Closed-loop Data-Enabled Predictive Control and its equivalence with Closed-loop Subspace Predictive Control
CoRR(2024)
摘要
Factors like improved data availability and increasing system complexity have
sparked interest in data-driven predictive control (DDPC) methods like
Data-enabled Predictive Control (DeePC). However, closed-loop identification
bias arises in the presence of noise, which reduces the effectiveness of
obtained control policies. In this paper we propose Closed-loop Data-enabled
Predictive Control (CL-DeePC), a framework that unifies different approaches to
address this challenge. To this end, CL-DeePC incorporates instrumental
variables (IVs) to synthesize and sequentially apply consistent single or
multi-step-ahead predictors. Furthermore, a computationally efficient CL-DeePC
implementation is developed that reveals an equivalence with Closed-loop
Subspace Predictive Control (CL-SPC). Compared to DeePC, CL-DeePC simulations
demonstrate superior reference tracking, with a sensitivity study finding a 48
lower susceptibility to noise-induced reference tracking performance
degradation.
更多查看译文
AI 理解论文
溯源树
样例
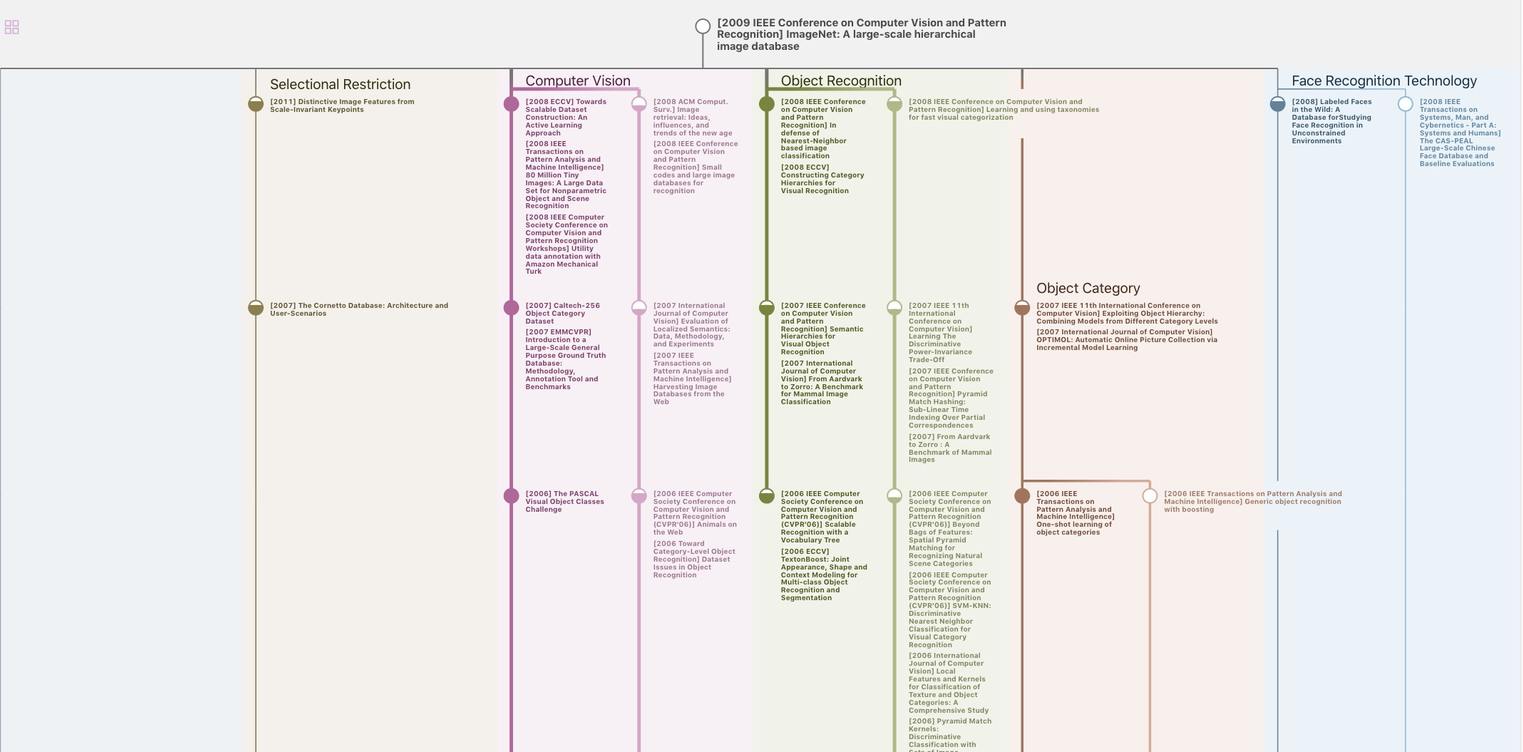
生成溯源树,研究论文发展脉络
Chat Paper
正在生成论文摘要