A novel model for fall detection and action recognition combined lightweight 3D-CNN and convolutional LSTM networks
Pattern Analysis and Applications(2024)
摘要
Three-dimensional convolutional neural networks (3D-CNNs) and full connection long short-term memory networks (FC-LSTMs) have been demonstrated as a kind of powerful non-intrusive approaches in fall detection. However, the feature extration of 3D-CNN-based requires a large-scale dataset. Meanwhile, the deployment of FC-LSTM to expand the input into one-dimension leads to the loss of spatial information. To this end, a novel model combined lightweight 3D-CNN and convolutional long short-term memory (ConvLSTM) networks is proposed in this paper. In this model, a lightweight 3D convolutional neural network with five layers is presented to avoid the phenomenon of over-fitting. To further explore the discrimnative features, the channel- and spatial-wise attention modules are adopted in each layer to improve the detection performance. In addition, the ConvLSTM is presented to extract the long-term spatial–temporal features of 3D tensors. Finally, we verify our model through extensive experiments by comprehensive comparisons with HMDB5, UCF11, URFD, and MCFD. Experimental results on the public benchmarks demonstrate that our method outperforms current state-of-the-art single-stream networks with 62.55 ± 7.99% on HMDB5, 97.28 ± 0.36% on UCF11, 98.06 ± 0.32% on URFD, and 94.84 ± 4.64% on MCFD.
更多查看译文
关键词
Fall detection,Action recognition,Three-dimensional CNN,Attention module,Single-stream network
AI 理解论文
溯源树
样例
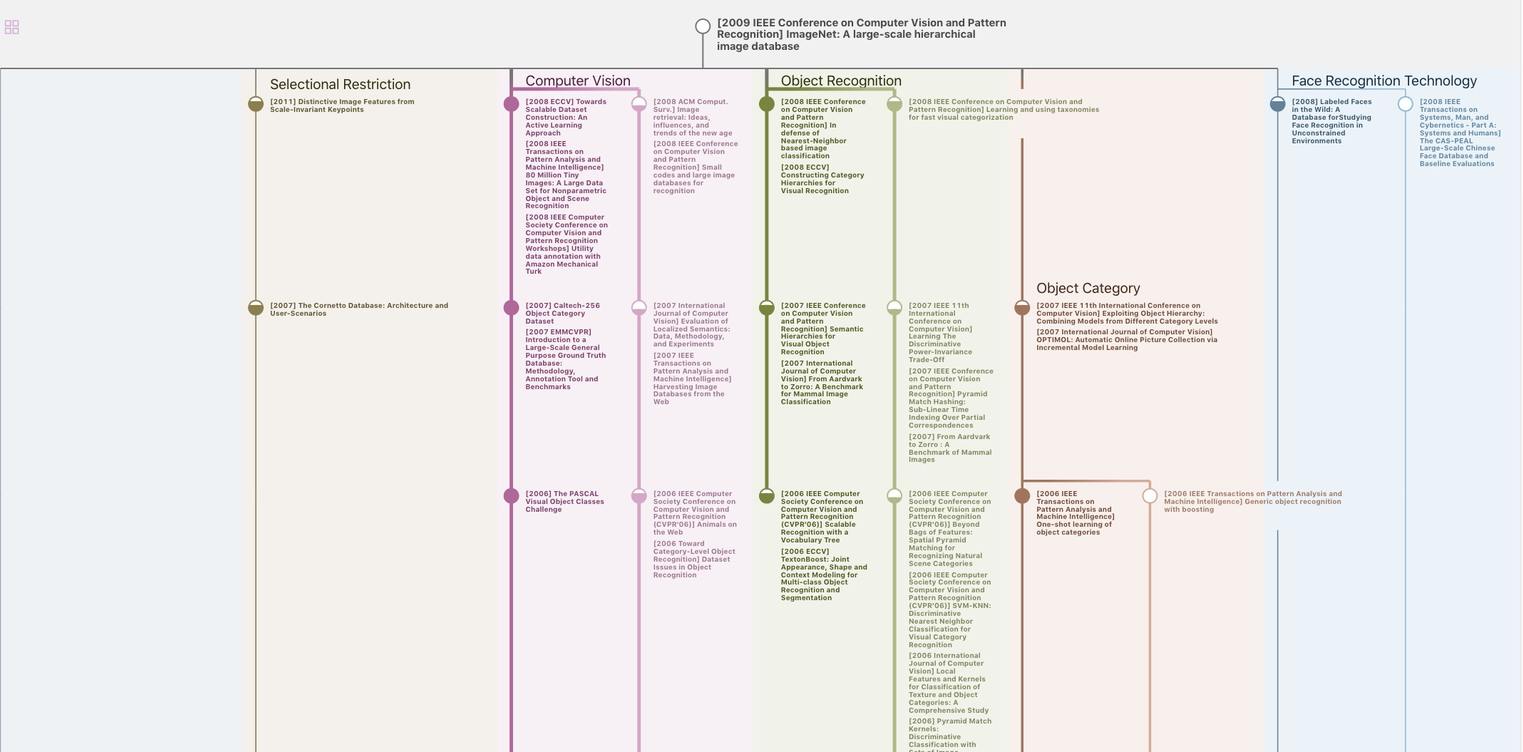
生成溯源树,研究论文发展脉络
Chat Paper
正在生成论文摘要