TTSR: A Transformer-Based Topography Neural Network for Digital Elevation Model Super-Resolution
IEEE TRANSACTIONS ON GEOSCIENCE AND REMOTE SENSING(2024)
摘要
Digital elevation models (DEMs) are crucial geographical data sources, whereas the resolution of commonly used DEM products is low and cannot meet the requirement of detailed geo-related applications. Deep learning-based methods have demonstrated to be effective in super-resolution (SR) techniques, which reconstruct high-resolution (HR) images from low-resolution (LR) images. However, existing deep learning methods have not fully considered the multiscale spatial heterogeneity and topographic knowledge of DEM data that differentiate them from traditional images. These defects inevitably lead to the localized smoothing of the reconstructed DEM and influence the reliability of downstream geographical analysis. This study proposes a transformer-based topography neural network (TTSR) for DEM SR incorporating a local-global deformable block (LGDB) for capturing the multiscale spatial heterogeneity and topographic knowledge, a spatio-channel coupled channel attention (SimAM) mechanism for reallocating channel weights and providing a supplement of the global spatial features, and an improved terrain loss function (iLoss) for mitigating noise across datasets. TTSR was validated using two publicly available real-world DEM datasets for recovering DEM from 30 to 10 m. The root mean square error (RMSE) of the proposed method was reduced by approximately 6%-30%, 4%-16%, and 1%-9% in elevation accuracy, slope accuracy, and aspect accuracy, respectively, compared to the best one of those state-of-the-art methods. This research provides new insights for improving the accuracy of the DEM SR, which will help generate global HR terrain products for geographical studies in the future.
更多查看译文
关键词
Transformers,Image reconstruction,Task analysis,Feature extraction,Visualization,Interpolation,Computational modeling,Digital elevation models (DEM) super-resolution (SR),multiscale features,neural network,spatial heterogeneity,terrain features,topographic knowledge
AI 理解论文
溯源树
样例
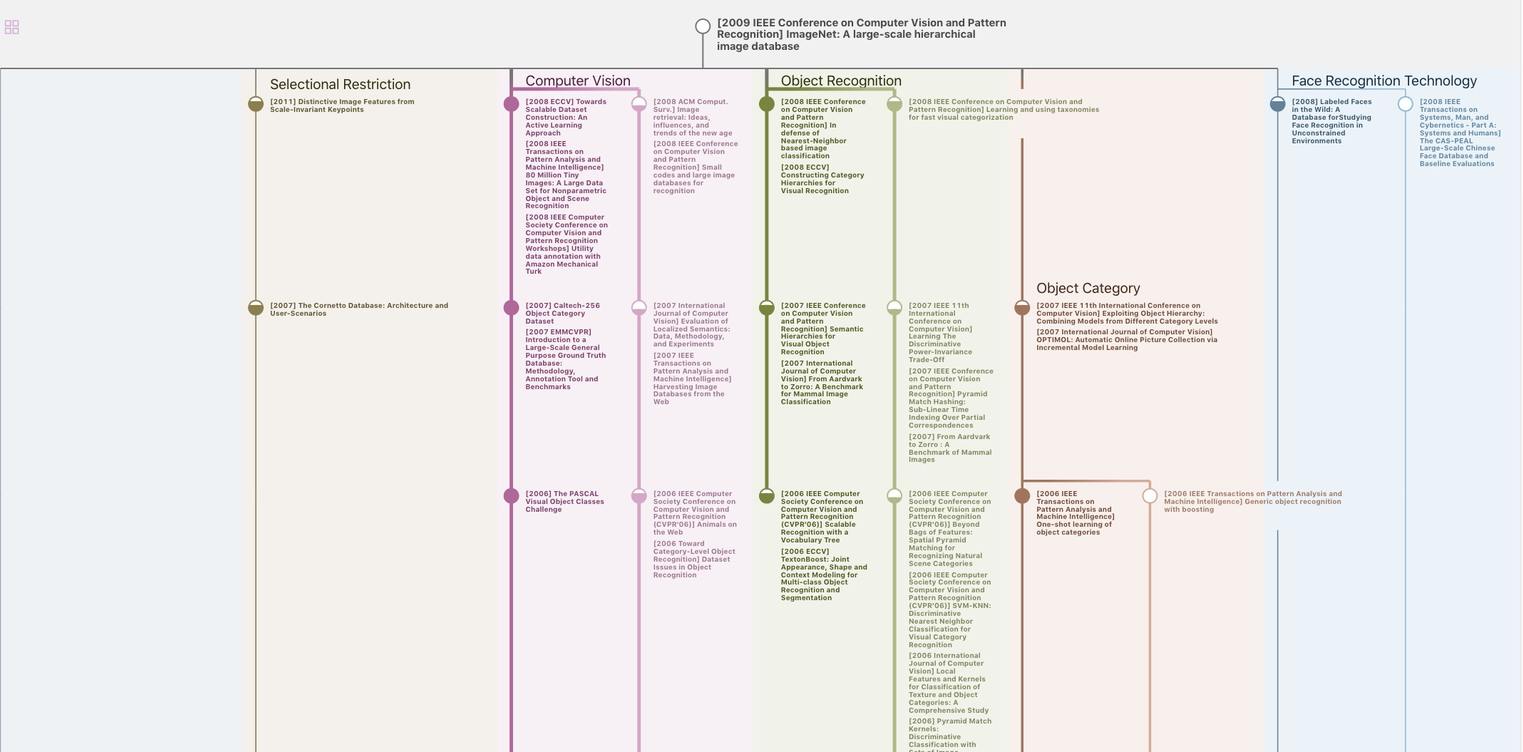
生成溯源树,研究论文发展脉络
Chat Paper
正在生成论文摘要