Enhancing lung cancer detection through hybrid features and machine learning hyperparameters optimization techniques
HELIYON(2024)
摘要
Machine learning offers significant potential for lung cancer detection, enabling early diagnosis and potentially improving patient outcomes. Feature extraction remains a crucial challenge in this domain. Combining the most relevant features can further enhance detection accuracy. This study employed a hybrid feature extraction approach, which integrates both Gray-level cooccurrence matrix (GLCM) with Haralick and autoencoder features with an autoencoder. These features were subsequently fed into supervised machine learning methods. Support Vector Machine (SVM) Radial Base Function (RBF) and SVM Gaussian achieved perfect performance measures, while SVM polynomial produced an accuracy of 99.89% when utilizing GLCM with an autoencoder, Haralick, and autoencoder features. SVM Gaussian achieved an accuracy of 99.56%, while SVM RBF achieved an accuracy of 99.35% when utilizing GLCM with Haralick features. These results demonstrate the potential of the proposed approach for developing improved diagnostic and prognostic lung cancer treatment planning and decision-making systems.
更多查看译文
关键词
Classification,Haralick texture features,Lung cancer types,Autoencoder and gray-level co-occurrence,(GLCM)
AI 理解论文
溯源树
样例
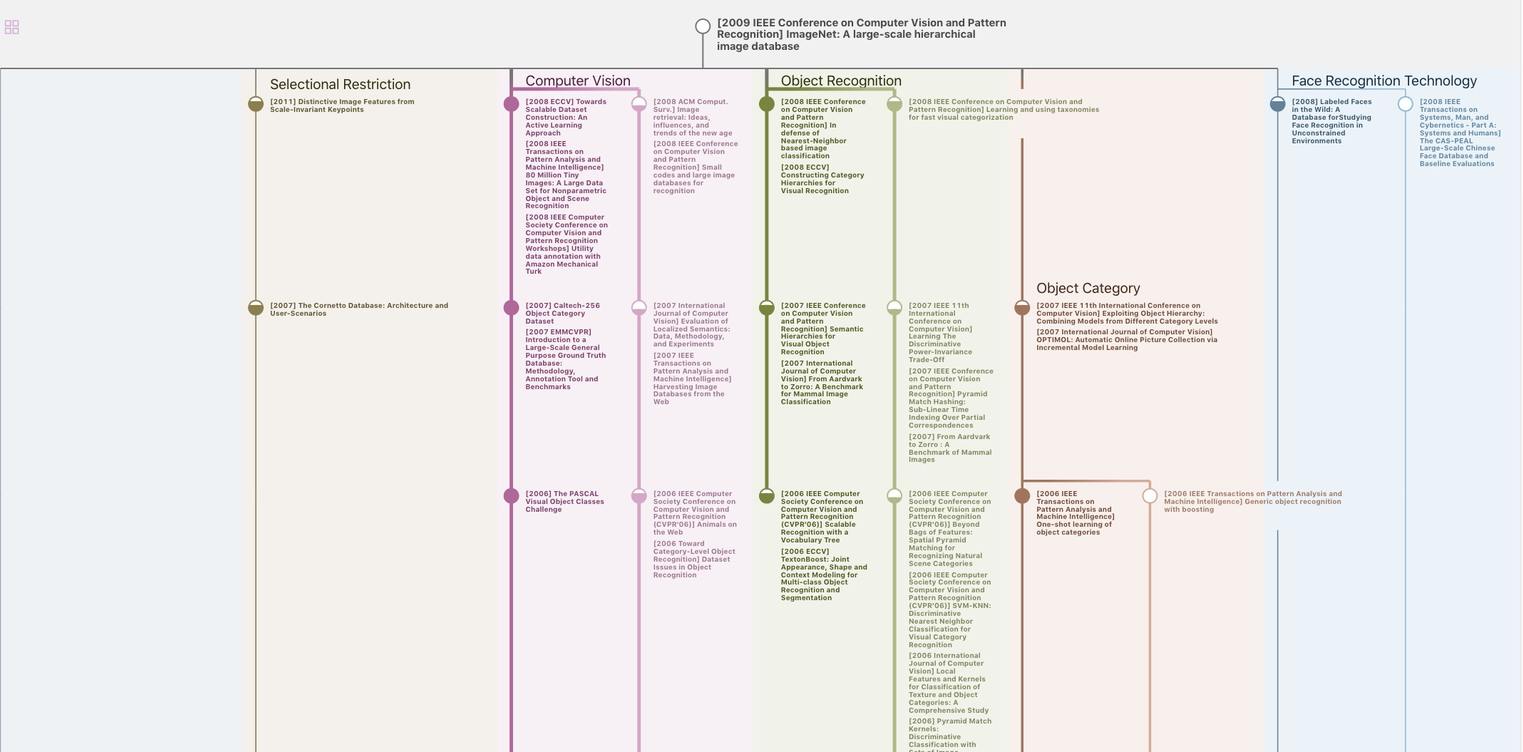
生成溯源树,研究论文发展脉络
Chat Paper
正在生成论文摘要