UnifiedSC: a unified framework via collaborative optimization for multi-task person re-identification
Applied Intelligence(2024)
摘要
Person re-identification (ReID) encompasses two independent study branches, i.e., single-modality and cross-modality identifications. Since single-modality and cross-modality pedestrian data have completely different properties, it is hard to accomplish both tasks at once. However, studying either of the two tasks alone limits the application of person ReID. Therefore, we first explore the relationship between single-modality and cross-modality person ReID and attempt to solve the multi-task optimization problem. To this end, we propose a unified framework, termed UnifiedSC, to mine identity-invariant discriminative features for multi-task person ReID. To effectively optimize the deep model, we construct a collaborative optimization strategy to simultaneously train visible and infrared images from two aspects. On the one hand, two independent classifiers are designed to separately perform single-modality and cross-modality pedestrian identification. On the other hand, we handle the identity-aware feature discrepancy problem at both the feature and classifier levels. At the feature level, we introduce a verification model to distinguish positive/negative sample pairs and employ the weighted regularization triplet to constrain the relative feature distribution. Meanwhile, at the classifier level, we create a shared-weight classifier to map pedestrian features from different domains into a similar feature space. With the promotion of collaborative optimization, the proposed UnifiedSC framework could perceive different pedestrian information and mine identity-invariant features. Our method achieves a mean rank-1 of = 84.7
更多查看译文
关键词
Single-modality,Cross-modality,Multi-task,Person re-identification
AI 理解论文
溯源树
样例
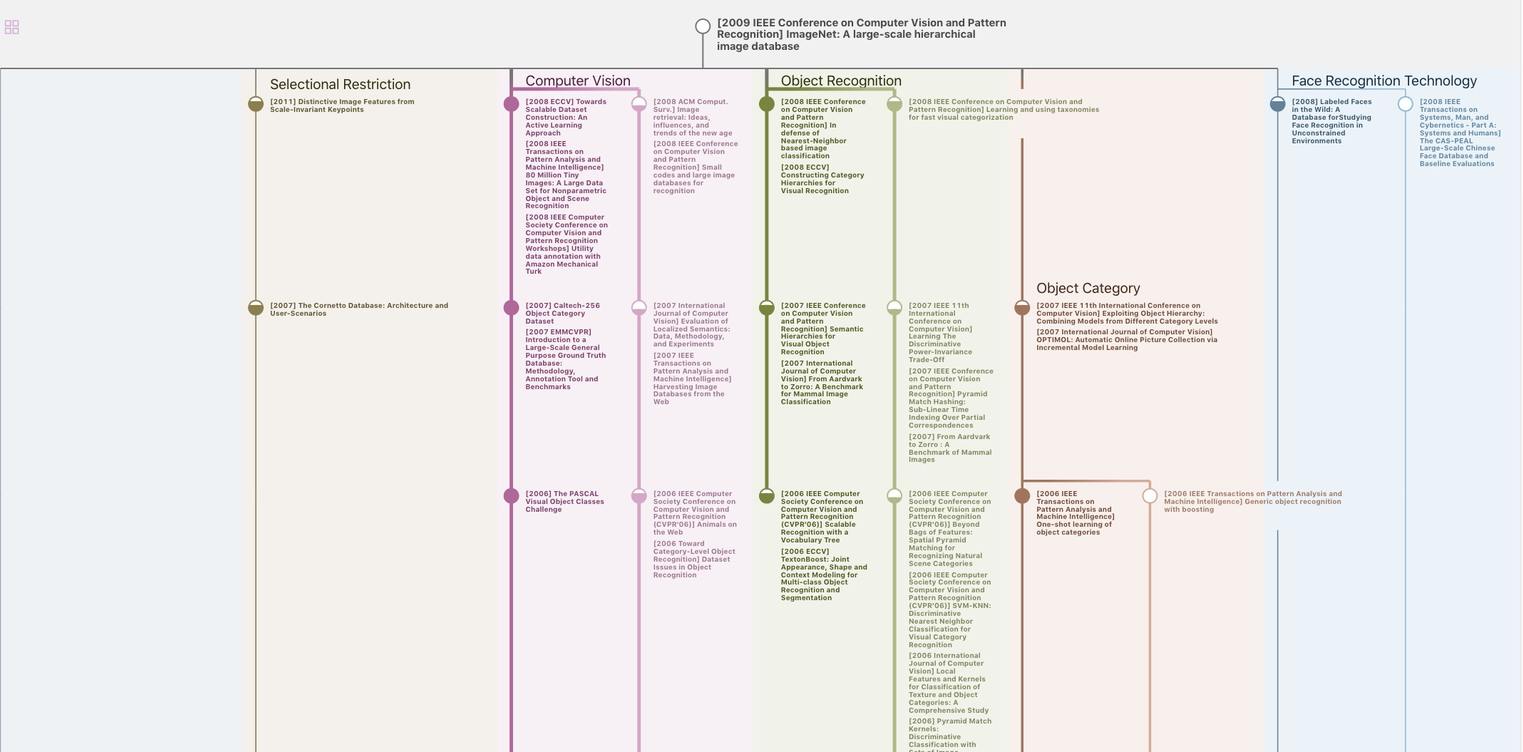
生成溯源树,研究论文发展脉络
Chat Paper
正在生成论文摘要