A Soft Sensor for Multirate Quality Variables Based on MC-CNN
IEEE TRANSACTIONS ON NEURAL NETWORKS AND LEARNING SYSTEMS(2024)
摘要
In recent years, data-driven soft sensor modeling methods have been widely used in industrial production, chemistry, and biochemical. In industrial processes, the sampling rates of quality variables are always lower than those of process variables. Meanwhile, the sampling rates among quality variables are also different. However, few multi-input multi-output (MIMO) sensors take this temporal factor into consideration. To solve this problem, a deep-learning (DL) model based on a multitemporal channels convolutional neural network (MC-CNN) is proposed. In the MC-CNN, the network consists of two parts: the shared network used to extract the temporal feature and the parallel prediction network used to predict each quality variable. The modified BP algorithm makes the blank values generated at unsampled moments not participate in the backpropagation (BP) process during training. By predicting multiple quality variables of two industrial cases, the effectiveness of the proposed method is verified.
更多查看译文
关键词
Feature extraction,Soft sensors,Predictive models,MIMO communication,Correlation,Convolutional neural networks,Training,Convolutional neural network (CNN),deep learning (DL),multirate sampling,quality variables,soft sensor
AI 理解论文
溯源树
样例
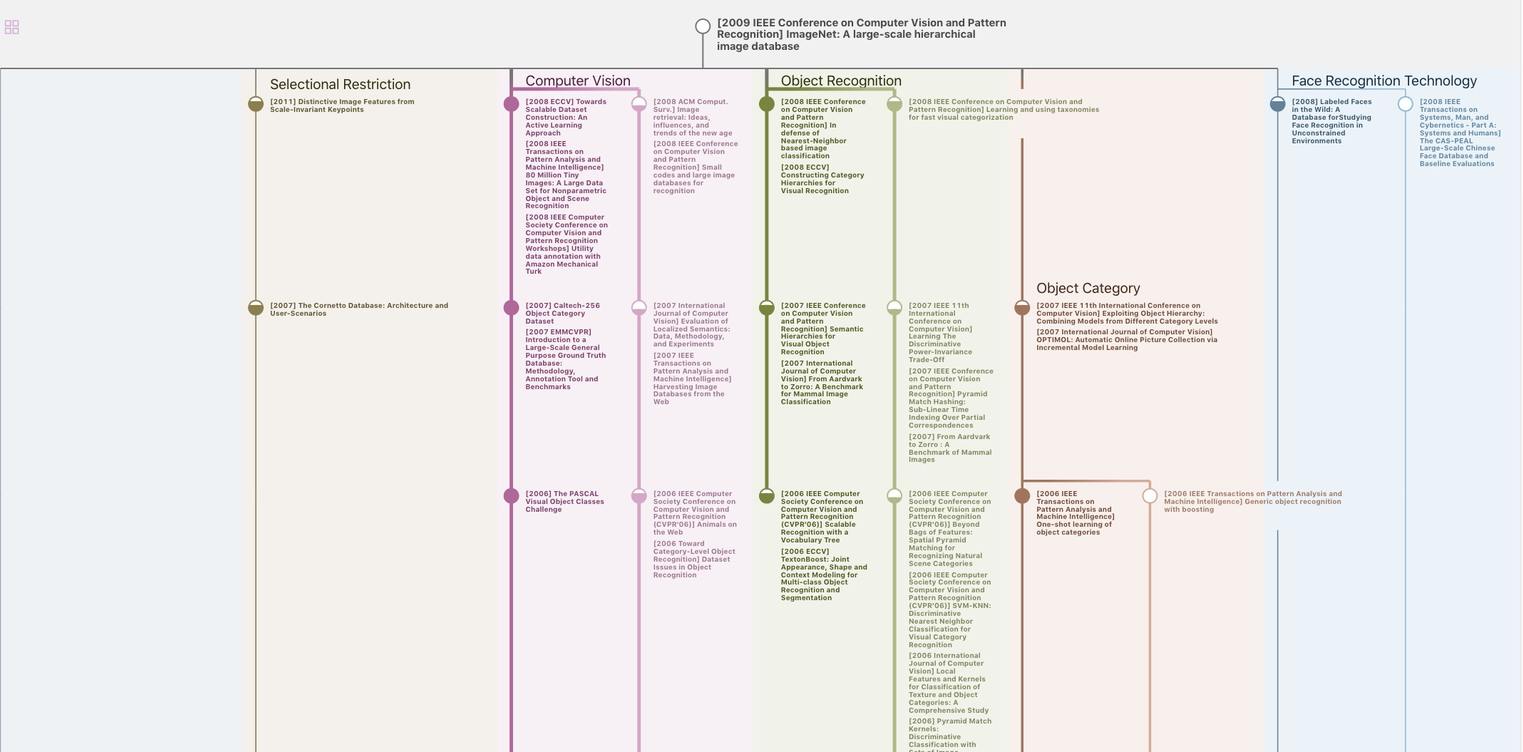
生成溯源树,研究论文发展脉络
Chat Paper
正在生成论文摘要