An Adaptive Spectral Clustering Algorithm for High-Dimensional Data
2023 6th International Conference on Software Engineering and Computer Science (CSECS)(2023)
摘要
As the samples are sparse in the high-dimensional space, the distance between the data samples cannot effectively represent their similarity. Consequently, the classical spectral clustering algorithm is limited in its effectiveness. The selection of the parameters for the Gaussian kernel function to measure similarity in the algorithm has a significant impact on the clustering results. To address the aforementioned problems, this paper proposes a novel algorithm called Relative Distance Adaptive Spectral Clustering (RDASC). The RDASC algorithm is specifically designed for high-dimensional data and is based on the concept of close distance. The algorithm is based on the Umap dimensionality reduction technique, which is used to visualize the similarity between data points in high-dimensional space. It achieves this by amplifying the differences between data points using the relative distance and integrating the Gaussian kernel function formulas based on both the relative and absolute distances. This allows for the calculation of a similarity measure between high-dimensional sample points. The scale parameter is determined based on the neighborhood distribution of the data points, resulting in an adaptive approach. The experimental results on the real UCI dataset show that the proposed algorithm is more effective than the traditional spectral clustering algorithm, with an average improvement of 4.38%, 7.37%, and 4.25% on the ACC, ARI, and NMI metrics, respectively.
更多查看译文
关键词
Spectral clustering,Adaptive,Scale parameter,Relative distance,High-dimensional data
AI 理解论文
溯源树
样例
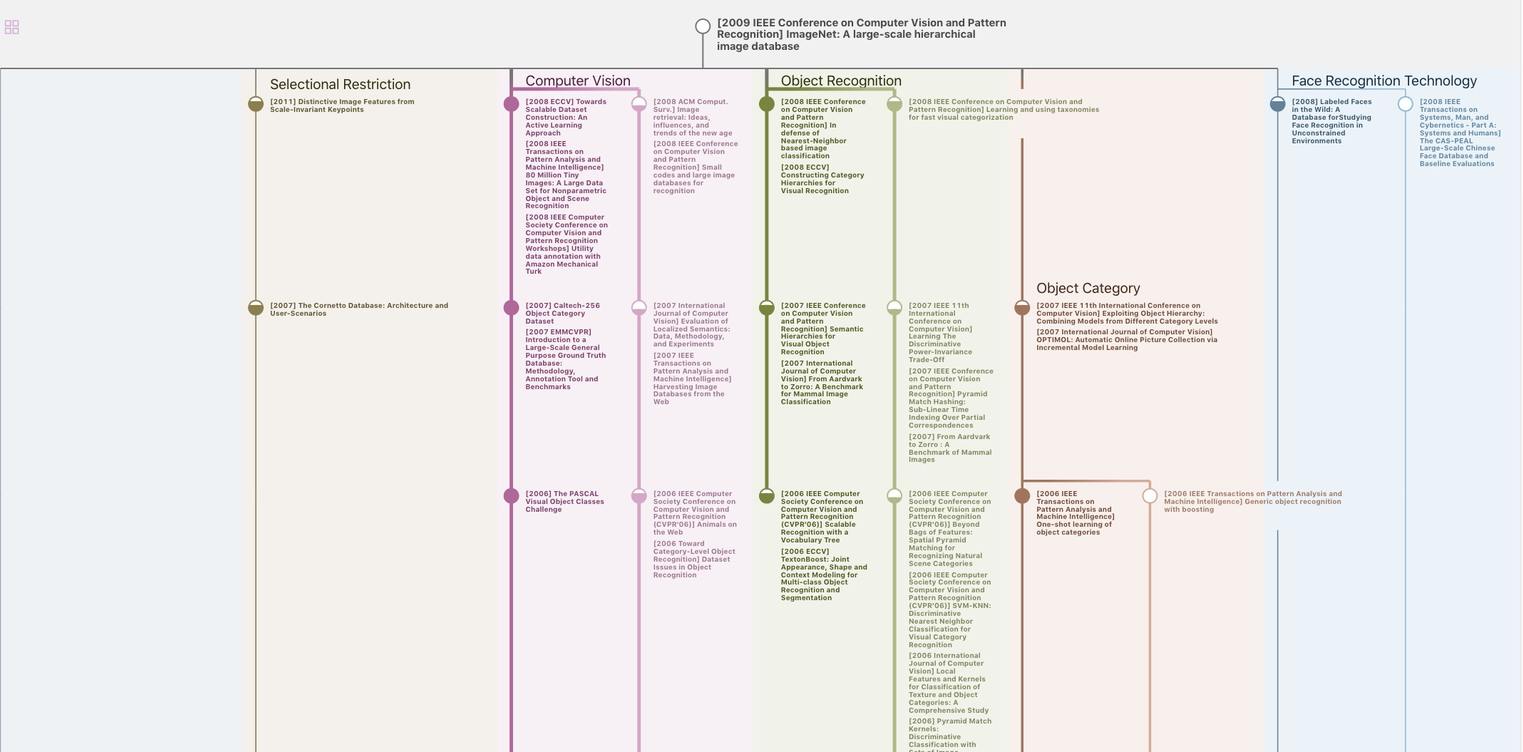
生成溯源树,研究论文发展脉络
Chat Paper
正在生成论文摘要