A new distributional treatment for time series anomaly detection
The VLDB Journal(2024)
摘要
Time series is traditionally treated with two main approaches, i.e., the time domain approach and the frequency domain approach. These approaches must rely on a sliding window so that time-shift versions of a sequence can be measured to be similar. Coupled with the use of a root point-to-point measure, existing methods often have quadratic time complexity. We offer the third ℝ domain approach. It begins with an insight that sequences in a stationary time series can be treated as sets of independent and identically distributed (iid) points generated from an unknown distribution in ℝ . This ℝ domain treatment enables two new possibilities: (a) The similarity between two sequences can be computed using a distributional measure such as Wasserstein distance (WD), kernel mean embedding or isolation distributional kernel ( 𝒦_I ), and (b) these distributional measures become non-sliding-window-based. Together, they offer an alternative that has more effective similarity measurements and runs significantly faster than the point-to-point and sliding-window-based measures. Our empirical evaluation shows that 𝒦_I is an effective and efficient distributional measure for time series; and 𝒦_I -based detectors have better detection accuracy than existing detectors in two tasks: (i) anomalous sequence detection in a stationary time series and (ii) anomalous time series detection in a dataset of non-stationary time series. The insight makes underutilized “old things new again” which gives existing distributional measures and anomaly detectors a new life in time series anomaly detection that would otherwise be impossible.
更多查看译文
关键词
Time series,Anomaly detection,Isolation kernel,Distributional kernel
AI 理解论文
溯源树
样例
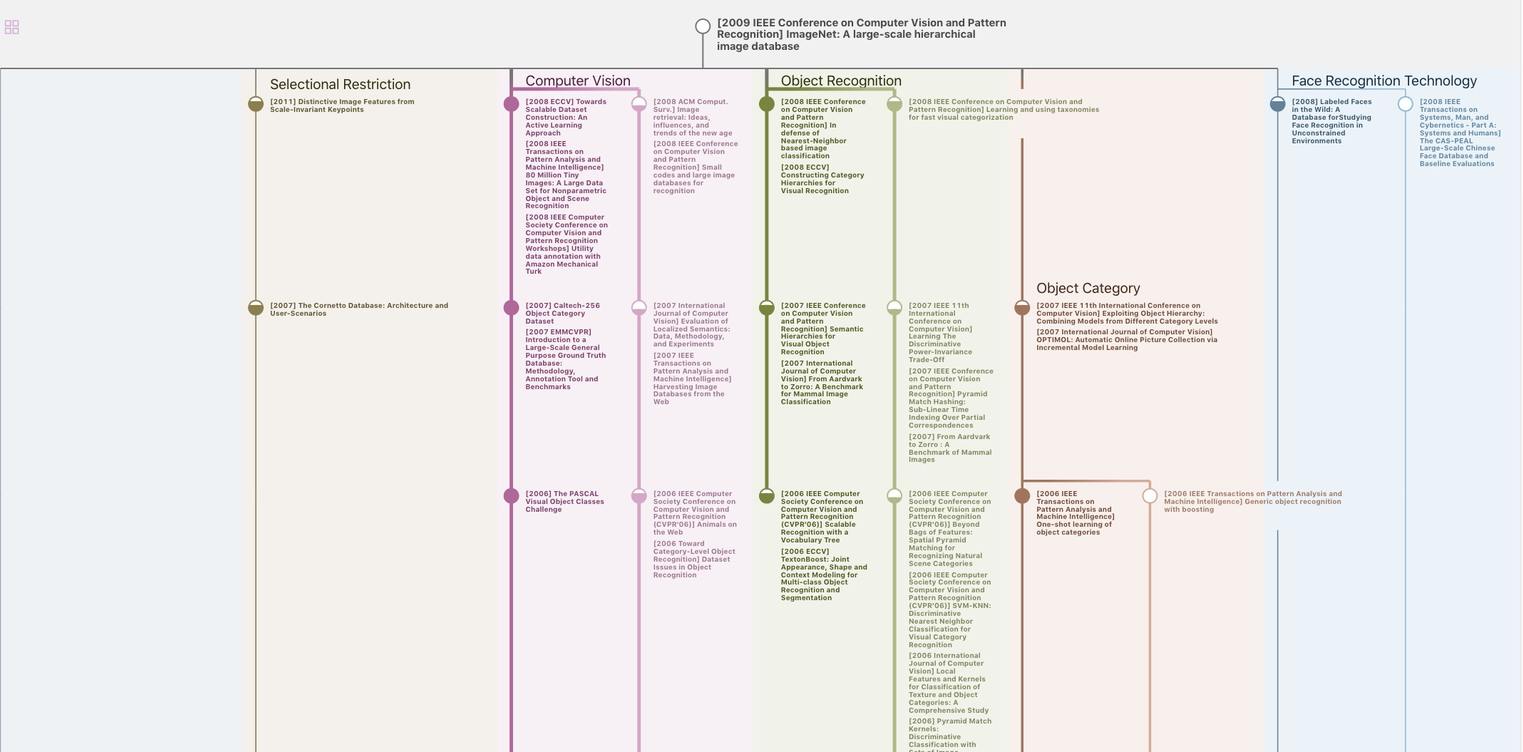
生成溯源树,研究论文发展脉络
Chat Paper
正在生成论文摘要