A Novel Training Approach in Deep Spiking Neural Network Based on Fuzzy Weighting and Meta-heuristic Algorithm
International Journal of Computational Intelligence Systems(2024)
摘要
The challenge of supervised learning in spiking neural networks (SNNs) for digit classification from speech signals is examined in this study. Meta-heuristic algorithms and a fuzzy logic framework are used to train SNNs. Using gray wolf optimization (GWO), the features obtained from audio signals are reduced depending on the dispersion of each feature. Then, it combines fuzzy weighting system (FWS) and spike time-dependent flexibility (STDP) approach to implement the learning rule in SNN. The FWS rule produces a uniformly distributed random weight in the STDP flexibility window, so that the system requires fewer training parameters. Finally, these neurons are fed data to estimate the training weights and threshold values of the neurons using wild horse algorithm (WHO). With the parameters given, these rule weights are applied to appropriately display the class's share in extracting the relevant feature. The suggested network can classify speech signals into categories with 97.17% accuracy. The dataset was obtained using neurons operating at sparse biological rates below 600 Hz in the TIDIGITS test database. The suggested method has been evaluated on the IRIS and Trip Data datasets, where the classification results showed a 98.93% and 97.36% efficiency, respectively. Compared to earlier efforts, this study's results demonstrate that the strategy is both computationally simpler and more accurate. The accuracy of classification of digits, IRIS and Trip Data has increased by 4.9, 3.46 and 1.24%, respectively. The principal goal of this research is to improve the accuracy of SNN by developing a new high-precision training method.
更多查看译文
关键词
Digit recognition system,Spiking neural network (SNN),Fuzzy weighting system (FWS),Gray wolf optimization (GWO),Wild horse algorithm (WHO)
AI 理解论文
溯源树
样例
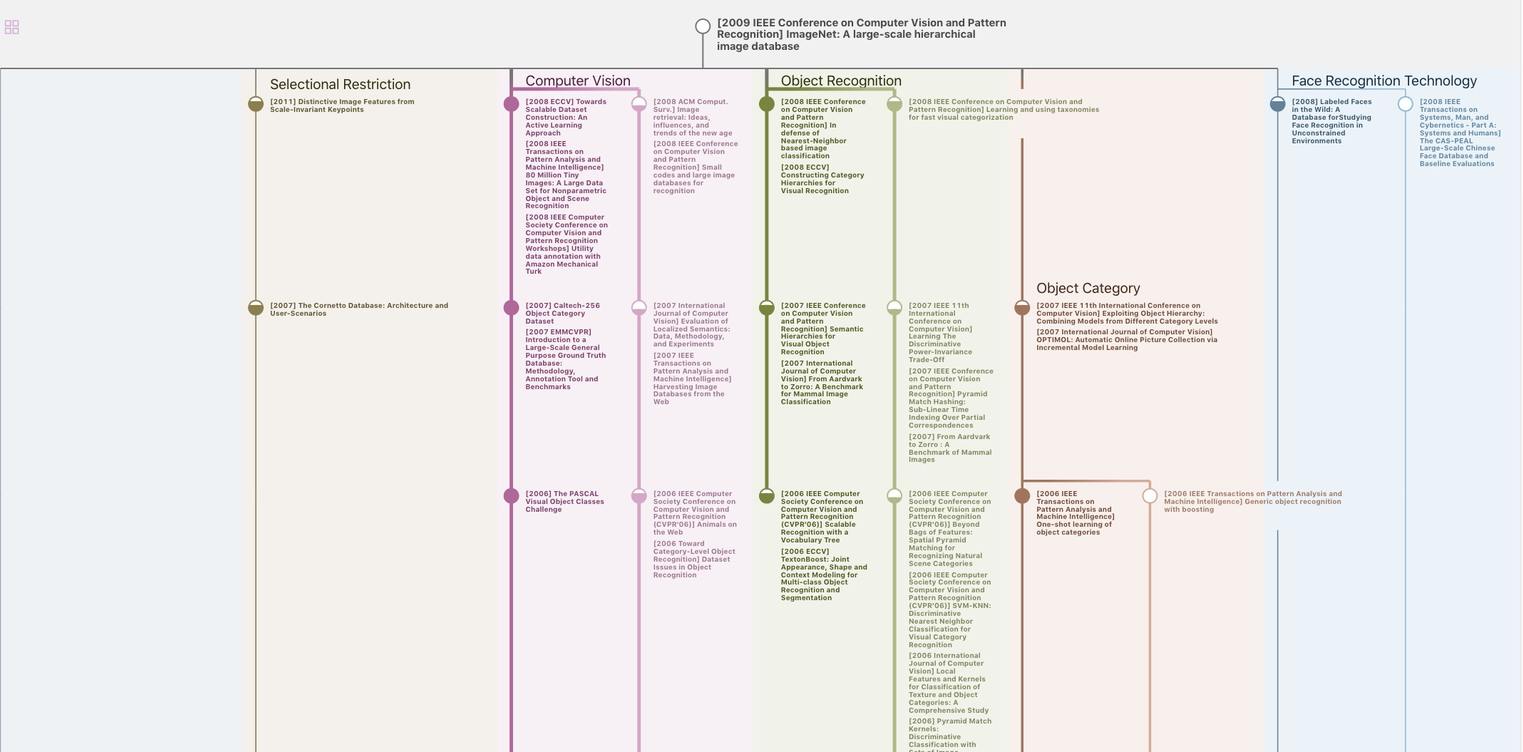
生成溯源树,研究论文发展脉络
Chat Paper
正在生成论文摘要