When LLMs Meet Cunning Questions: A Fallacy Understanding Benchmark for Large Language Models
CoRR(2024)
摘要
Recently, Large Language Models (LLMs) have made remarkable evolutions in
language understanding and generation. Following this, various benchmarks for
measuring all kinds of capabilities of LLMs have sprung up. In this paper, we
challenge the reasoning and understanding abilities of LLMs by proposing a
FaLlacy Understanding Benchmark (FLUB) containing cunning questions that are
easy for humans to understand but difficult for models to grasp. Specifically,
the cunning questions that FLUB focuses on mainly consist of the tricky,
humorous, and misleading questions collected from the real internet
environment. And we design three tasks with increasing difficulty in the FLUB
benchmark to evaluate the fallacy understanding ability of LLMs. Based on FLUB,
we investigate the performance of multiple representative and advanced LLMs,
reflecting our FLUB is challenging and worthy of more future study. Interesting
discoveries and valuable insights are achieved in our extensive experiments and
detailed analyses. We hope that our benchmark can encourage the community to
improve LLMs' ability to understand fallacies.
更多查看译文
AI 理解论文
溯源树
样例
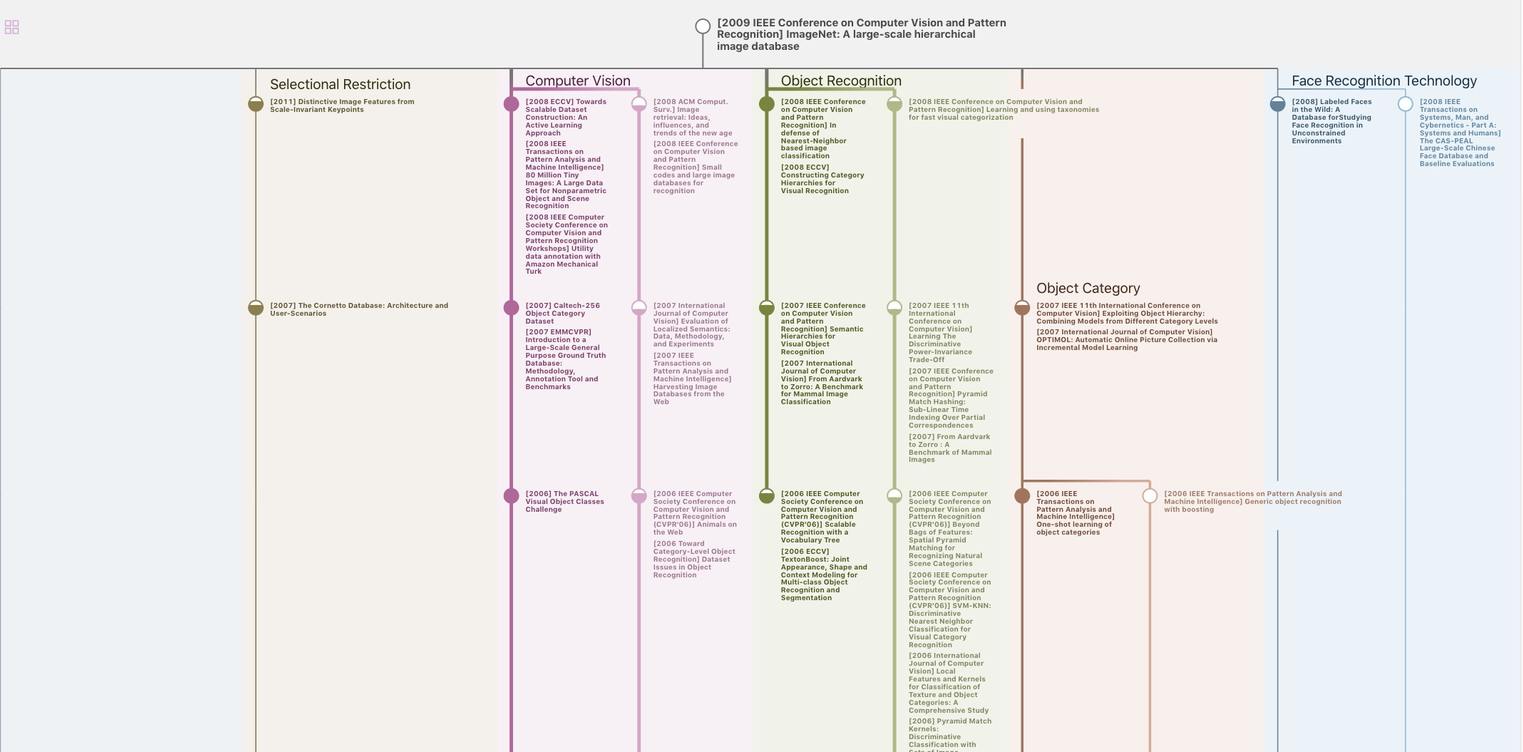
生成溯源树,研究论文发展脉络
Chat Paper
正在生成论文摘要