Predictive Method for Diabetes using Machine Learning
2023 International Conference on Computing, Communication, and Intelligent Systems (ICCCIS)(2023)
摘要
When the human body lacks sufficient insulin or when body cells become unresponsive to insulin, an excess of glucose remains in the blood. That can lead to serious health problems like renal disease, eyesight loss, and heart disease. Machine learning has the potential to anticipate diabetes. In this paper, the authors have used four classification algorithms: Support vector machine (SVM), K-nearest neighbors (KNN), Random Forest (RF), and Logistic Regression (LR), to predict diabetes and have conducted experiments using the PIMA Indians Diabetes Dataset (PIDD). SMOTE has been utilized to balance the dataset. Class balancing improved performance of classifiers. After implementing class balancing SVM obtained 78% accuracy, KNN obtained 74% accuracy, RF obtained 85% accuracy, and LR obtained 71 % accuracy.
更多查看译文
关键词
Diabetes,Support Vector Machine,Random Forest,Logistic Regression,K-nearest neighbors
AI 理解论文
溯源树
样例
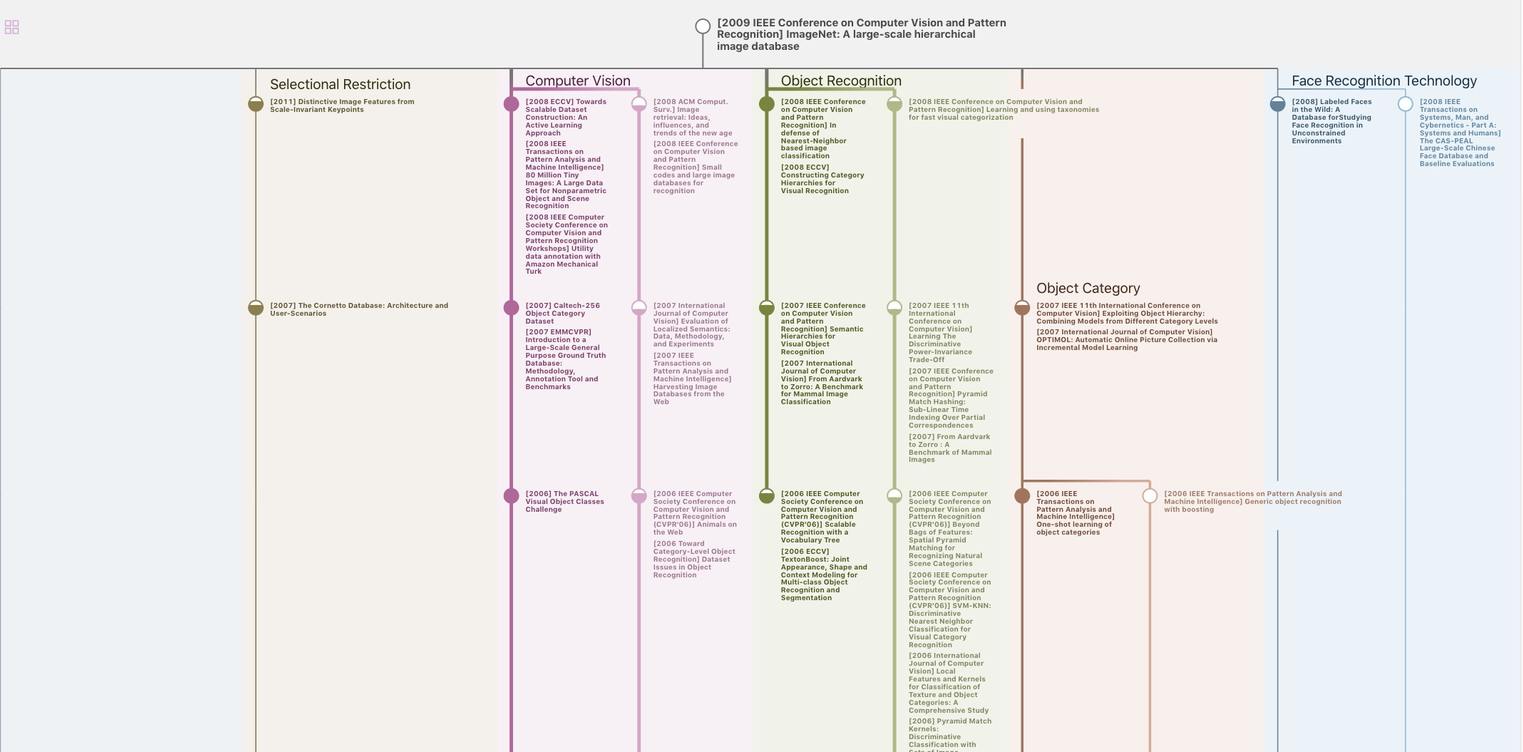
生成溯源树,研究论文发展脉络
Chat Paper
正在生成论文摘要