Evaluating Program Repair with Semantic-Preserving Transformations: A Naturalness Assessment
CoRR(2024)
摘要
In this paper, we investigate the naturalness of semantic-preserving
transformations and their impacts on the evaluation of NPR. To achieve this, we
conduct a two-stage human study, including (1) interviews with senior software
developers to establish the first concrete criteria for assessing the
naturalness of code transformations and (2) a survey involving 10 developers to
assess the naturalness of 1178 transformations, i.e., pairs of original and
transformed programs, applied to 225 real-world bugs. Our findings reveal that
nearly 60
unnatural with substantially high agreement among human annotators.
Furthermore, the unnatural code transformations introduce a 25.2
rate on robustness of five well-known NPR systems. Additionally, the
performance of the NPR systems drops notably when evaluated using natural
transformations, i.e., a drop of up to 22.9
of correct and plausible patches generated by these systems. These results
highlight the importance of robustness testing by considering naturalness of
code transformations, which unveils true effectiveness of NPR systems. Finally,
we conduct an exploration study on automating the assessment of naturalness of
code transformations by deriving a new naturalness metric based on
Cross-Entropy. Based on our naturalness metric, we can effectively assess
naturalness for code transformations automatically with an AUC of 0.7.
更多查看译文
AI 理解论文
溯源树
样例
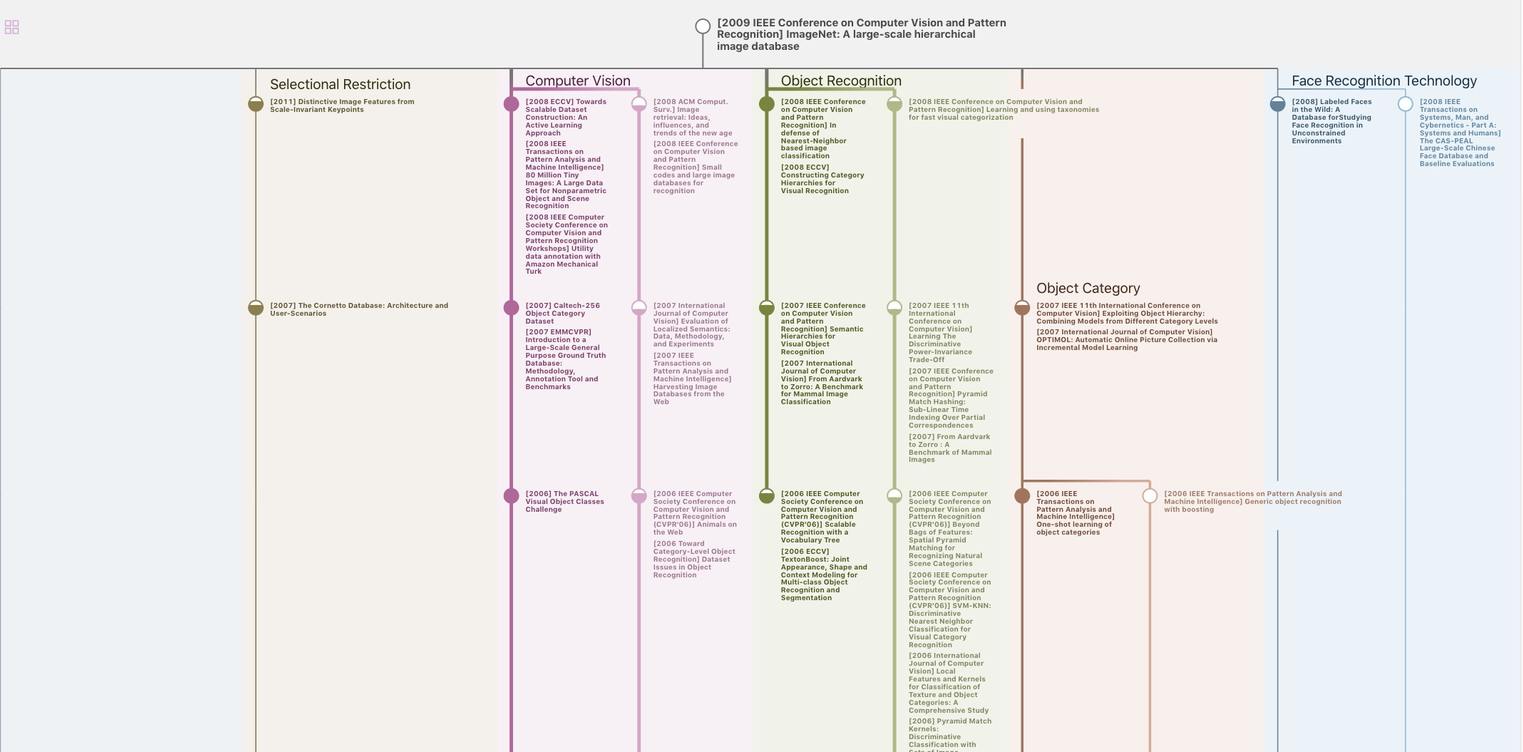
生成溯源树,研究论文发展脉络
Chat Paper
正在生成论文摘要