MOINER: A Novel Multiomics Early Integration Framework for Biomedical Classification and Biomarker Discovery
JOURNAL OF CHEMICAL INFORMATION AND MODELING(2024)
摘要
In the context of precision medicine, multiomics data integration provides a comprehensive understanding of underlying biological processes and is critical for disease diagnosis and biomarker discovery. One commonly used integration method is early integration through concatenation of multiple dimensionally reduced omics matrices due to its simplicity and ease of implementation. However, this approach is seriously limited by information loss and lack of latent feature interaction. Herein, a novel multiomics early integration framework (MOINER) based on information enhancement and image representation learning is thus presented to address the challenges. MOINER employs the self-attention mechanism to capture the intrinsic correlations of omics-features, which make it significantly outperform the existing state-of-the-art methods for multiomics data integration. Moreover, visualizing the attention embedding and identifying potential biomarkers offer interpretable insights into the prediction results.
更多查看译文
AI 理解论文
溯源树
样例
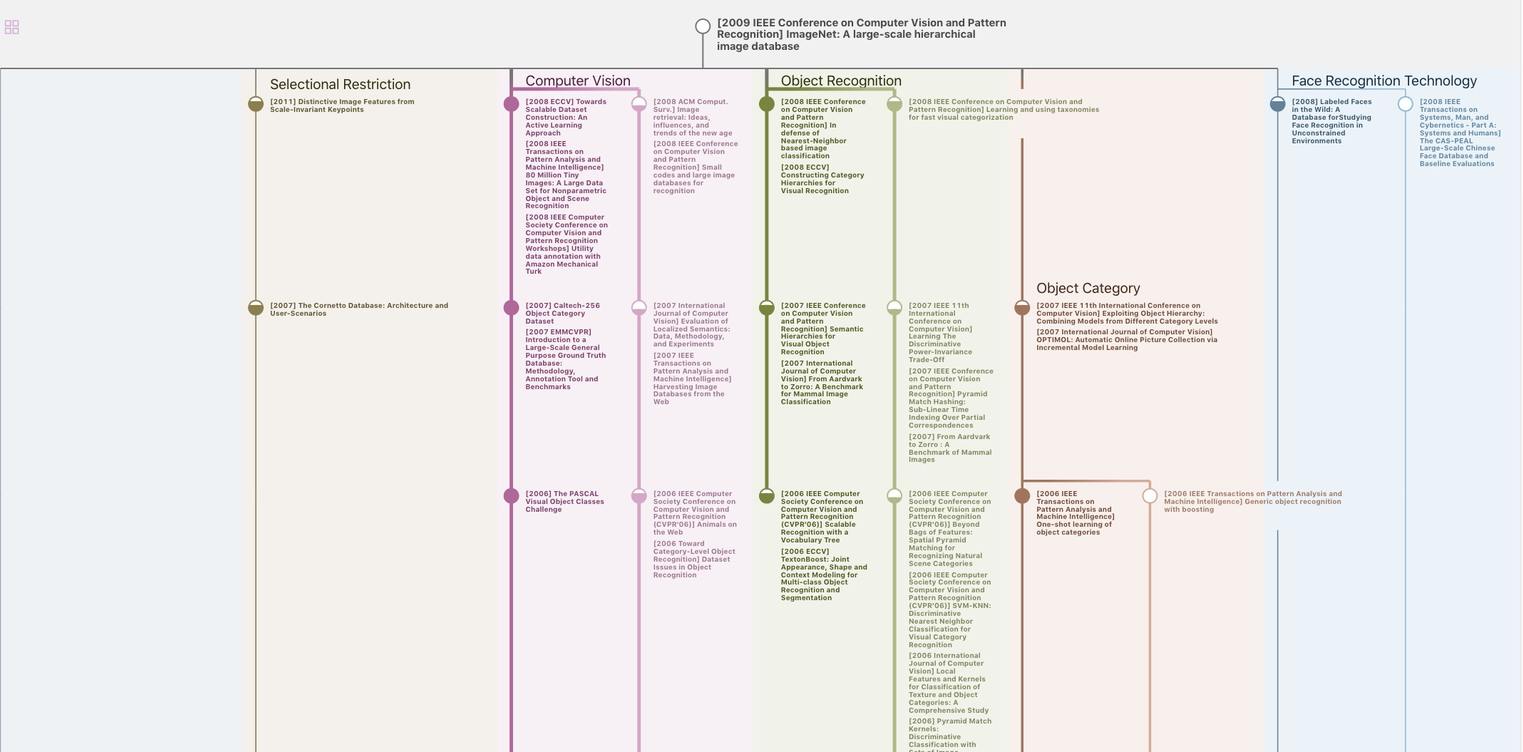
生成溯源树,研究论文发展脉络
Chat Paper
正在生成论文摘要