Gaussian Broadcast on Grids
CoRR(2024)
摘要
Motivated by the classical work on finite noisy automata (Gray 1982, Gács
2001, Gray 2001) and by the recent work on broadcasting on grids (Makur,
Mossel, and Polyanskiy 2022), we introduce Gaussian variants of these models.
These models are defined on graded posets. At time 0, all nodes begin with
X_0. At time k≥ 1, each node on layer k computes a combination of its
inputs at layer k-1 with independent Gaussian noise added. When is it
possible to recover X_0 with non-vanishing correlation? We consider different
notions of recovery including recovery from a single node, recovery from a
bounded window, and recovery from an unbounded window.
Our main interest is in two models defined on grids:
In the infinite model, layer k is the vertices of ℤ^d+1 whose
sum of entries is k and for a vertex v at layer k ≥ 1, X_v=α∑
(X_u + W_u,v), summed over all u on layer k-1 that differ from v
exactly in one coordinate, and W_u,v are i.i.d. 𝒩(0,1). We show
that when α<1/(d+1), the correlation between X_v and X_0 decays
exponentially, and when α>1/(d+1), the correlation is bounded away from
0. The critical case when α=1/(d+1) exhibits a phase transition in
dimension, where X_v has non-vanishing correlation with X_0 if and only if
d≥ 3. The same results hold for any bounded window.
In the finite model, layer k is the vertices of ℤ^d+1 with
nonnegative entries with sum k. We identify the sub-critical and the
super-critical regimes. In the sub-critical regime, the correlation decays to
0 for unbounded windows. In the super-critical regime, there exists for every
t a convex combination of X_u on layer t whose correlation is bounded
away from 0. We find that for the critical parameters, the correlation is
vanishing in all dimensions and for unbounded window sizes.
更多查看译文
AI 理解论文
溯源树
样例
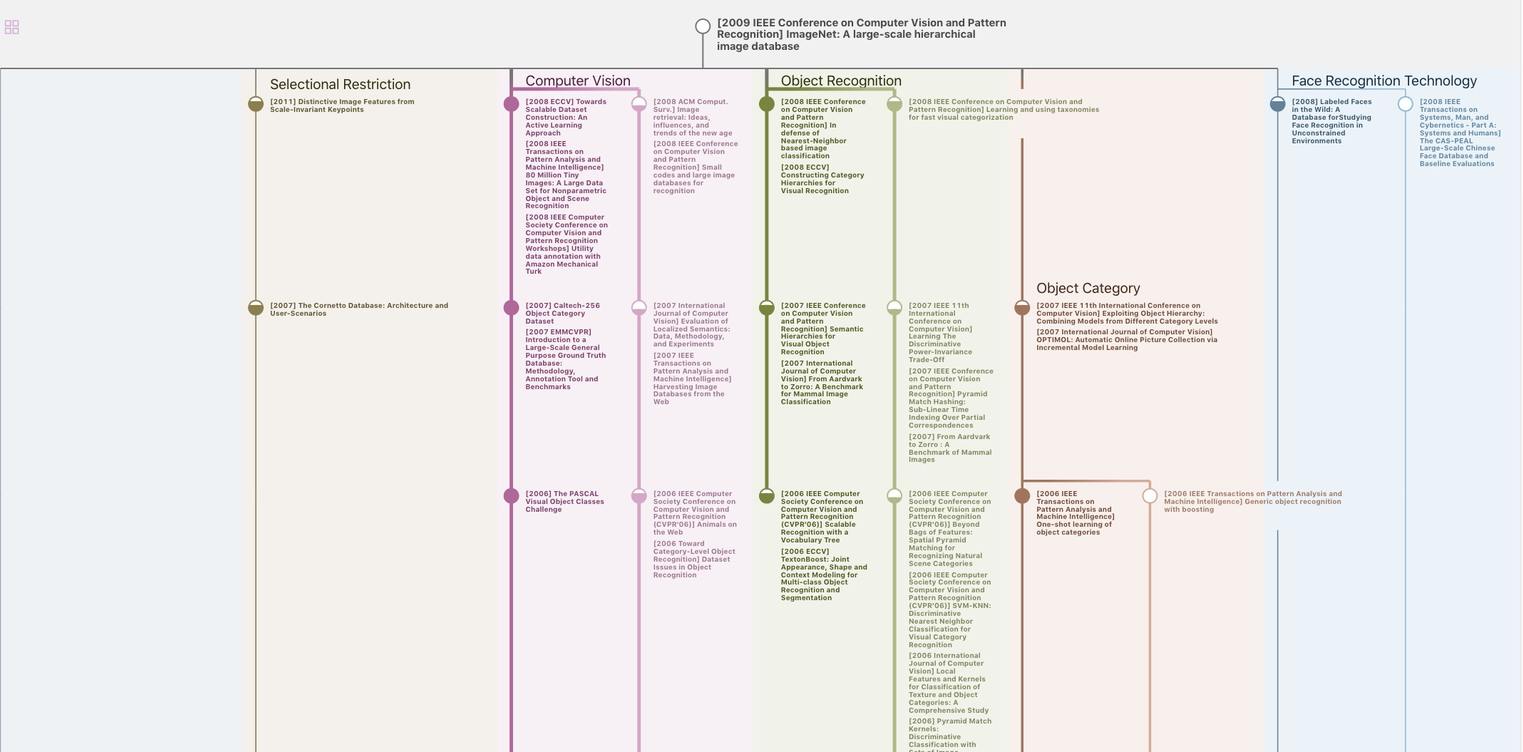
生成溯源树,研究论文发展脉络
Chat Paper
正在生成论文摘要