Spectrum learning for super-resolution tomographic reconstruction
PHYSICS IN MEDICINE AND BIOLOGY(2024)
摘要
Objective. Computed Tomography (CT) has been widely used in industrial high-resolution non-destructive testing. However, it is difficult to obtain high-resolution images for large-scale objects due to their physical limitations. The objective is to develop an improved super-resolution technique that preserves small structures and details while efficiently capturing high-frequency information. Approach. The study proposes a new deep learning based method called spectrum learning (SPEAR) network for CT images super-resolution. This approach leverages both global information in the image domain and high-frequency information in the frequency domain. The SPEAR network is designed to reconstruct high-resolution images from low-resolution inputs by considering not only the main body of the images but also the small structures and other details. The symmetric property of the spectrum is exploited to reduce weight parameters in the frequency domain. Additionally, a spectrum loss is introduced to enforce the preservation of both high-frequency components and global information. Main results. The network is trained using pairs of low-resolution and high-resolution CT images, and it is fine-tuned using additional low-dose and normal-dose CT image pairs. The experimental results demonstrate that the proposed SPEAR network outperforms state-of-the-art networks in terms of image reconstruction quality. The approach successfully preserves high-frequency information and small structures, leading to better results compared to existing methods. The network's ability to generate high-resolution images from low-resolution inputs, even in cases of low-dose CT images, showcases its effectiveness in maintaining image quality. Significance. The proposed SPEAR network's ability to simultaneously capture global information and high-frequency details addresses the limitations of existing methods, resulting in more accurate and informative image reconstructions. This advancement can have substantial implications for various industries and medical diagnoses relying on accurate imaging.
更多查看译文
关键词
computed tomography (CT),image reconstruction,super-resolution,spectrum learning,low-dose reconstruction
AI 理解论文
溯源树
样例
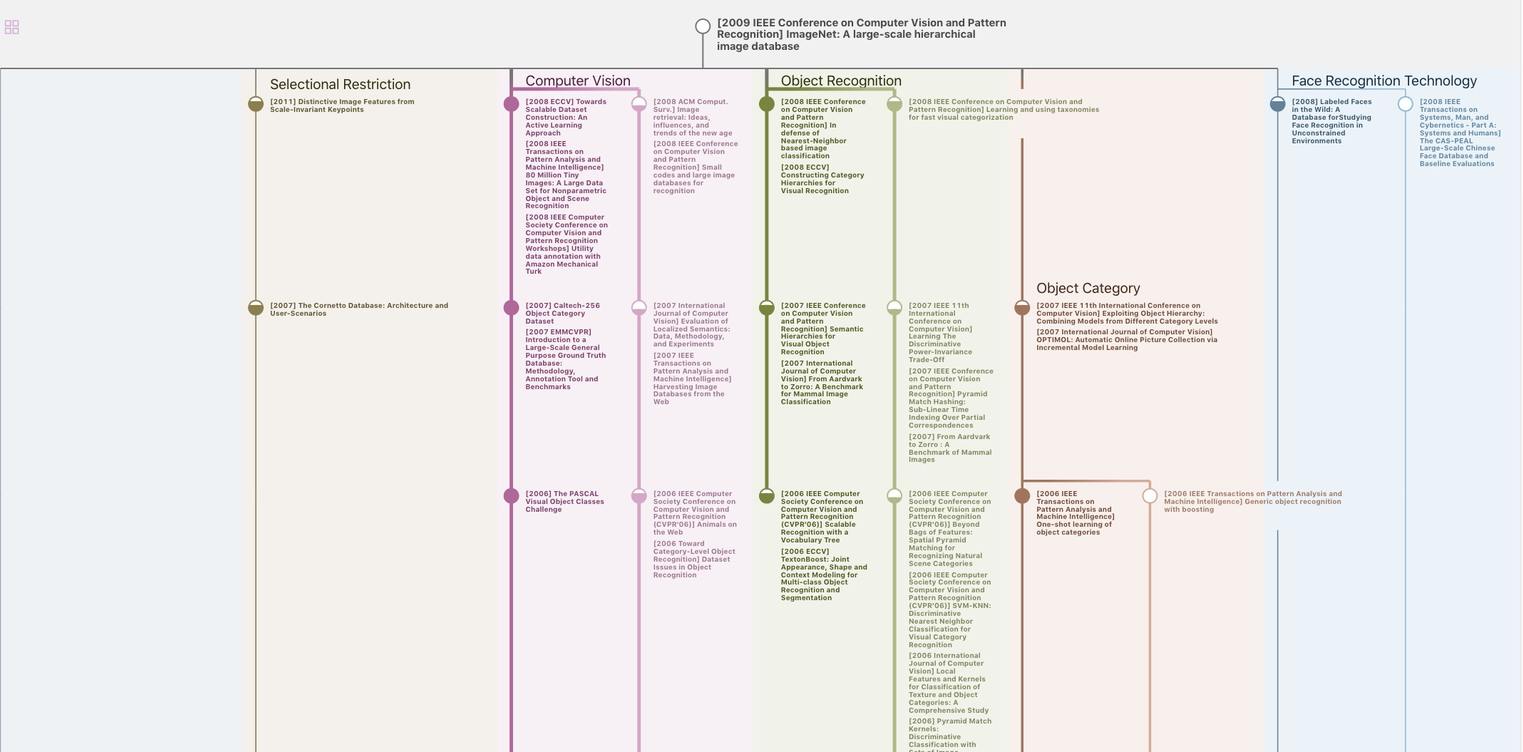
生成溯源树,研究论文发展脉络
Chat Paper
正在生成论文摘要