Randomization Can Reduce Both Bias and Variance: A Case Study in Random Forests
CoRR(2024)
摘要
We study the often overlooked phenomenon, first noted in
, that random forests appear to reduce bias compared to
bagging. Motivated by an interesting paper by ,
where the authors argue that random forests reduce effective degrees of freedom
and only outperform bagging ensembles in low signal-to-noise ratio (SNR)
settings, we explore how random forests can uncover patterns in the data missed
by bagging. We empirically demonstrate that in the presence of such patterns,
random forests reduce bias along with variance and increasingly outperform
bagging ensembles when SNR is high. Our observations offer insights into the
real-world success of random forests across a range of SNRs and enhance our
understanding of the difference between random forests and bagging ensembles
with respect to the randomization injected into each split. Our investigations
also yield practical insights into the importance of tuning mtry in random
forests.
更多查看译文
AI 理解论文
溯源树
样例
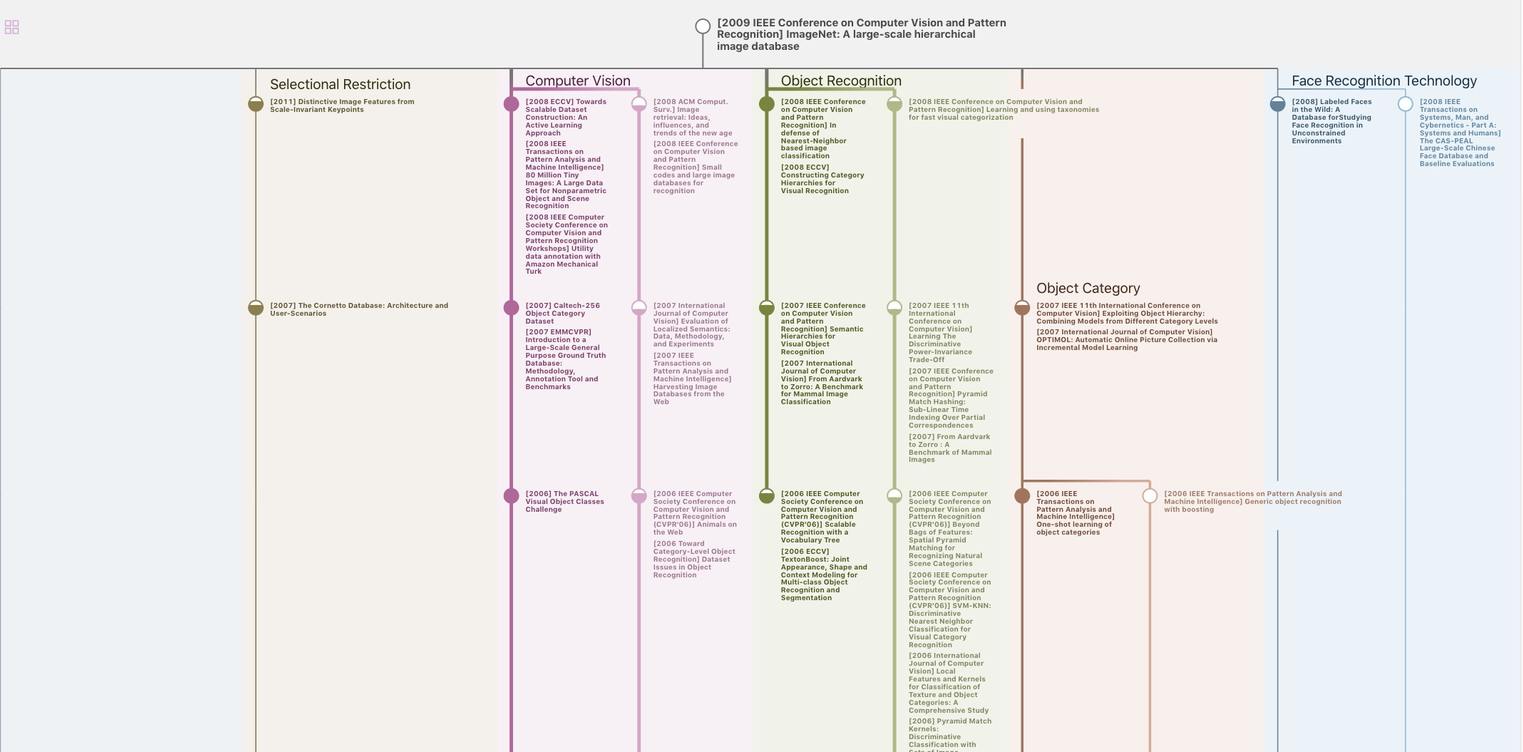
生成溯源树,研究论文发展脉络
Chat Paper
正在生成论文摘要