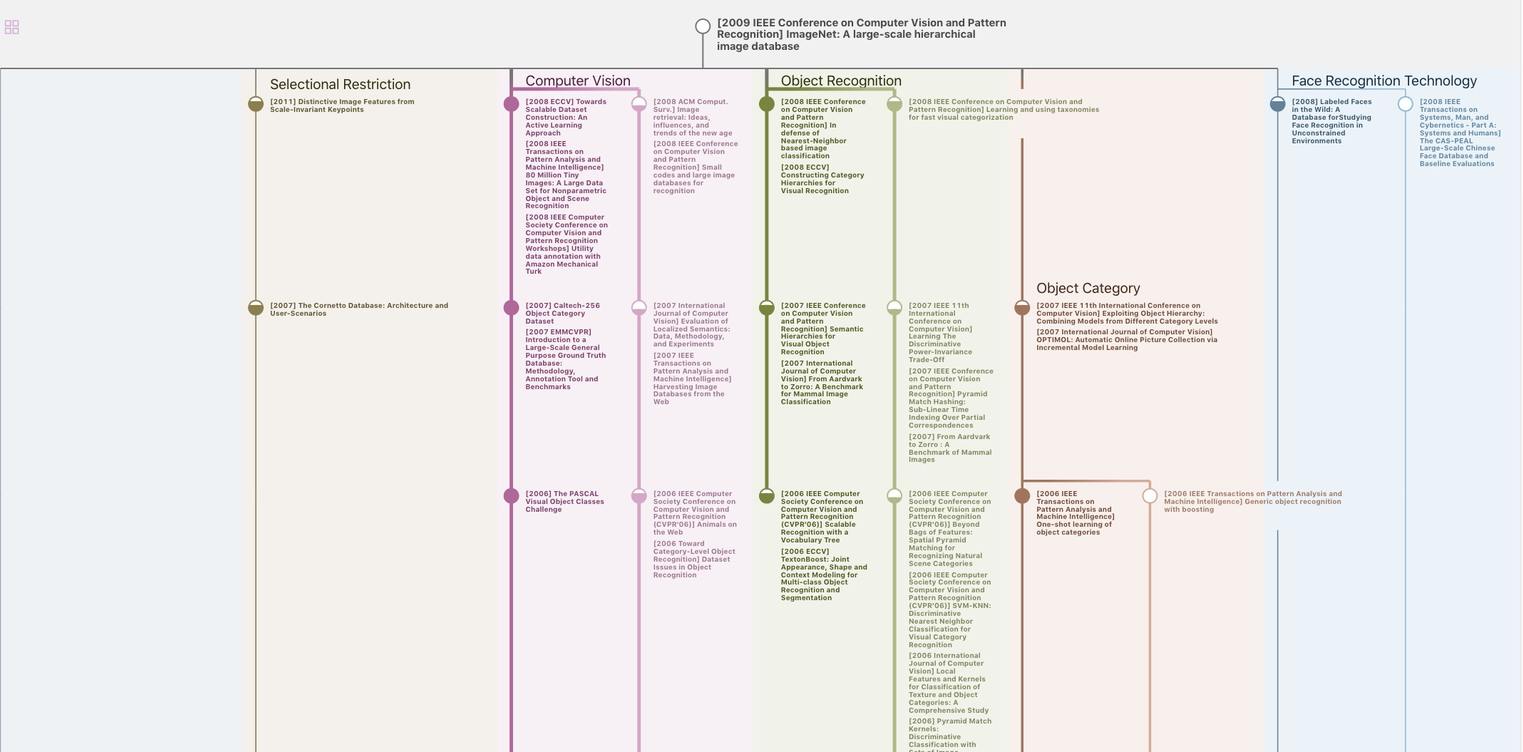
生成溯源树,研究论文发展脉络
Chat Paper
正在生成论文摘要