Combining Swin Transformer and Attention-Weighted Fusion for Scene Text Detection
Neural Processing Letters(2024)
摘要
The existing text detection algorithms based on Convolutional Neural Networks (CNN) commonly have the problems of insufficient receptive fields and inadequate extraction of spatial positional information, which limit their ability to detect large-scale variation text instances, long-distance and wide-spaced text instances as well as effectively distinguish complex background textures. To address the above problems, in this paper, a scene text detection algorithm combining Swin Transformer and attention-weighted fusion is proposed. Firstly, an attention-weighted fusion (AWF) module is proposed, which embeds a modified coordinate attention module (CAM) in the feature pyramid network (FPN). This module learns spatial positional weights of foreground information in different-scale features while suppressing redundant background information. As a result, the fused features are more focused on the text regions, enhancing the localization ability for text regions and boundaries. Secondly, the window-based self-attention mechanism of the Swin Transformer is utilized to achieve global feature perception on the fused features of the pyramid network. This compensates for the insufficient receptive fields of CNN and enhances the representation capability of global contextual features, thereby further improving the performance of text detection. Experimental results demonstrate that the proposed algorithm achieves competitive performance on three public datasets, namely ICDAR2015, MSRA-TD500, and Total-Text, with F-measure reaching 87.9%, 91.4%, and 86.7%, respectively. Code is available at: https://github.com/xgli411/ST-AWFNet .
更多查看译文
关键词
Scene text detection,Swin transformer,Attention-weighted fusion,Global feature perception
AI 理解论文
溯源树
样例
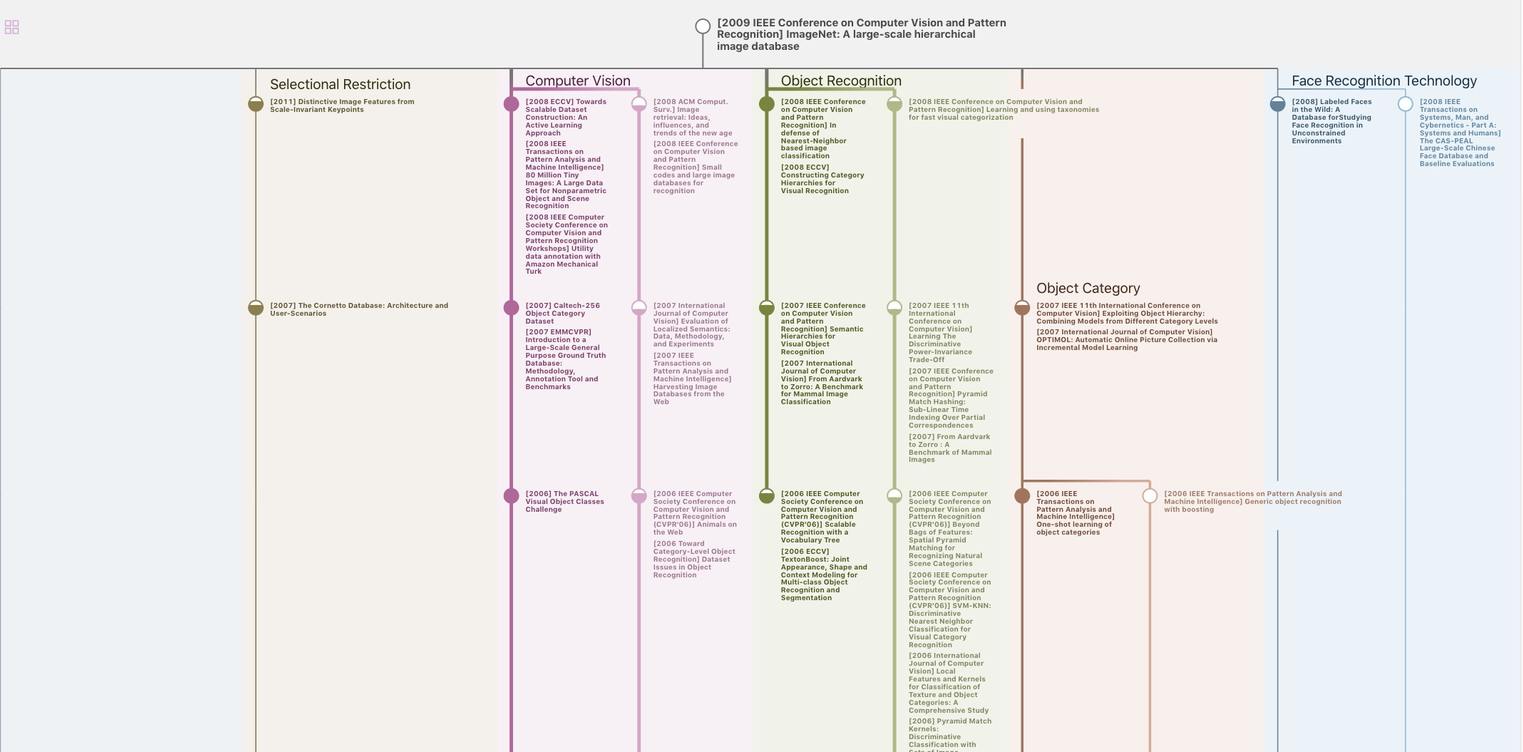
生成溯源树,研究论文发展脉络
Chat Paper
正在生成论文摘要