TofuEval: Evaluating Hallucinations of LLMs on Topic-Focused Dialogue Summarization
CoRR(2024)
摘要
Single document news summarization has seen substantial progress on
faithfulness in recent years, driven by research on the evaluation of factual
consistency, or hallucinations. We ask whether these advances carry over to
other text summarization domains. We propose a new evaluation benchmark on
topic-focused dialogue summarization, generated by LLMs of varying sizes. We
provide binary sentence-level human annotations of the factual consistency of
these summaries along with detailed explanations of factually inconsistent
sentences. Our analysis shows that existing LLMs hallucinate significant
amounts of factual errors in the dialogue domain, regardless of the model's
size. On the other hand, when LLMs, including GPT-4, serve as binary factual
evaluators, they perform poorly and can be outperformed by prevailing
state-of-the-art specialized factuality evaluation metrics. Finally, we
conducted an analysis of hallucination types with a curated error taxonomy. We
find that there are diverse errors and error distributions in model-generated
summaries and that non-LLM based metrics can capture all error types better
than LLM-based evaluators.
更多查看译文
AI 理解论文
溯源树
样例
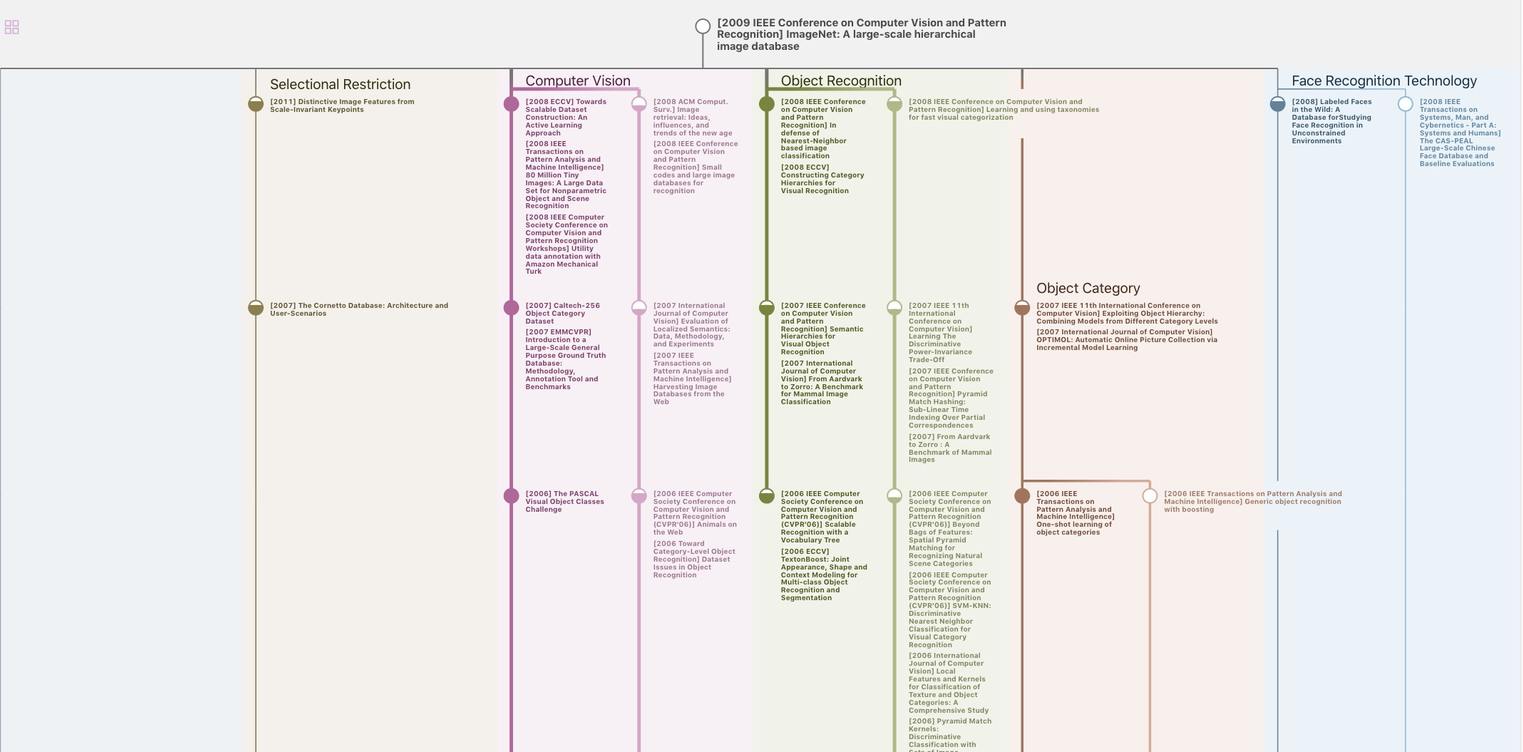
生成溯源树,研究论文发展脉络
Chat Paper
正在生成论文摘要