Order-Optimal Regret in Distributed Kernel Bandits using Uniform Sampling with Shared Randomness
CoRR(2024)
摘要
We consider distributed kernel bandits where N agents aim to
collaboratively maximize an unknown reward function that lies in a reproducing
kernel Hilbert space. Each agent sequentially queries the function to obtain
noisy observations at the query points. Agents can share information through a
central server, with the objective of minimizing regret that is accumulating
over time T and aggregating over agents. We develop the first algorithm that
achieves the optimal regret order (as defined by centralized learning) with a
communication cost that is sublinear in both N and T. The key features of
the proposed algorithm are the uniform exploration at the local agents and
shared randomness with the central server. Working together with the sparse
approximation of the GP model, these two key components make it possible to
preserve the learning rate of the centralized setting at a diminishing rate of
communication.
更多查看译文
AI 理解论文
溯源树
样例
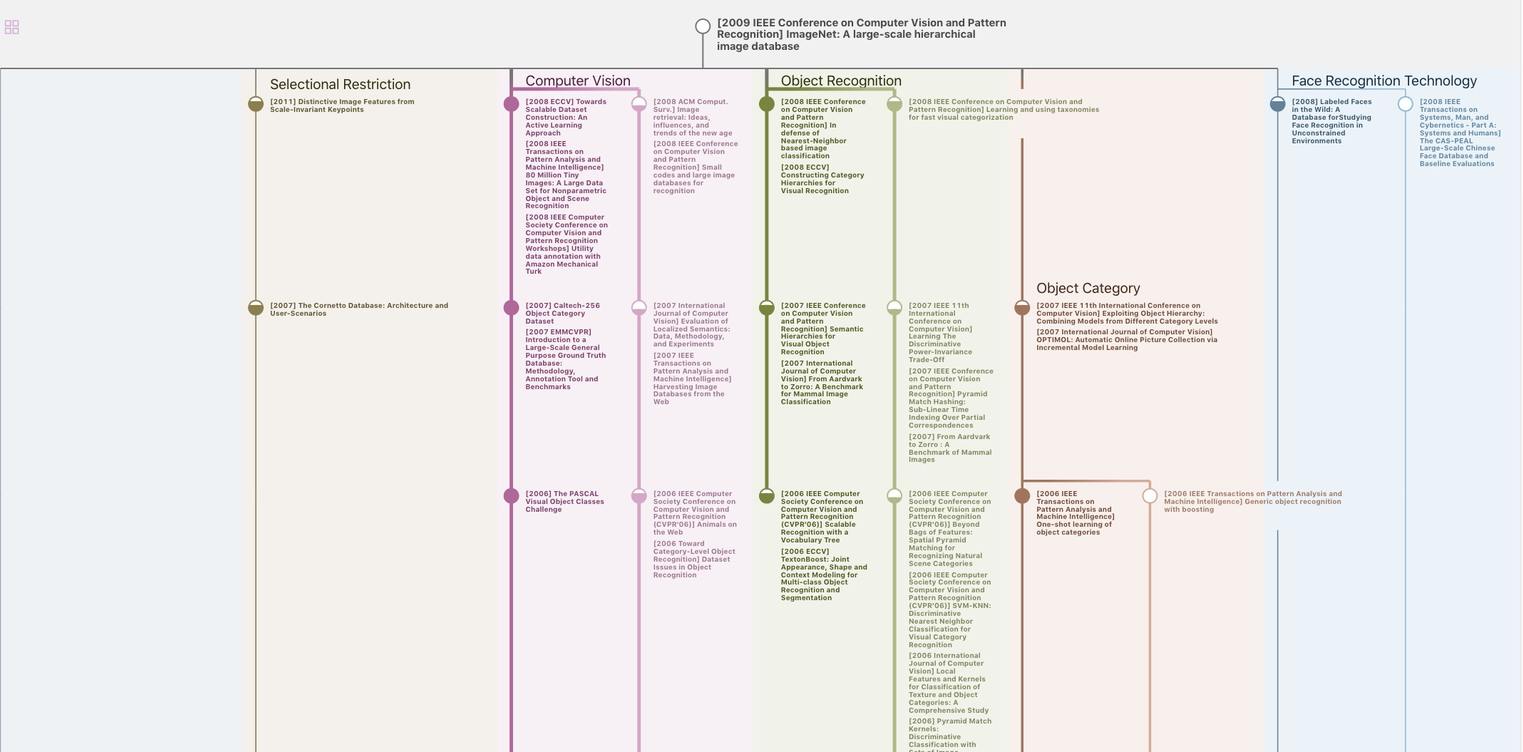
生成溯源树,研究论文发展脉络
Chat Paper
正在生成论文摘要