A Nested-Cascade Machine Learning Based Model for Intrusion Detection Systems
2023 IEEE CHILEAN Conference on Electrical, Electronics Engineering, Information and Communication Technologies (CHILECON)(2023)
摘要
In datasets, the preponderance of imbalanced classes impedes accurate cyberattack categorization. While high aggregate accuracy is sought, it's paramount to adeptly classify all attack types, especially the under-represented ones. Existing methodologies, such as Ensemble techniques and the Synthetic Minority Oversampling Technique (SMOTE), address these disparities, yet the dynamic nature of underrepresented cyberattacks in cybersecurity remains a concern. To address this, we introduce a nested cascade model tailored for diverse cyberattacks within imbalanced datasets. This model leverages binary classifiers across tiers, each targeting a specific attack type. Before initializing the cascade, SMOTE is applied to counterbalance class disparities. The cascade's classification sequence employs a dual strategy: an initial one-vs-all binary classifier approach for pending classes, followed by prioritization based on model performance. We assessed our approach using the UNSW-NB15 dataset. Preliminary results indicate approximately 80% efficiency across metrics like accuracy, recall, and Fl-score. Notably, SMOTE's in- tegration yielded significant improvements for underrepresented classes.
更多查看译文
关键词
Intrusion detection,imbalanced dataset,classification,cascading,machine learning,cybersecurity
AI 理解论文
溯源树
样例
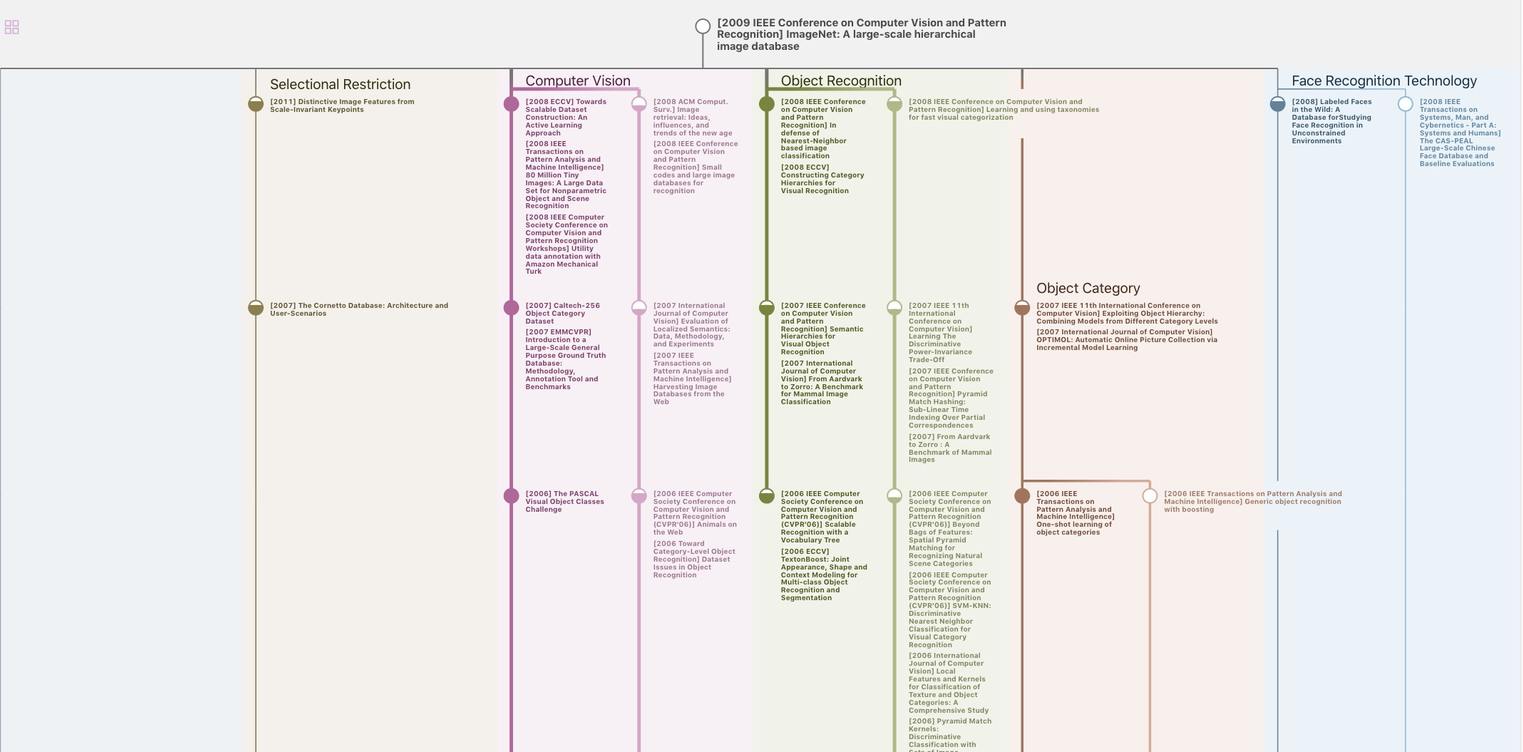
生成溯源树,研究论文发展脉络
Chat Paper
正在生成论文摘要