Machine-learning-driven discovery of metal-organic framework adsorbents for hexavalent chromium removal from aqueous environments
JOURNAL OF COLLOID AND INTERFACE SCIENCE(2024)
摘要
Hypothesis: Metal -organic frameworks (MOFs) have been widely studied for Cr(VI) adsorption in water. Theoretically, numerous MOFs can be synthesised by assembling diverse metals and ligands. However, the traditional manual experimentation for screening high-performance MOFs is resource -intensive and inefficient. Experiments: A screening strategy for MOFs based on machine learning was proposed for the adsorption and removal of Cr(VI) from water. By collecting the characteristics of MOFs and the experimental parameters of Cr (VI) adsorption from the literature, a dataset was constructed to predict the adsorption performance. Among the six regression models, the model trained by the extreme gradient boosted tree algorithm had the best performance and was used to simulate the adsorption and screen potential high-performance adsorbents. Findings: Structure -property analysis indicated that prepared MOF adsorbents with properties of 0.37 < largest cavity diameter < 0.71 nm, 0.18 < pore volume < 0.57 cm(3)/g, 412 < specific surface area < 1588 m(2)/g, 0.43 < void fraction < 0.62 will achieve enhanced adsorption of Cr(VI) in water. High-performance adsorbents were successfully screened using a combination of machine -learning prediction and analysis. Experiments were conducted to verify the exceptional adsorption capacity of UiO-66 and MOF-801. This method effectively identified adsorbents and accelerated the development of new MOF adsorbents for contaminant removal, providing a novel approach for the discovery of superior adsorbents.
更多查看译文
关键词
Cr(VI) removal from solution,Adsorption,Metal-organic frameworks,Machine learning,Material screening,Prediction model
AI 理解论文
溯源树
样例
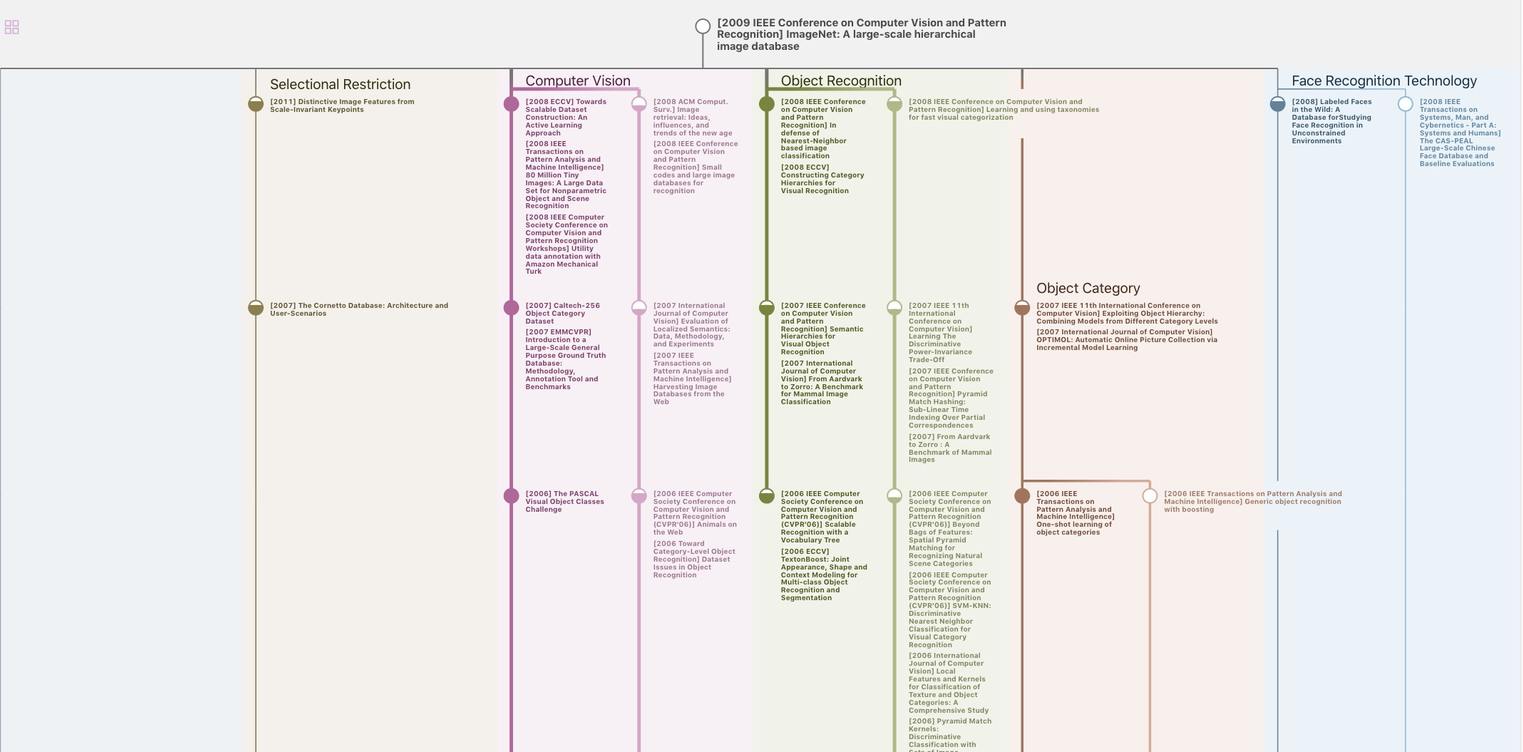
生成溯源树,研究论文发展脉络
Chat Paper
正在生成论文摘要