Analyzing the Impact of Design Factors on Solar Module Thermomechanical Durability Using Interpretable Machine Learning Techniques
arxiv(2024)
摘要
Solar modules in utility-scale PV systems are expected to maintain decades of
lifetime to effectively rival the cost of conventional energy sources. However,
the long-term performance of these modules is often degraded by cyclic
thermomechanical loading, emphasizing the need for a proper module design to
counteract the detrimental effects of thermal expansion mismatch between module
materials. Given the complex composition of solar modules, isolating the impact
of individual components on overall durability remains a challenging task. In
this work, we analyze a comprehensive data set capturing bill-of-materials and
post-thermal-cycling power loss from over 250 distinct module designs. Using
the data set, we develop a machine learning model to correlate the design
factors with the degradation and apply the Shapley additive explanation to
provide interpretative insights into the model's decision-making. Our analysis
reveals that the type of silicon solar cell, whether monocrystalline or
polycrystalline, predominantly influences the degradation, and monocrystalline
cells present better durability. This finding is further substantiated by
statistical testing on our raw data set. We also demonstrate that the thickness
of the encapsulant, particularly the front side one, remains another important
factor. While thicker encapsulants lead to reduced power loss, further
increasing their thickness does not yield additional benefits. The study
moreover provides a blueprint for utilizing explainable machine learning
techniques in a complex material system and can potentially steer future
research on optimizing solar module design.
更多查看译文
AI 理解论文
溯源树
样例
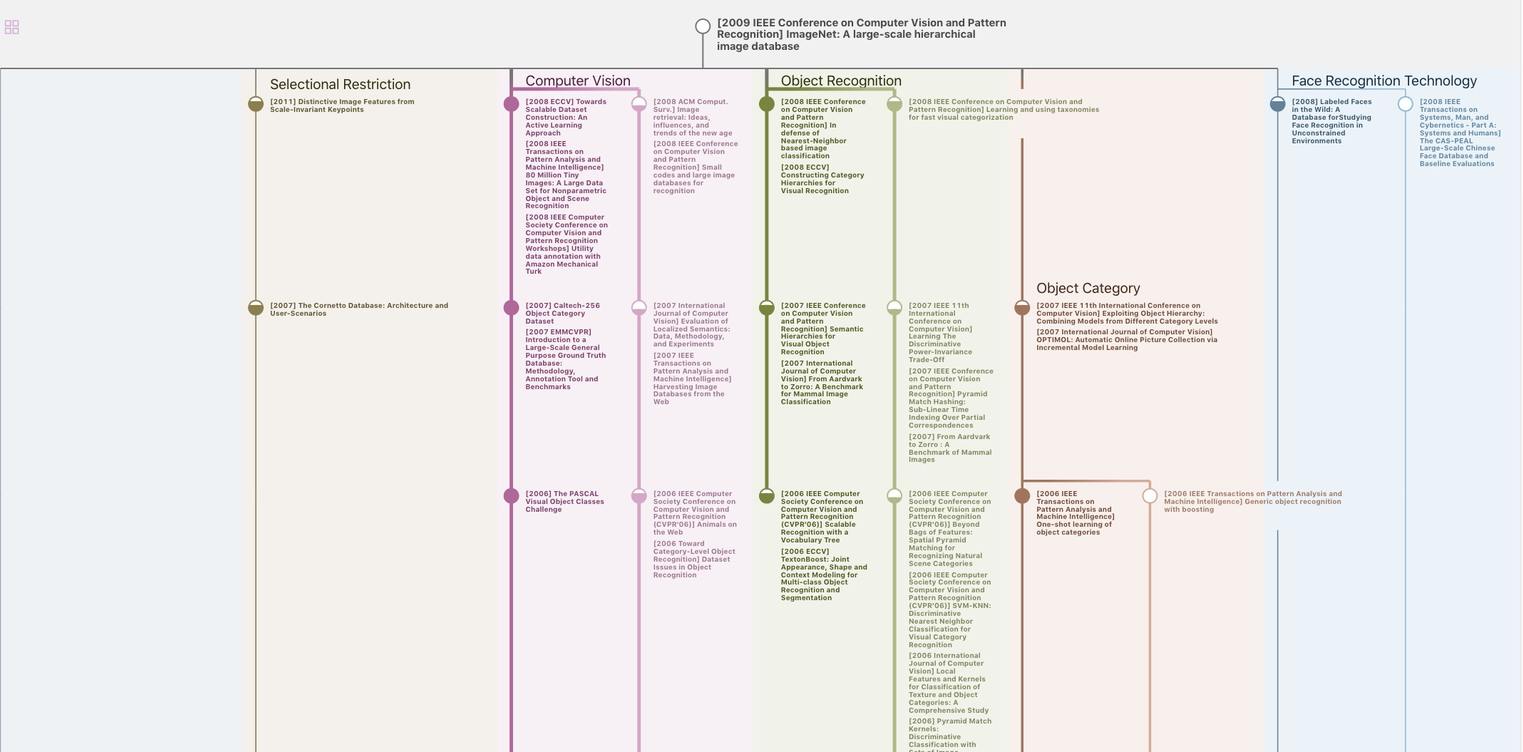
生成溯源树,研究论文发展脉络
Chat Paper
正在生成论文摘要