Direct Evaluation of Chain-of-Thought in Multi-hop Reasoning with Knowledge Graphs
Findings of the Association for Computational Linguistics ACL 2024(2024)
摘要
Large language models (LLMs) demonstrate strong reasoning abilities whenprompted to generate chain-of-thought (CoT) explanations alongside answers.However, previous research on evaluating LLMs has solely focused on answeraccuracy, neglecting the correctness of the generated CoT. In this paper, wedelve deeper into the CoT reasoning capabilities of LLMs in multi-hop questionanswering by utilizing knowledge graphs (KGs). We propose a noveldiscriminative and generative CoT evaluation paradigm to assess LLMs' knowledgeof reasoning and the accuracy of the generated CoT. Through experimentsconducted on 5 different families of LLMs across 2 multi-hop question-answeringdatasets, we find that LLMs possess sufficient knowledge to perform reasoning.However, there exists a significant disparity between answer accuracy andfaithfulness of the CoT reasoning generated by LLMs, indicating that they oftenarrive at correct answers through incorrect reasoning.
更多查看译文
AI 理解论文
溯源树
样例
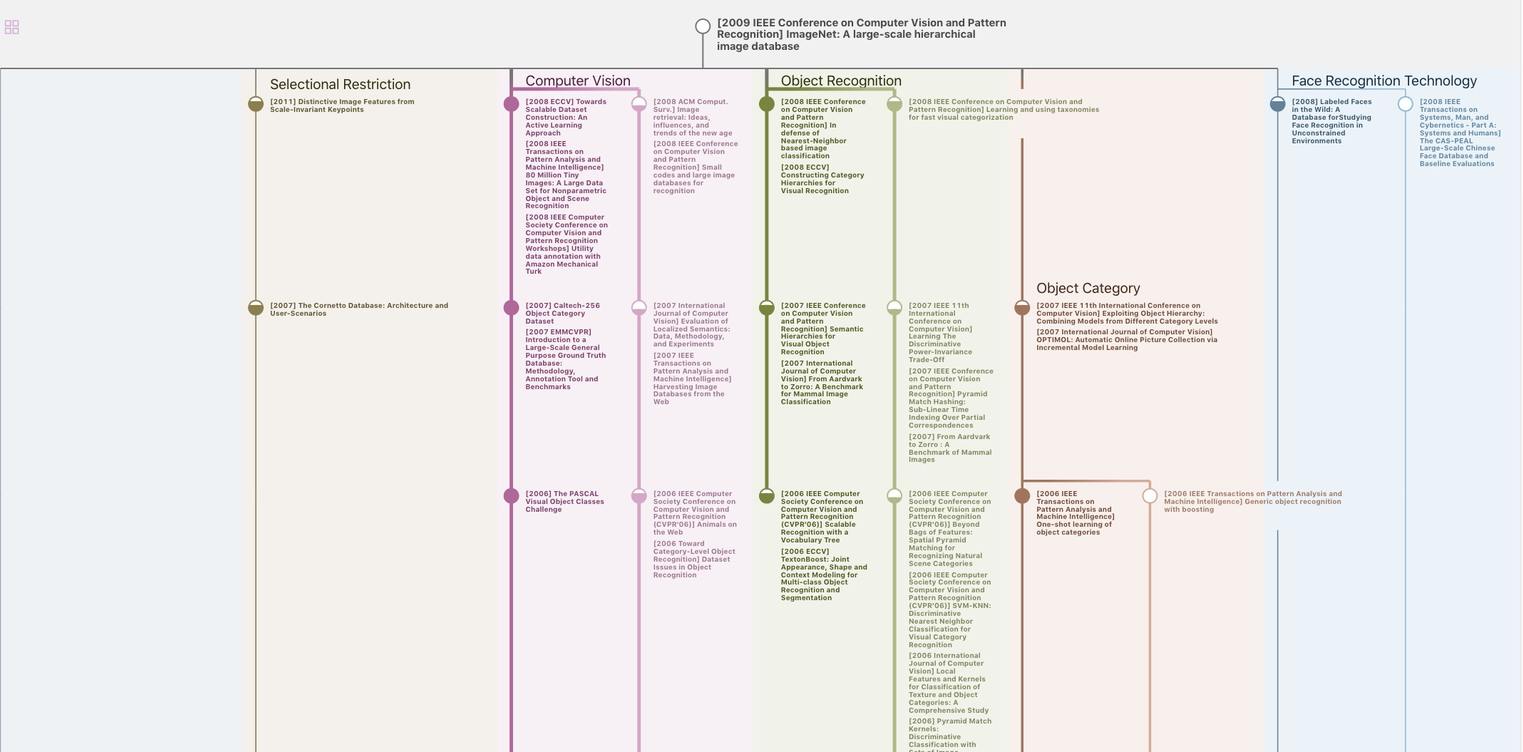
生成溯源树,研究论文发展脉络
Chat Paper
正在生成论文摘要