Laying the experimental foundation for corrosion inhibitor discovery through machine learning
npj Materials Degradation(2024)
摘要
Creating durable, eco-friendly coatings for long-term corrosion protection requires innovative strategies to streamline design and development processes, conserve resources, and decrease maintenance costs. In this pursuit, machine learning emerges as a promising catalyst, despite the challenges presented by the scarcity of high-quality datasets in the field of corrosion inhibition research. To address this obstacle, we have created an extensive electrochemical library of around 80 inhibitor candidates. The electrochemical behaviour of inhibitor-exposed AA2024-T3 substrates was captured using linear polarisation resistance, electrochemical impedance spectroscopy, and potentiodynamic polarisation techniques at different exposure times to obtain the most comprehensive electrochemical picture of the corrosion inhibition over a 24-h period. The experimental results yield target parameters and additional input features that can be combined with computational descriptors to develop quantitative structure–property relationship (QSPR) models augmented by mechanistic input features.
更多查看译文
AI 理解论文
溯源树
样例
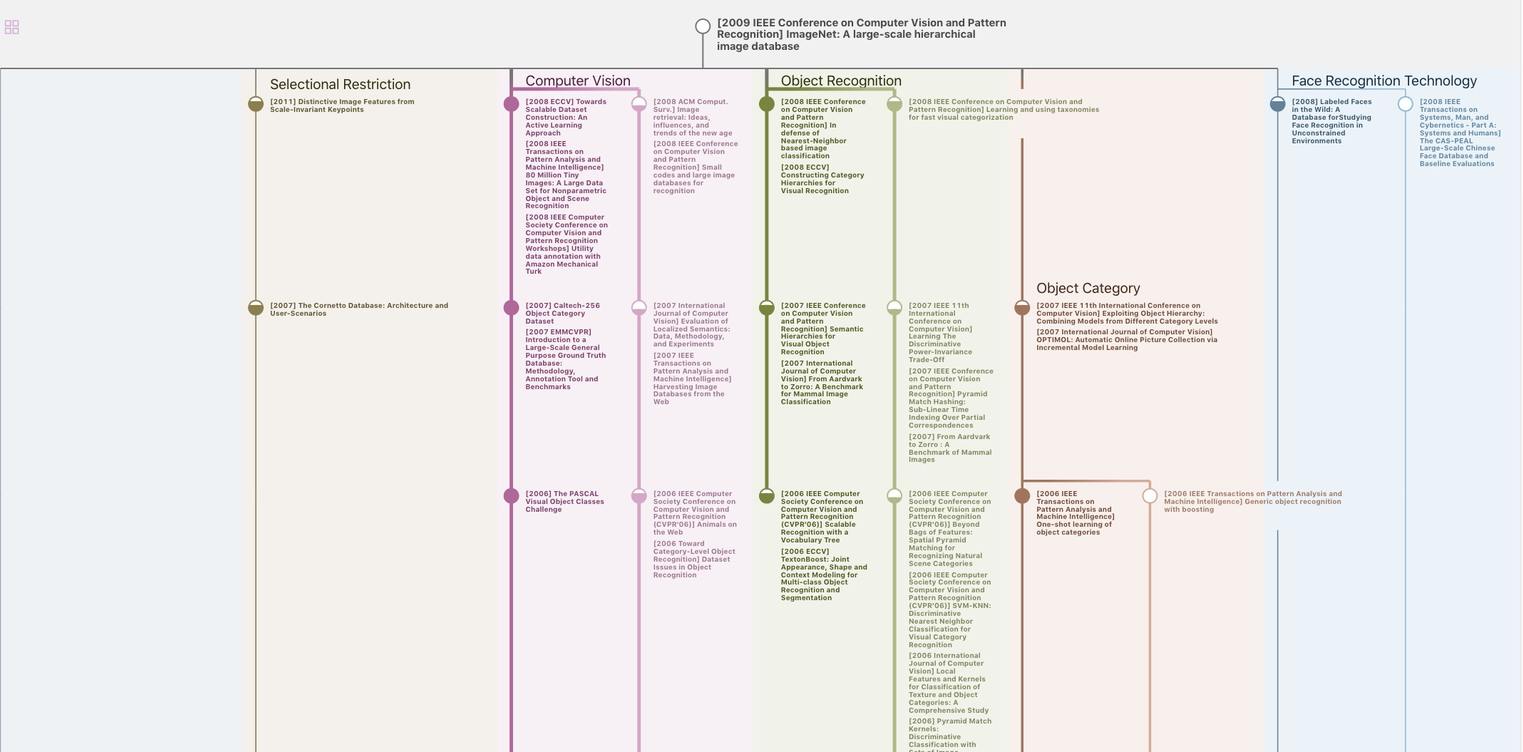
生成溯源树,研究论文发展脉络
Chat Paper
正在生成论文摘要