Rethinking the Role of Scale for In-Context Learning: An Interpretability-based Case Study at 66 Billion Scale.
CoRR(2022)
摘要
Language models have been shown to perform better with an increase in scale on a wide variety of tasks via the in-context learning paradigm. In this paper, we investigate the hypothesis that the ability of a large language model to in-context learn-perform a task is not uniformly spread across all of its underlying components. Using a 66 billion parameter language model (OPT-66B) across a diverse set of 14 downstream tasks, we find this is indeed the case: similar to 70% of the attention heads and similar to 20% of the feed forward networks can be removed with minimal decline in task performance. We find substantial overlap in the set of attention heads (un)important for incontext learning across tasks and number of in-context examples. We also address our hypothesis through a task-agnostic lens, finding that a small set of attention heads in OPT-66B score highly on their ability to perform primitive induction operations associated with incontext learning, namely, prefix matching and copying. These induction heads overlap with task-specific important heads, reinforcing arguments by Olsson et al. (2022) regarding induction head generality to more sophisticated behaviors associated with in-context learning. Overall, our study provides several insights that indicate large language models may be undertrained for in-context learning and opens up questions on how to pre-train language models to more effectively perform in-context learning.
更多查看译文
AI 理解论文
溯源树
样例
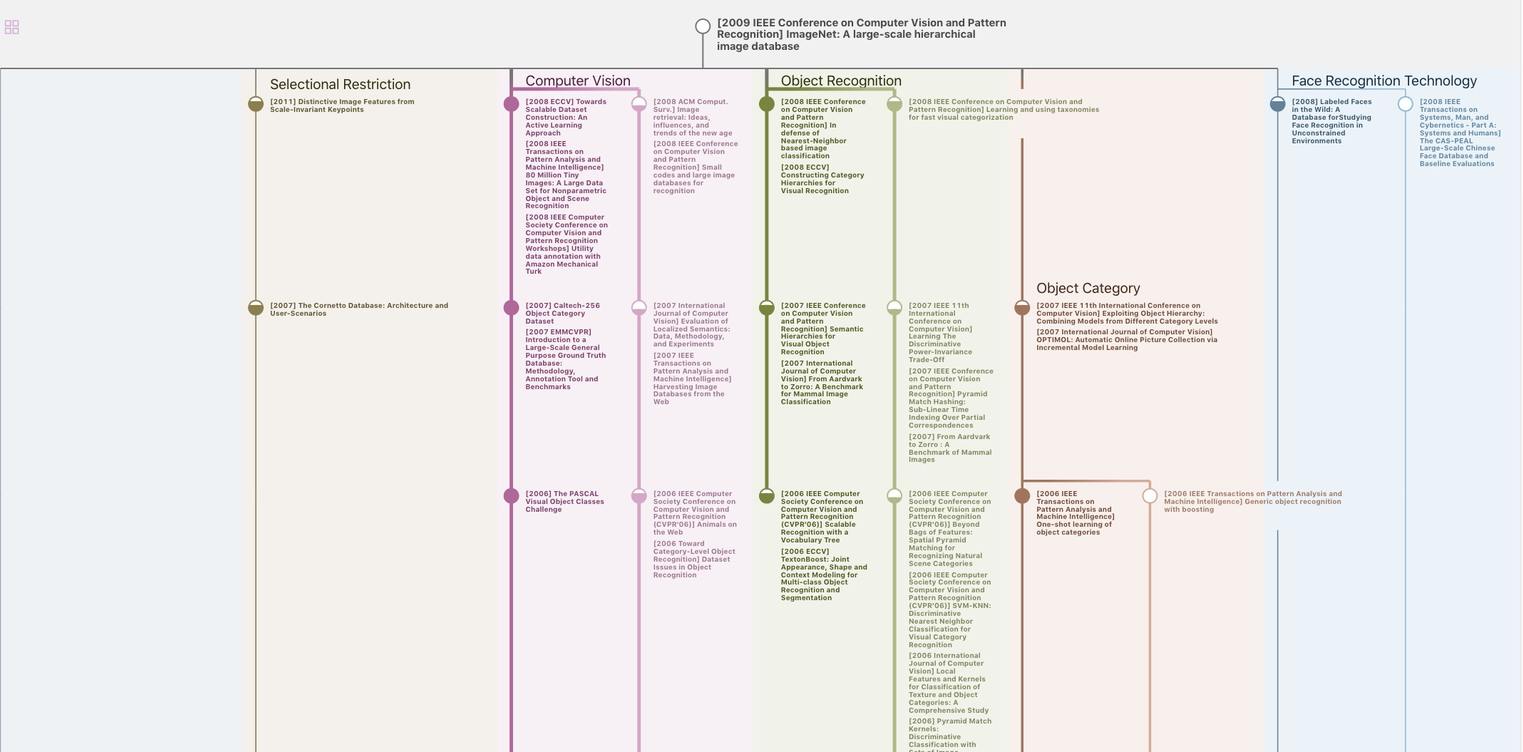
生成溯源树,研究论文发展脉络
Chat Paper
正在生成论文摘要