Unveiling dominant recombination loss in perovskite solar cells with a XGBoost-based machine learning approach
ISCIENCE(2024)
摘要
Remarkable and intelligent perovskite solar cells (PSCs) have attracted substantial attention from researchers and are undergoing rapid advancements in photovoltaic technology. These developments aim to create highly efficient energy devices with fewer dominant recombination losses within the realm of third -generation solar cells. Diverse machine learning (ML) algorithms implemented, addressing dominant losses due to recombination in PSCs, focusing on grain boundaries (GBs), interfaces, and band -to -band recombination. The extreme gradient boosting (XGBoost) classifier effectively predicts the recombination losses. Our model trained with 7 -fold cross -validation to ensure generalizability and robustness. Leveraging Optuna and shapley additive explanations (SHAP) for hyperparameter optimization and investigate the influence of features on target variables, achieved 85% accuracy on over 2 million simulated data, respectively. Because of the input parameters (light intensity and open -circuit voltage), the performance evaluation measures for the dominant losses caused by the recombination predicted by proposed model were superior to those of state-of-the-art models.
更多查看译文
关键词
Machine learning,Electronic engineering,Energy application
AI 理解论文
溯源树
样例
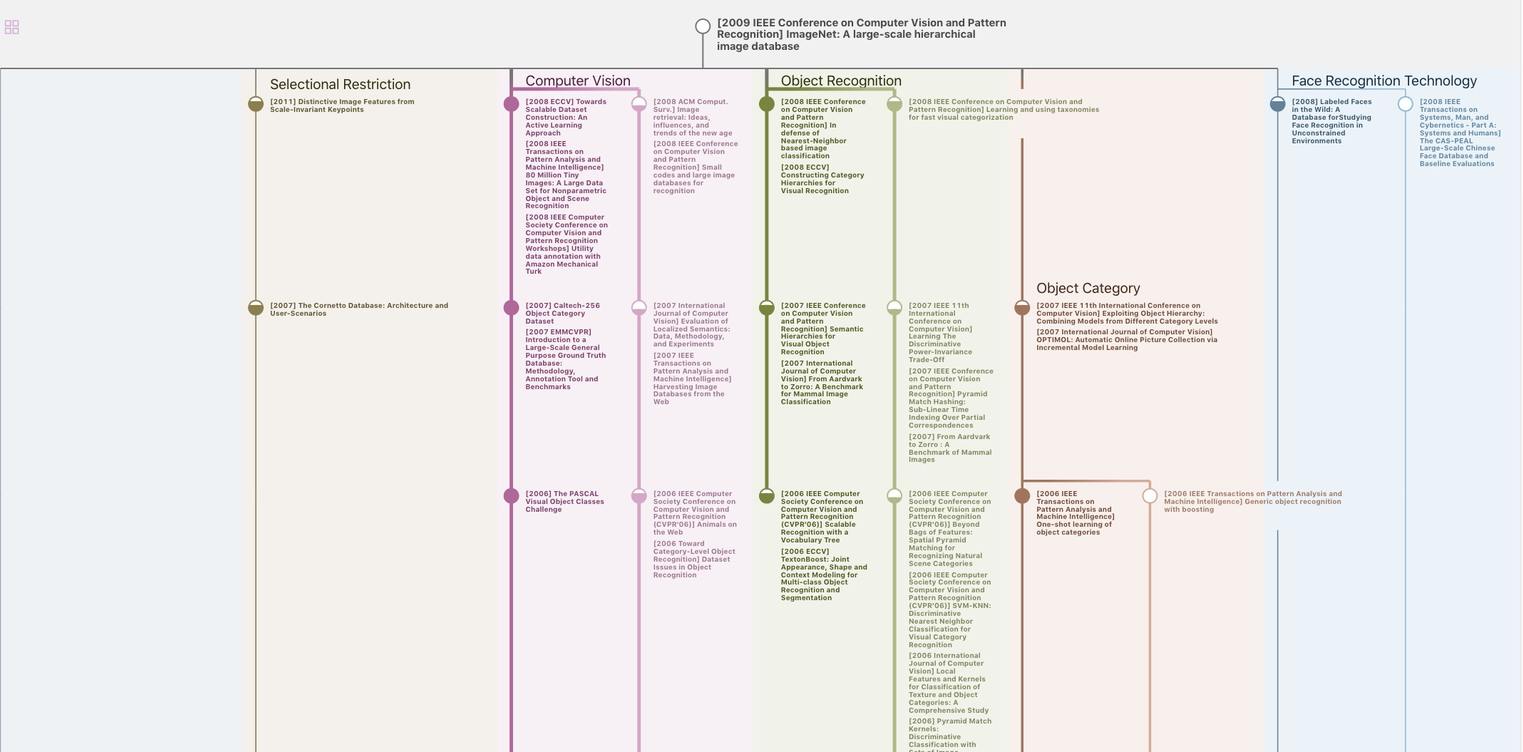
生成溯源树,研究论文发展脉络
Chat Paper
正在生成论文摘要