Decentralized Federated Unlearning on Blockchain
CoRR(2024)
摘要
Blockchained Federated Learning (FL) has been gaining traction for ensuring
the integrity and traceability of FL processes. Blockchained FL involves
participants training models locally with their data and subsequently
publishing the models on the blockchain, forming a Directed Acyclic Graph
(DAG)-like inheritance structure that represents the model relationship.
However, this particular DAG-based structure presents challenges in updating
models with sensitive data, due to the complexity and overhead involved. To
address this, we propose Blockchained Federated Unlearning (BlockFUL), a
generic framework that redesigns the blockchain structure using Chameleon Hash
(CH) technology to mitigate the complexity of model updating, thereby reducing
the computational and consensus costs of unlearning tasks.Furthermore, BlockFUL
supports various federated unlearning methods, ensuring the integrity and
traceability of model updates, whether conducted in parallel or serial. We
conduct a comprehensive study of two typical unlearning methods, gradient
ascent and re-training, demonstrating the efficient unlearning workflow in
these two categories with minimal CH and block update operations. Additionally,
we compare the computation and communication costs of these methods.
更多查看译文
AI 理解论文
溯源树
样例
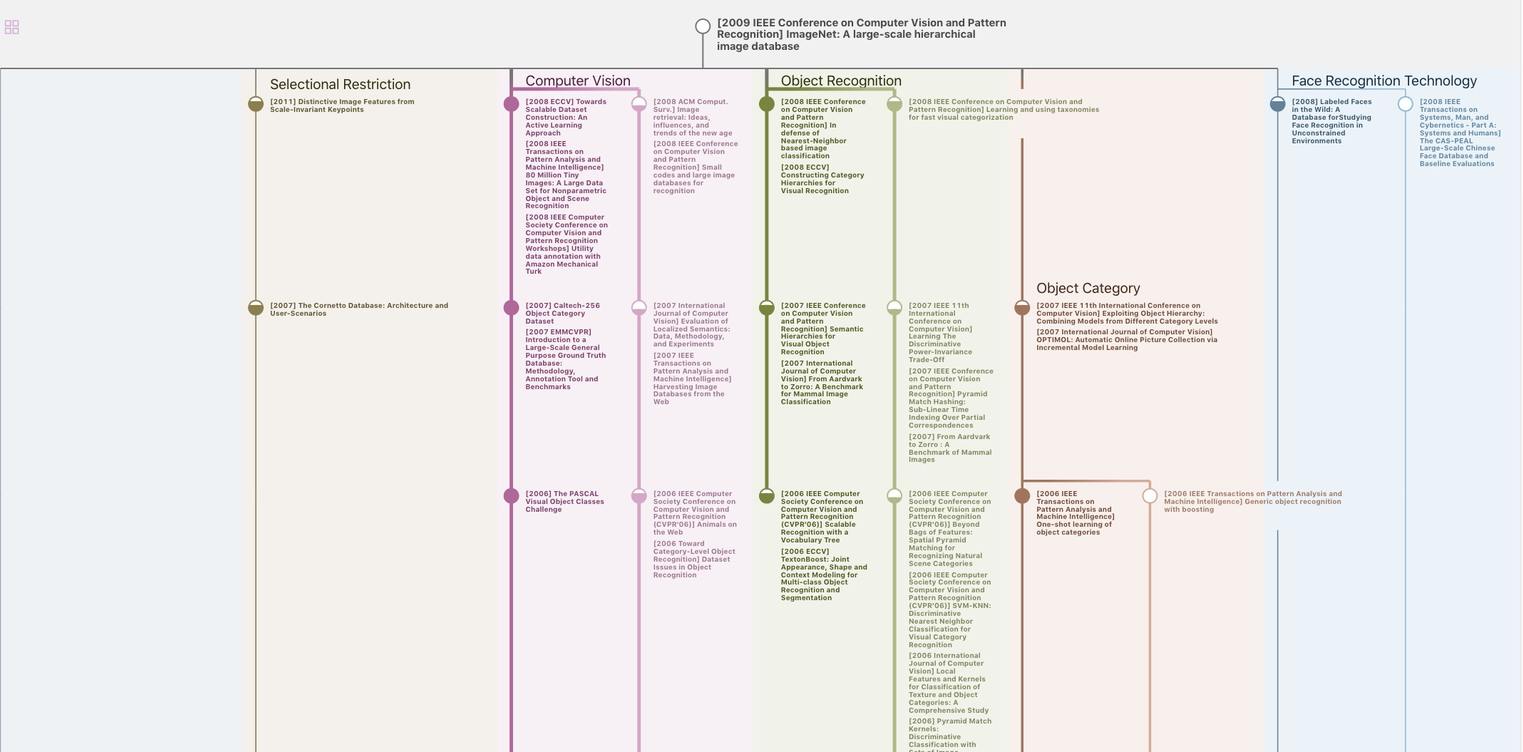
生成溯源树,研究论文发展脉络
Chat Paper
正在生成论文摘要