Machine Learning Integrating Protein Structure, Sequence, and Dynamics to Predict the Enzyme Activity of Bovine Enterokinase Variants.
Journal of chemical information and modeling(2024)
摘要
Despite recent advances in computational protein science, the dynamic behavior of proteins, which directly governs their biological activity, cannot be gleaned from sequence information alone. To overcome this challenge, we propose a framework that integrates the peptide sequence, protein structure, and protein dynamics descriptors into machine learning algorithms to enhance their predictive capabilities and achieve improved prediction of the protein variant function. The resulting machine learning pipeline integrates traditional sequence and structure information with molecular dynamics simulation data to predict the effects of multiple point mutations on the fold improvement of the activity of bovine enterokinase variants. This study highlights how the combination of structural and dynamic data can provide predictive insights into protein functionality and address protein engineering challenges in industrial contexts.
更多查看译文
AI 理解论文
溯源树
样例
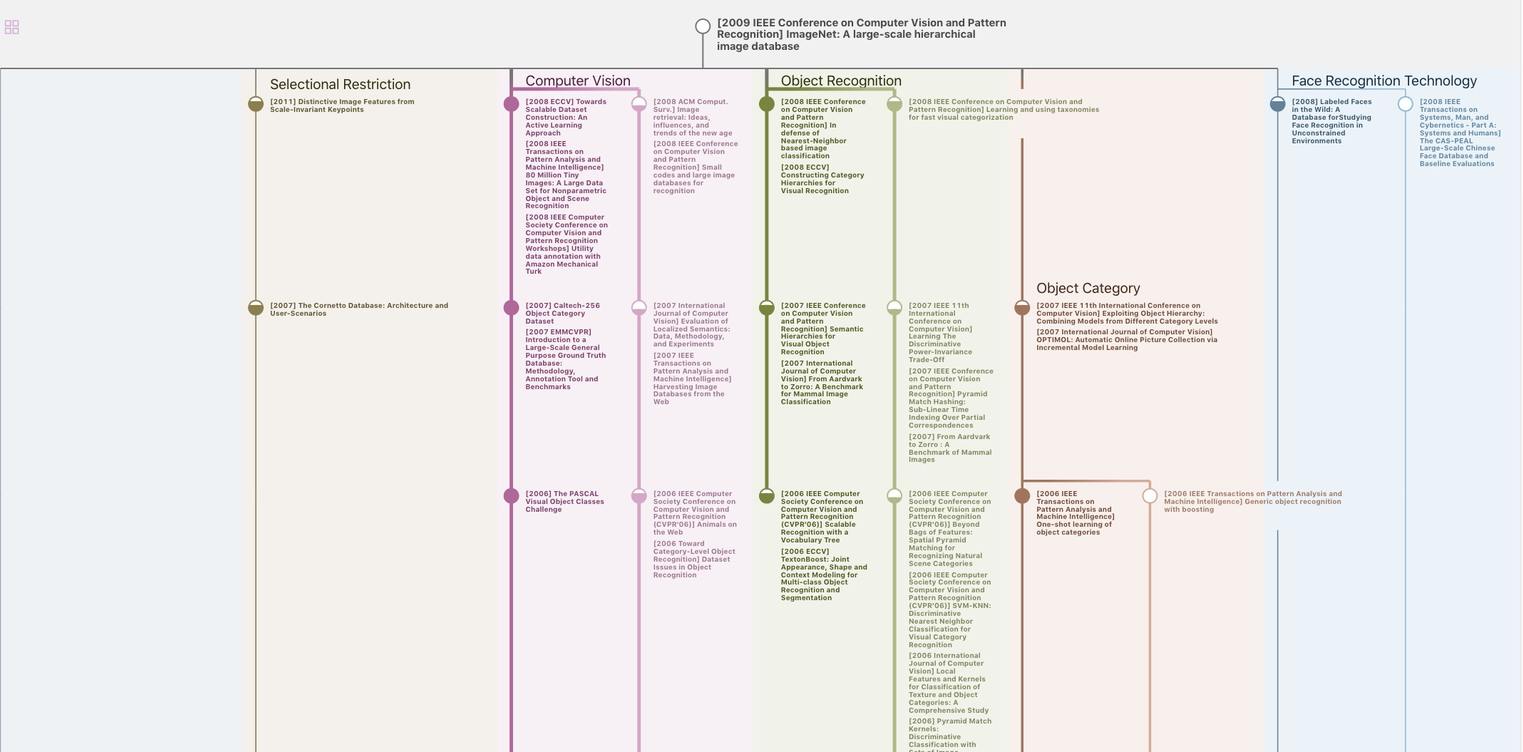
生成溯源树,研究论文发展脉络
Chat Paper
正在生成论文摘要