Enhancing Knee MR Image Clarity through Image Domain Super-Resolution Reconstruction
BIOENGINEERING-BASEL(2024)
摘要
This study introduces a hybrid analytical super-resolution (SR) pipeline aimed at enhancing the resolution of medical magnetic resonance imaging (MRI) scans. The primary objective is to overcome the limitations of clinical MRI resolution without the need for additional expensive hardware. The proposed pipeline involves three key steps: pre-processing to re-slice and register the image stacks; SR reconstruction to combine information from three orthogonal image stacks to generate a high-resolution image stack; and post-processing using an artefact reduction convolutional neural network (ARCNN) to reduce the block artefacts introduced during SR reconstruction. The workflow was validated on a dataset of six knee MRIs obtained at high resolution using various sequences. Quantitative analysis of the method revealed promising results, showing an average mean error of 1.40 +/- 2.22% in voxel intensities between the SR denoised images and the original high-resolution images. Qualitatively, the method improved out-of-plane resolution while preserving in-plane image quality. The hybrid SR pipeline also displayed robustness across different MRI sequences, demonstrating potential for clinical application in orthopaedics and beyond. Although computationally intensive, this method offers a viable alternative to costly hardware upgrades and holds promise for improving diagnostic accuracy and generating more anatomically accurate models of the human body.
更多查看译文
关键词
super-resolution,magnetic resonance images (MRI),image reconstruction,machine learning,convolutional neural networks (CNN)
AI 理解论文
溯源树
样例
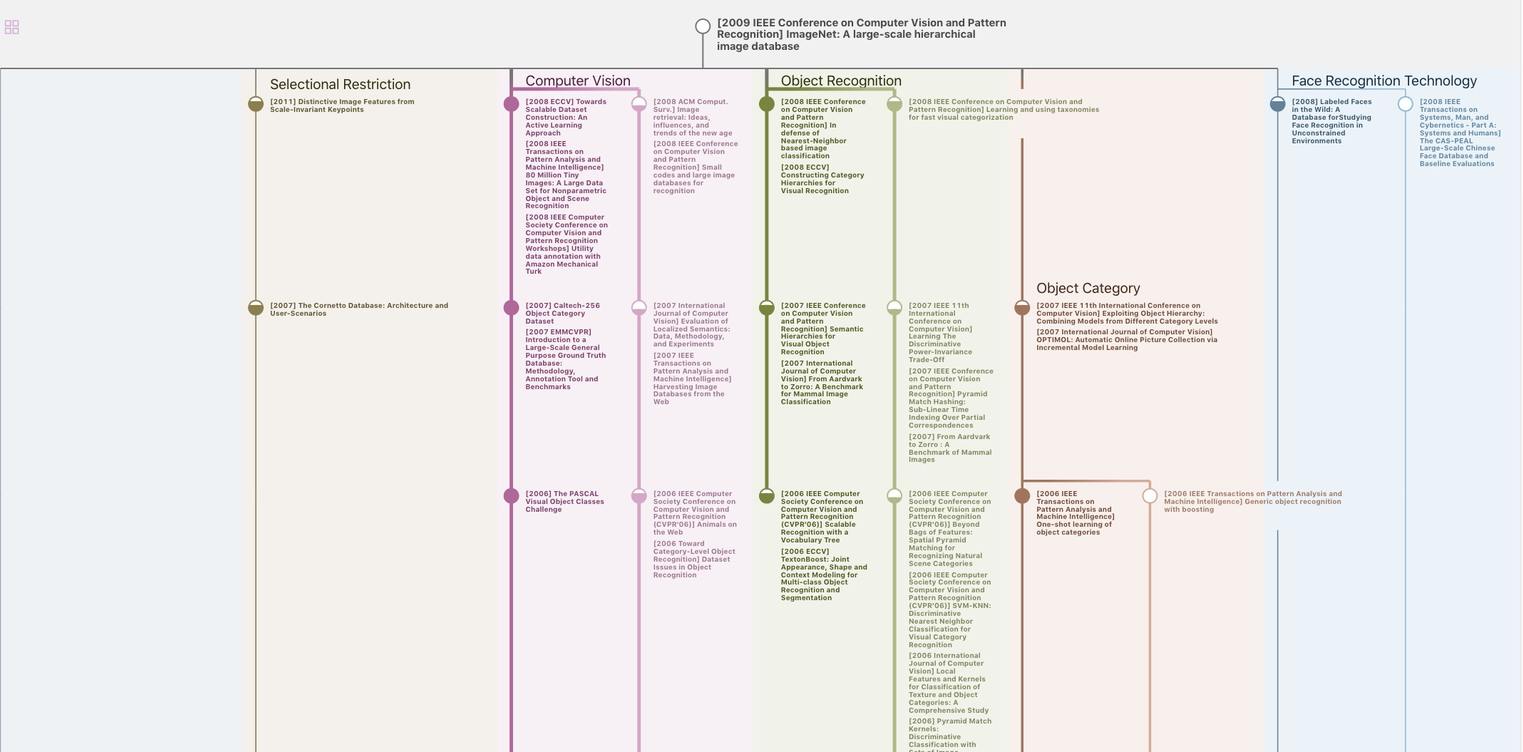
生成溯源树,研究论文发展脉络
Chat Paper
正在生成论文摘要