Large Language Model-Driven Urban Traffic Signal Control
2024 AUSTRALIAN & NEW ZEALAND CONTROL CONFERENCE, ANZCC(2024)
摘要
In recent years, large language models (LLM) have received a lot of attention for their ability to understand, generate and process natural language. By fine-tuning the models on specific domains or using prompts, LLMs can generate summaries tailored to different contexts and requirements. We hope to utilize these advantages of LLM in urban traffic signal control and provide a new paradigm for traffic control. In this paper, we propose a large language model-driven urban traffic control method, which is designed based on artificial systems, computational experiments, parallel execution (ACP) method. There are three traffic control modes, autonomous mode, feedback mode, and human takeover mode. In autonomous mode, we adopt recommendations based on inventory strategy, which can to some extent reduce the acquisition time of traffic control strategies. With the continuous construction and improvement of the strategic library, the efficiency of the system will be further improved. The feedback mode is mainly aimed at traffic scenarios that are not in the library, generating new control strategies and introducing human feedback. The human takeover model ensures that human engineers have direct control over the actual transportation system. LLM drives the interaction between human engineers and computers in the process of traffic control. Assist human engineers in achieving traffic control and improving work efficiency.
更多查看译文
关键词
Light Signal,Traffic Control,Traffic Signal Control,Urban Traffic Control,Control Strategy,Paradigm Shift,Efficient System,Library Construction,Natural Language,Direct Control,Artificial Systems,Language Model,Human Technology,Feedback Modalities,Traffic Scenarios,Parallel Execution,Autonomous Mode,Improve Work Efficiency,Operation Mode,Traffic Flow,Computer Language,Scenario Generation,Road Information
AI 理解论文
溯源树
样例
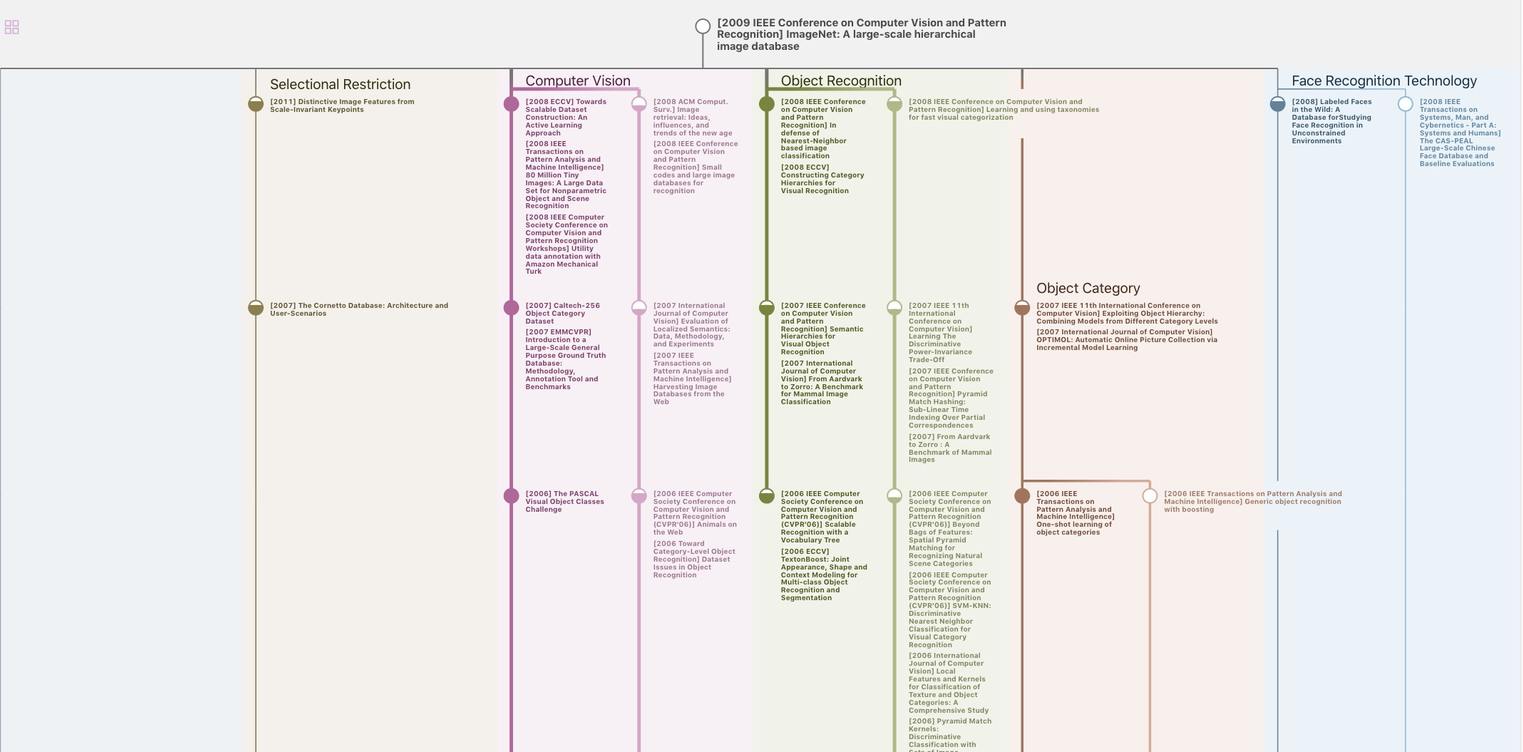
生成溯源树,研究论文发展脉络
Chat Paper
正在生成论文摘要