A Data-Driven Supervised Machine Learning Approach to Estimating Global Ambient Air Pollution Concentrations With Associated Prediction Intervals
CoRR(2024)
摘要
Global ambient air pollution, a transboundary challenge, is typically
addressed through interventions relying on data from spatially sparse and
heterogeneously placed monitoring stations. These stations often encounter
temporal data gaps due to issues such as power outages. In response, we have
developed a scalable, data-driven, supervised machine learning framework. This
model is designed to impute missing temporal and spatial measurements, thereby
generating a comprehensive dataset for pollutants including NO_2, O_3,
PM_10, PM_2.5, and SO_2. The dataset, with a fine granularity of
0.25^∘ at hourly intervals and accompanied by prediction intervals for
each estimate, caters to a wide range of stakeholders relying on outdoor air
pollution data for downstream assessments. This enables more detailed studies.
Additionally, the model's performance across various geographical locations is
examined, providing insights and recommendations for strategic placement of
future monitoring stations to further enhance the model's accuracy.
更多查看译文
AI 理解论文
溯源树
样例
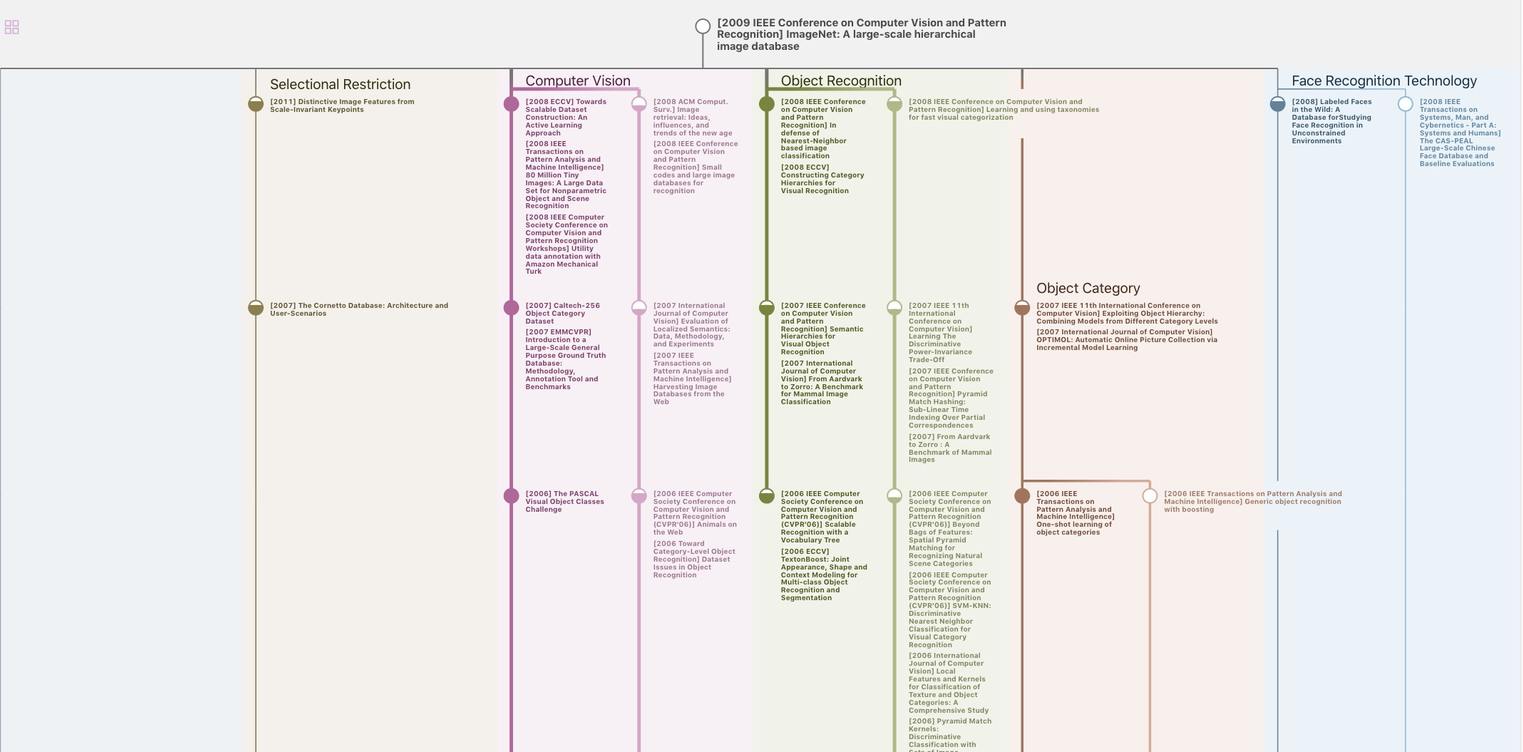
生成溯源树,研究论文发展脉络
Chat Paper
正在生成论文摘要