Fundamental bounds for parameter estimation with few measurements
arxiv(2024)
摘要
Bounding the optimal precision in parameter estimation tasks is of central importance for technological applications. In the regime of a small number of measurements, or that of low signal-to-noise ratios, the meaning of common frequentist bounds such as the Cram\'er-Rao bound (CRB) become questionable. Here, we discuss different linear (Barankin-like) conditions that can be imposed on estimators and analyze when these conditions admit an optimal estimator with finite variance, for any number of measurement repetitions. We show that, if the number of imposed conditions is larger than the number of measurement outcomes, there generally does not exist a corresponding estimator with finite variance. We analyze this result from different viewpoints and examples and elaborate on connections to the shot-noise limit and the Kitaev phase estimation algorithm. We then derive an extended Cram\'er-Rao bound that is compatible with a finite variance in situations where the Barankin bound is undefined. Finally, we show an exemplary numerical confrontation between frequentist and Bayesian approaches to parameter estimation.
更多查看译文
AI 理解论文
溯源树
样例
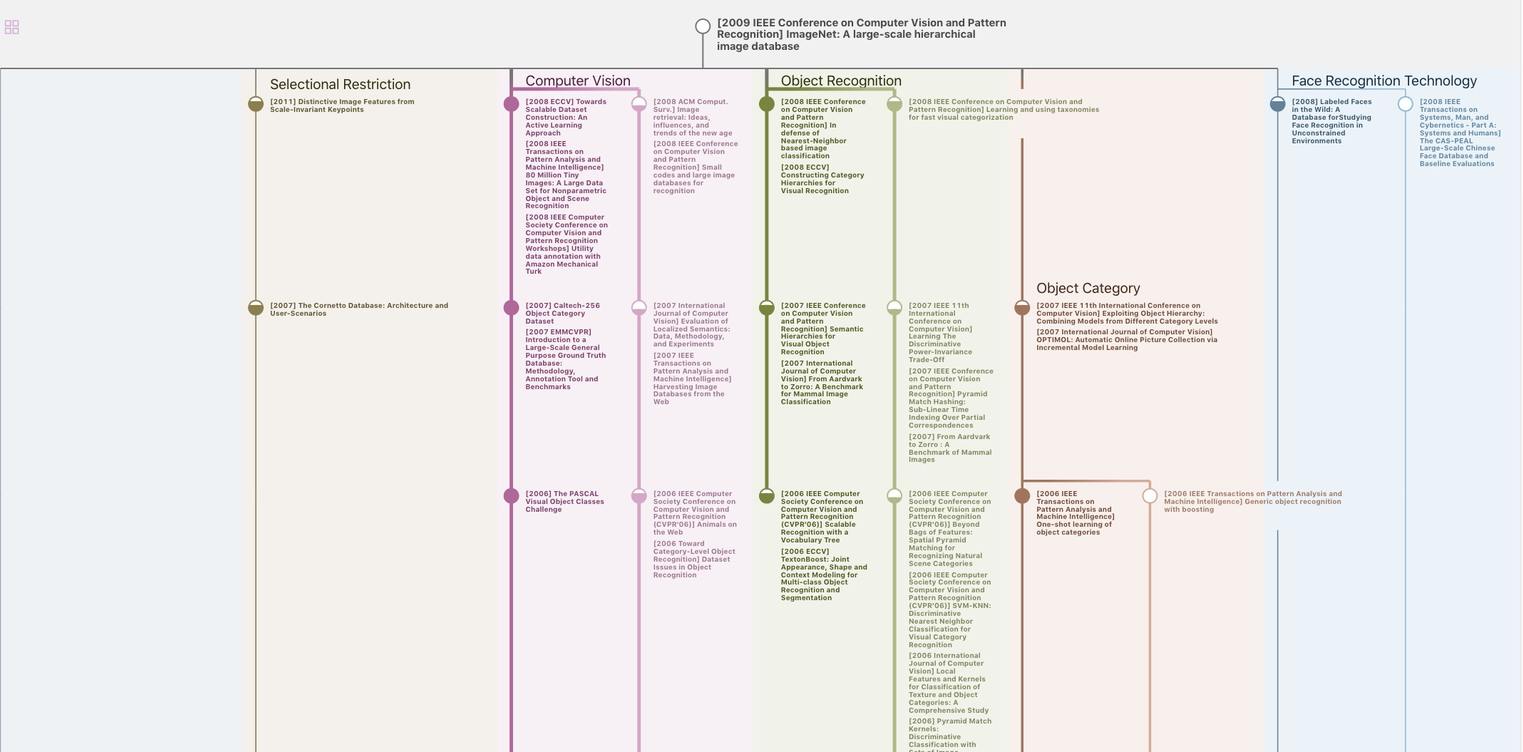
生成溯源树,研究论文发展脉络
Chat Paper
正在生成论文摘要