An embedded TensorFlow lite model for classification of chip images with respect to chip morphology depending on varying feed
Journal of Intelligent Manufacturing(2024)
摘要
Turning is one of the fundamental machining processes used to produce superior machine parts. It is critical to manage the machining conditions to maintain the desired properties of the final product. Chip morphology and chip control are crucial factors to be monitored. In particular, the selection of an appropriate feed has one of the most significant effects. On the other hand, machine learning is an advanced approach that is continuously evolving and helping many industries. Moreover, mobile applications with learning models have been deployed in the field, recently. Taking these motivations into account, in this study, we propose a practical mobile application that includes an embedded learning model to provide chip classification based on chip morphology. For this purpose, a dataset of chips with different morphological properties is obtained and manually labeled according to ISO 3685 standards by using 20 different feeds on AISI 4140 material. Accordingly, TensorFlow Lite is used to train a learning model, and the model is embedded into a real-time Android mobile application. Eventually, the final software is evaluated through experiments conducted on the dataset and in the field, respectively. According to the evaluation results, it can be stated that the learning model is able to predict chip morphology with a test accuracy of 85.4
更多查看译文
关键词
Turning,Machine learning,Chip morphology,TensorFlow lite,Mobile application
AI 理解论文
溯源树
样例
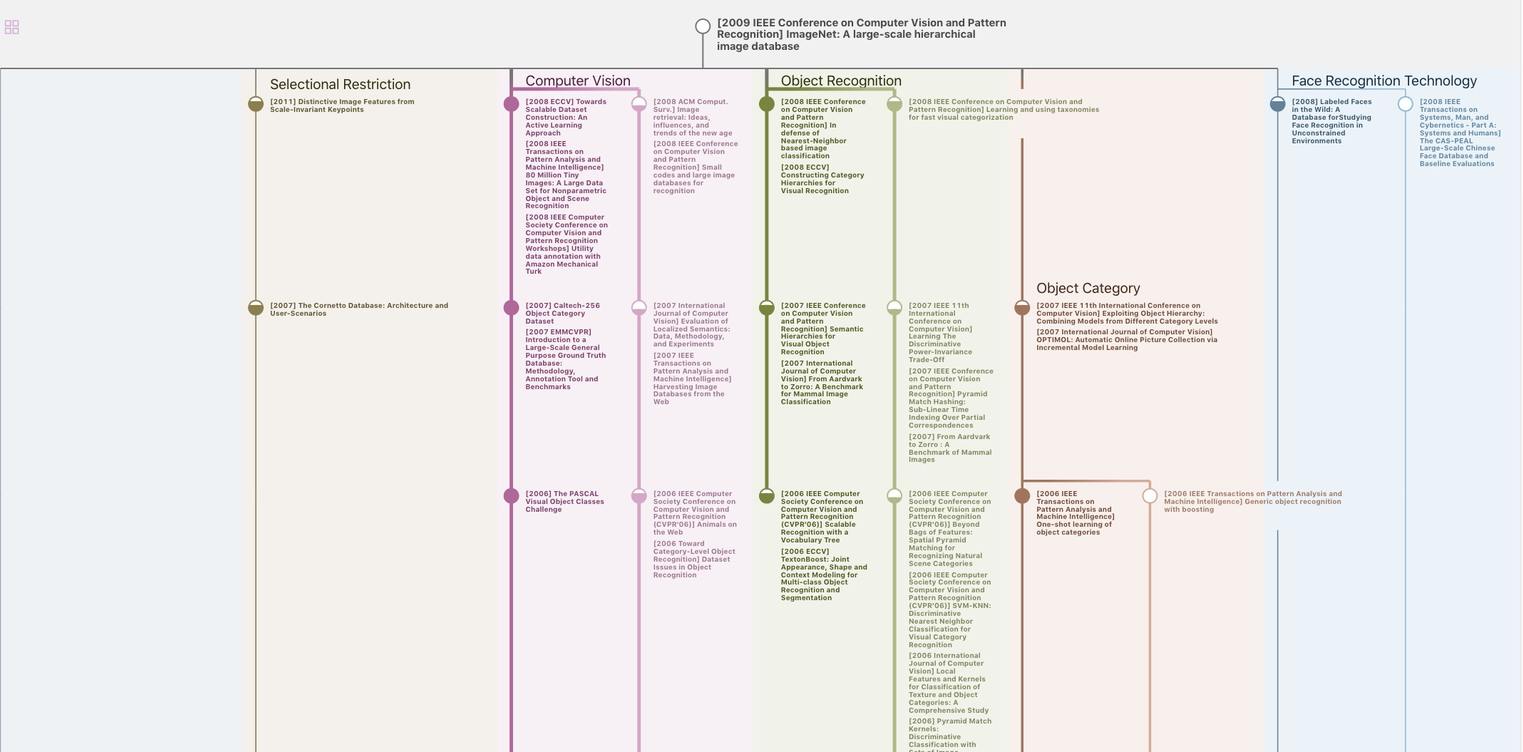
生成溯源树,研究论文发展脉络
Chat Paper
正在生成论文摘要