Intermediate-term memory mechanism inspired lightweight single image super resolution
Multimedia Tools and Applications(2024)
摘要
The essence of the Single Image Super Resolution (SISR) task revolves around learning and memorizing the mapping relationship between low-resolution and high-resolution images. Presently, prevailing SISR methods predominantly depend on simplistic long-range connections and intricate dense connections to establish long-term memory within the network. However, a notable limitation is the absence of a transitioning mechanism from short-term to long-term memory, impeding the smooth transfer of information. The intermediate-term memory mechanism assumes a critical role in the human memory system, acting as a vital bridge between short-term and long-term memory. Its ability to integrate fragmented neuronal information through specific intermediate-term connectivity patterns facilitates the formation of long-term memory. Inspired by this mechanism, we propose a deep feature extraction and fusion method based on intermediate-term connectivity, addressing the challenges associated with smooth information transfer between short-term and long-term memory. In this study, we introduce IMSR (Intermediate-term Memory Super Resolution), a lightweight model that efficiently aggregates features from different temporal and spatial levels within the network. By doing so, IMSR enhances the representation of both global and local information, contributing to improved performance. Additionally, we incorporate the Reconstructive Attention Mechanism (RAM) at the end of IMSR, allowing for precise tuning and fine-grained control of features to facilitate the integration of complementary information. Through extensive experimental evaluations, we demonstrate that IMSR surpasses other state-of-the-art lightweight models across four benchmark datasets, while maintaining a low parameter count and computational complexity.
更多查看译文
关键词
Super resolution,Recursive learning,Intermediate-term memory,Reconstructive attention mechanism,Lightweight model
AI 理解论文
溯源树
样例
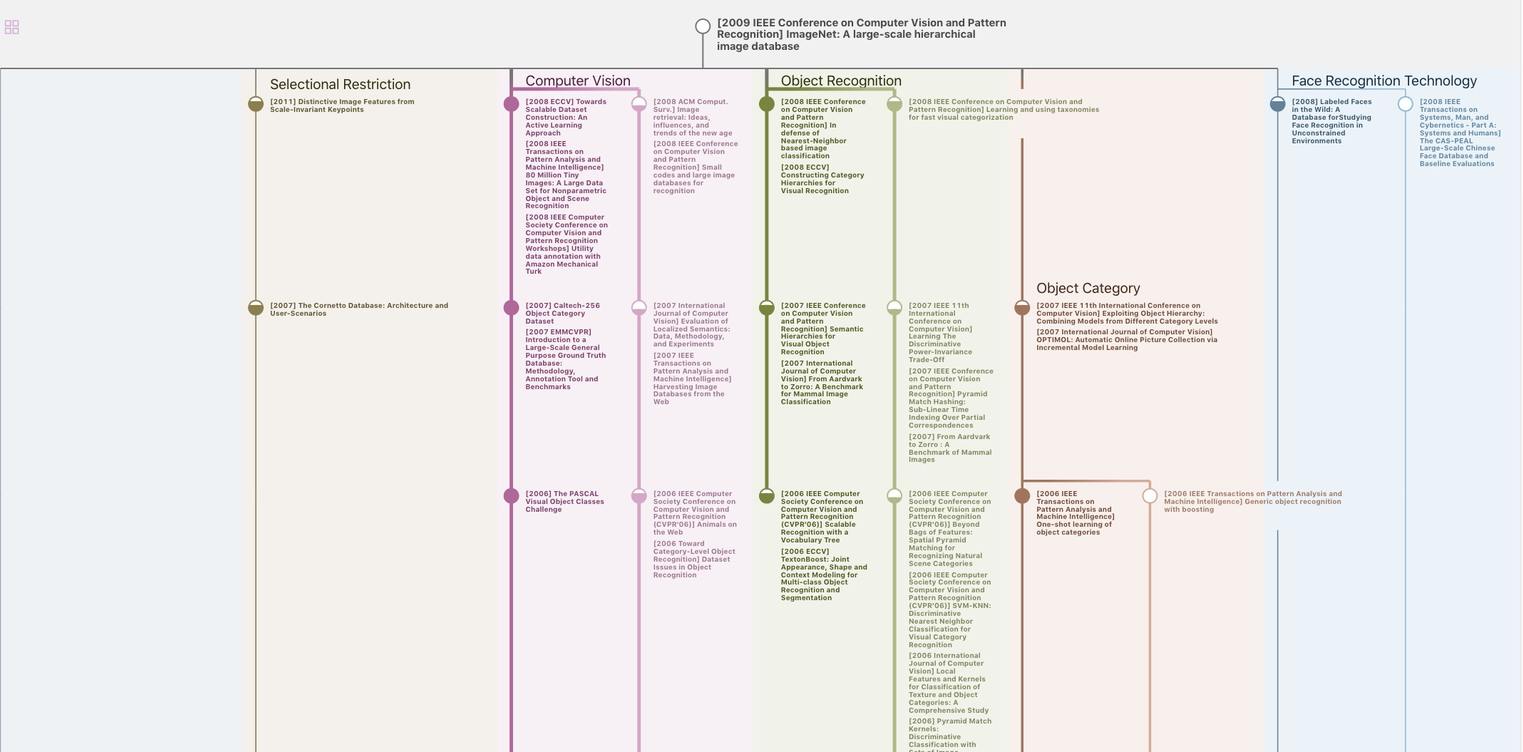
生成溯源树,研究论文发展脉络
Chat Paper
正在生成论文摘要