DeepRisk network: an AI-based tool for digital pathology signature and treatment responsiveness of gastric cancer using whole-slide images
Journal of Translational Medicine(2024)
摘要
Background Digital histopathology provides valuable information for clinical decision-making. We hypothesized that a deep risk network (DeepRisk) based on digital pathology signature (DPS) derived from whole-slide images could improve the prognostic value of the tumor, node, and metastasis (TNM) staging system and offer chemotherapeutic benefits for gastric cancer (GC). Methods DeepRisk is a multi-scale, attention-based learning model developed on 1120 GCs in the Zhongshan dataset and validated with two external datasets. Then, we assessed its association with prognosis and treatment response. The multi-omics analysis and multiplex Immunohistochemistry were conducted to evaluate the potential pathogenesis and spatial immune contexture underlying DPS. Results Multivariate analysis indicated that the DPS was an independent prognosticator with a better C-index (0.84 for overall survival and 0.71 for disease-free survival). Patients with low-DPS after neoadjuvant chemotherapy responded favorably to treatment. Spatial analysis indicated that exhausted immune clusters and increased infiltration of CD11b + CD11c + immune cells were present at the invasive margin of high-DPS group. Multi-omics data from the Cancer Genome Atlas-Stomach adenocarcinoma (TCGA-STAD) hint at the relevance of DPS to myeloid derived suppressor cells infiltration and immune suppression. Conclusion DeepRisk network is a reliable tool that enhances prognostic value of TNM staging and aid in precise treatment, providing insights into the underlying pathogenic mechanisms.
更多查看译文
关键词
Deep learning,Gastric cancer,Whole slide image,Artificial intelligence,Suppressive immune microenvironment
AI 理解论文
溯源树
样例
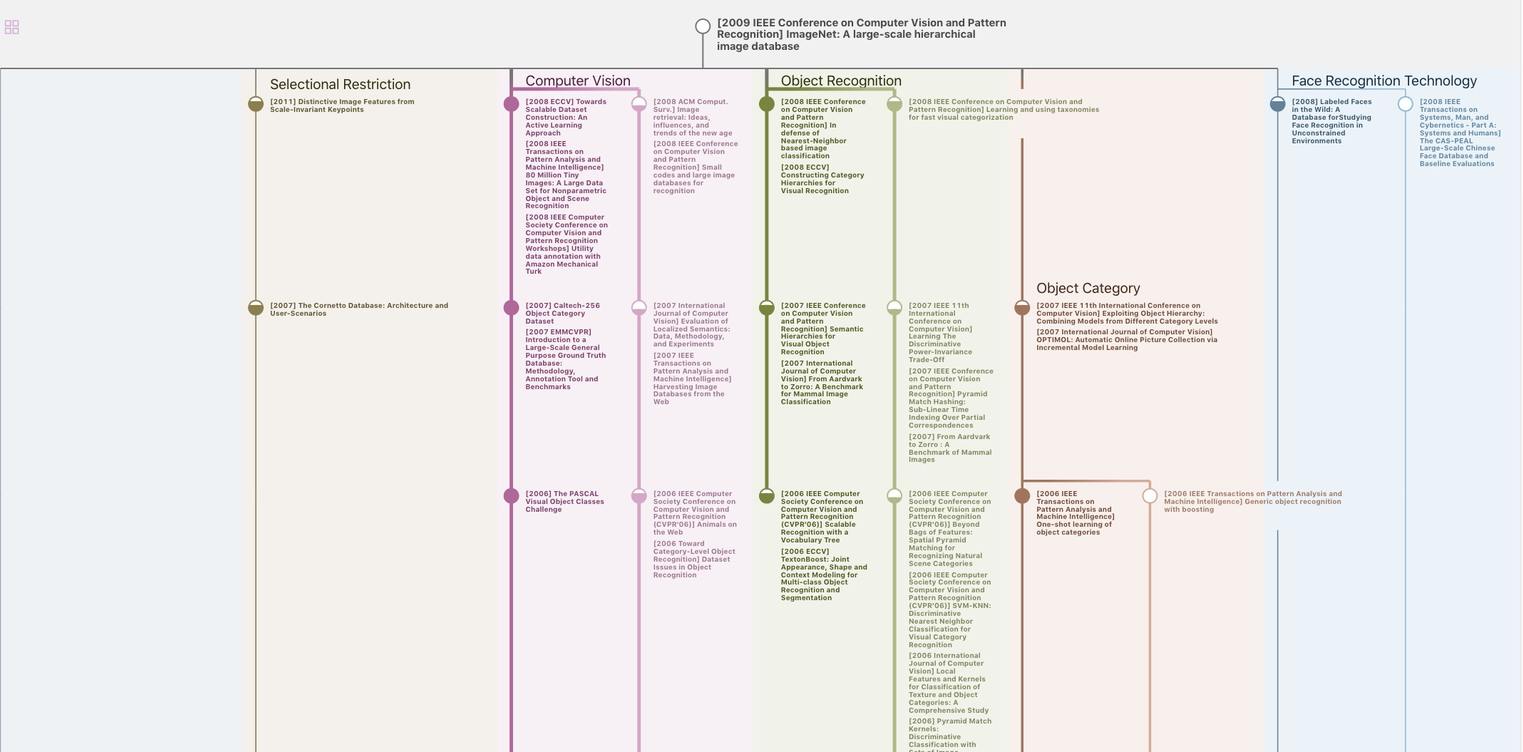
生成溯源树,研究论文发展脉络
Chat Paper
正在生成论文摘要