A multi-factor combination prediction model of carbon emissions based on improved CEEMDAN
Environmental Science and Pollution Research(2024)
摘要
As the global greenhouse effect intensifies, carbon emissions are gradually becoming a hot topic of discussion. Accurate carbon emissions prediction is an important foundation to realize carbon neutrality and peak carbon dioxide emissions. To accurately predict carbon emissions, a multi-factor combination prediction model based on improved complete ensemble empirical mode decomposition with adaptive noise (ICEEMDAN), bidirectional long short-term memory optimized by lemurs optimizer (LOBiLSTM) and least squares support vector machine optimized by lemurs optimizer (LOLSSVM), named ICEEMDAN-LOBiLSTM-LOLSSVM, is proposed. Firstly, the influencing factors of carbon emissions are selected by Spearman correlation coefficient, and carbon emissions are decomposed into intrinsic mode functions (IMFs) by ICEEMDAN. Secondly, the influencing factors and IMFs are input into LOBiLSTM and LOLSSVM respectively for prediction. Then, the point prediction results are obtained by weighting the prediction results of LOBiLSTM and LOLSSVM. Finally, probability density function of point prediction error is calculated by kernel density estimation, and the interval prediction results are calculated according to different confidence intervals. Carbon emissions of China and Germany are selected to verify the superiority of ICEEMDAN-LOBiLSTM-LOLSSVM. The experiment shows that RMSE, MAE, MAPE, and R 2 of the proposed model are 0.4468, 0.3612, 0.0120, and 0.9839 respectively for China, which is the best among the nine models, as well as for Germany.
更多查看译文
关键词
Carbon emission,Prediction,Decomposition,Multi-factor,Weighting
AI 理解论文
溯源树
样例
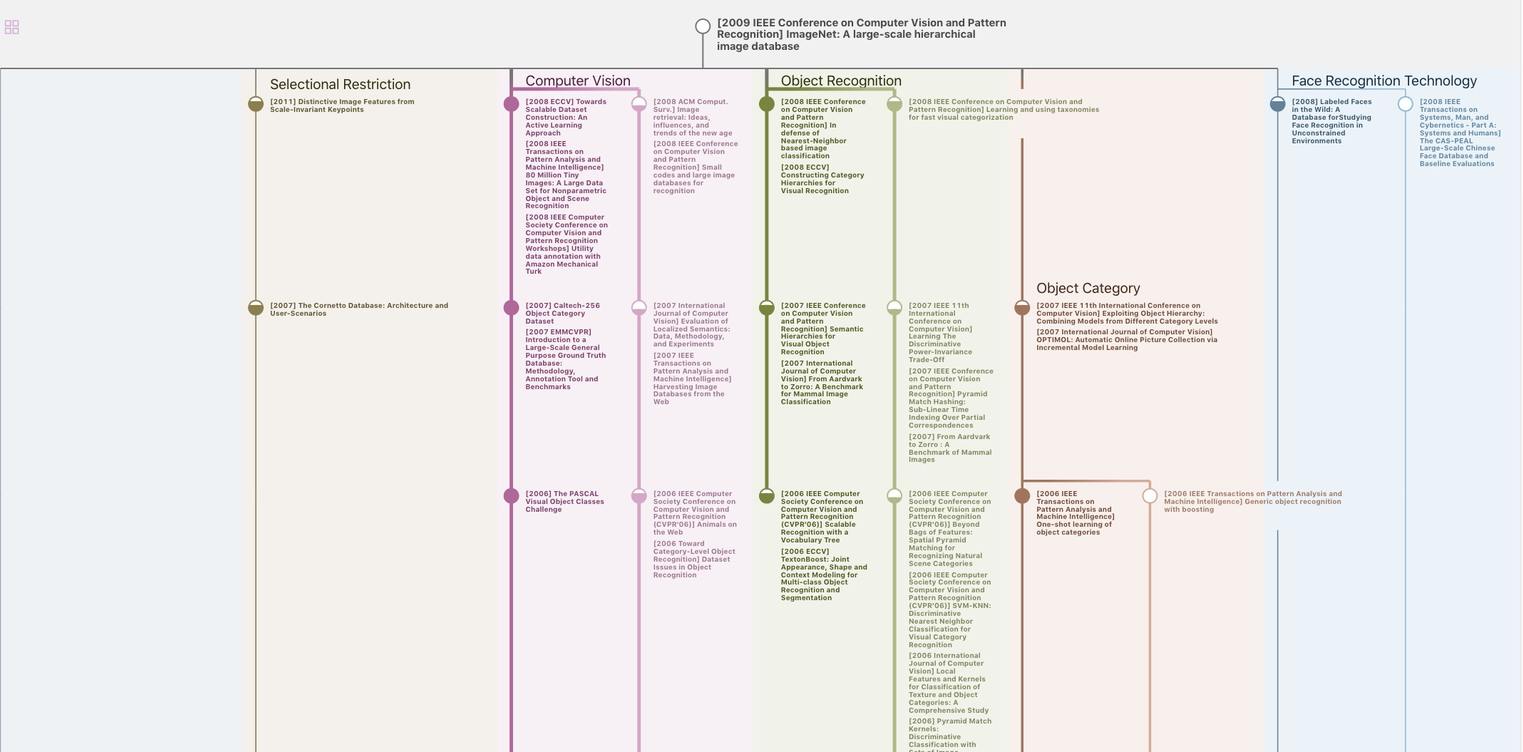
生成溯源树,研究论文发展脉络
Chat Paper
正在生成论文摘要