Composition design and optimization of Fe-C-Mn-Al steel based on machine learning
PHYSICAL CHEMISTRY CHEMICAL PHYSICS(2024)
摘要
The purpose of this study is to explore the composition space of Fe-C-Mn-Al steel using machine learning in order to identify materials with high-strength mechanical properties. A dataset of 580 steel samples was collected from the literature, each containing information on elemental composition, heat treatment processes, specimen dimensions, and mechanical properties (ultimate tensile strength and total elongation). Eight common machine learning models were constructed to predict the ultimate tensile strength (UTS) and total elongation (TE) of the steel. It was observed that the random forest regression (RFR) model, when trained, demonstrated superior overall performance in predicting UTS, with an average absolute error of approximately 90 MPa, and TE, with an average absolute error of about 7.9%. Validation of the model using eight sets of data that were not part of the dataset revealed that the predictions were in close agreement with experimental results, indicating the strong predictive capability of the RFR model. Subsequently, the trained RFR model was used to explore the composition space of Fe-C-Mn-Al steel, identifying the top fifty combinations of elemental compositions and heat treatment parameters, all of which manifest high ultimate tensile strength (UTS). This provides valuable research directions and methods to expedite the development of high-strength Fe-C-Mn-Al steel. The purpose of this study is to explore the composition space of Fe-C-Mn-Al steel using machine learning in order to identify materials with high-strength mechanical properties.
更多查看译文
AI 理解论文
溯源树
样例
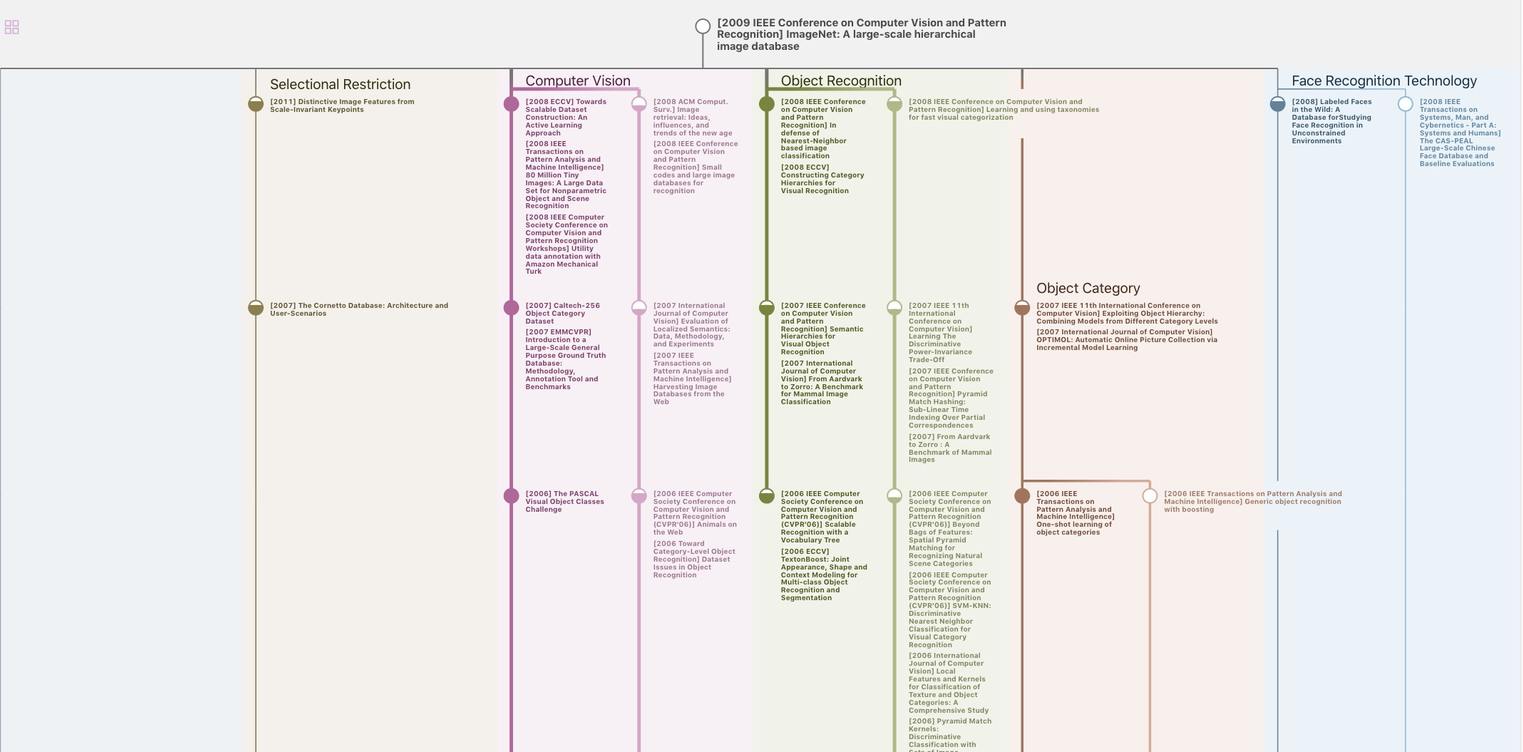
生成溯源树,研究论文发展脉络
Chat Paper
正在生成论文摘要