Bayesian Active Learning for Censored Regression
CoRR(2024)
摘要
Bayesian active learning is based on information theoretical approaches that
focus on maximising the information that new observations provide to the model
parameters. This is commonly done by maximising the Bayesian Active Learning by
Disagreement (BALD) acquisitions function. However, we highlight that it is
challenging to estimate BALD when the new data points are subject to
censorship, where only clipped values of the targets are observed. To address
this, we derive the entropy and the mutual information for censored
distributions and derive the BALD objective for active learning in censored
regression (𝒞-BALD). We propose a novel modelling approach to
estimate the 𝒞-BALD objective and use it for active learning in the
censored setting. Across a wide range of datasets and models, we demonstrate
that 𝒞-BALD outperforms other Bayesian active learning methods in
censored regression.
更多查看译文
AI 理解论文
溯源树
样例
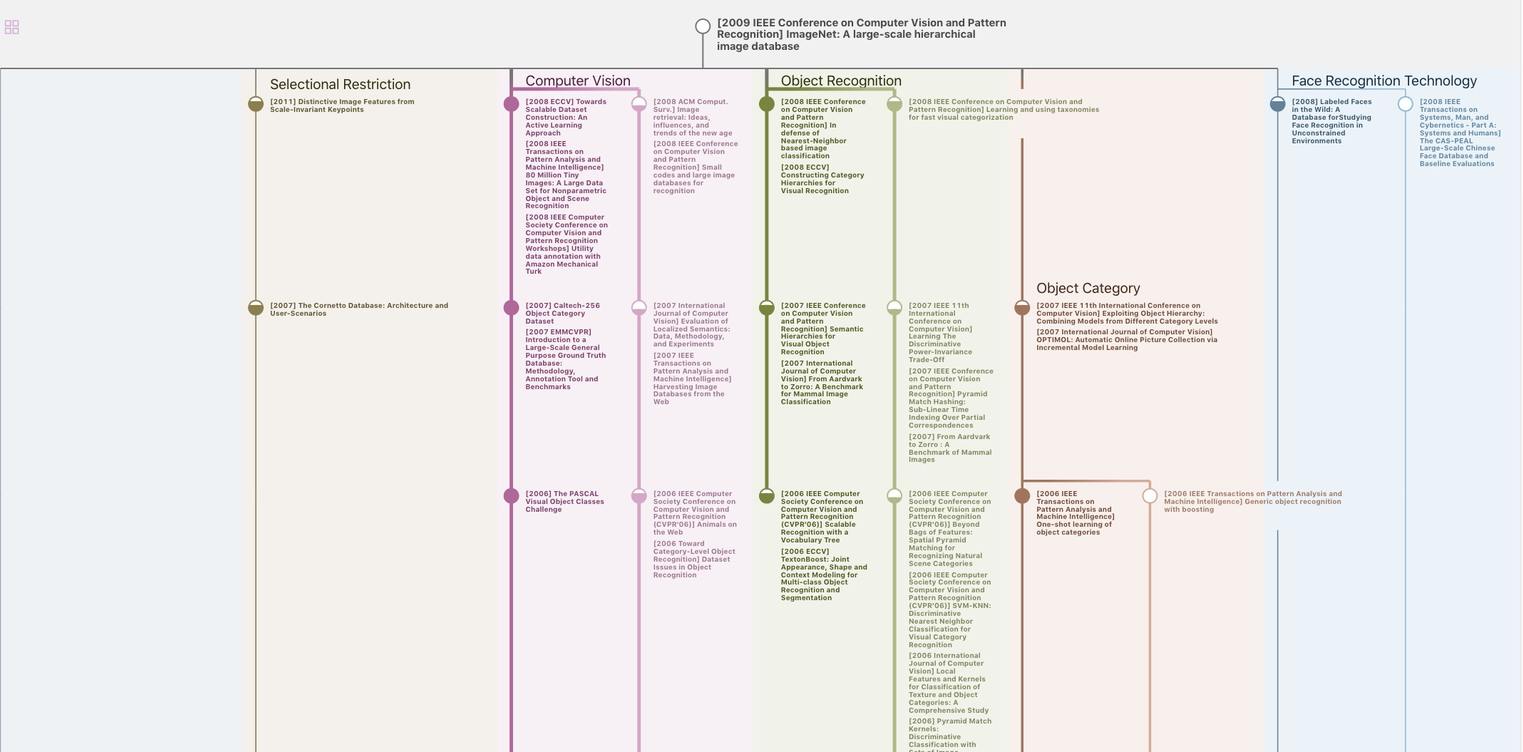
生成溯源树,研究论文发展脉络
Chat Paper
正在生成论文摘要