A novel granular decomposition based predictive modeling framework for cryptocurrencies' prices forecasting
CHINA FINANCE REVIEW INTERNATIONAL(2024)
摘要
PurposeOwing to highly volatile and chaotic external events, predicting future movements of cryptocurrencies is a challenging task. This paper advances a granular hybrid predictive modeling framework for predicting the future figures of Bitcoin (BTC), Litecoin (LTC), Ethereum (ETH), Stellar (XLM) and Tether (USDT) during normal and pandemic regimes.Design/methodology/approachInitially, the major temporal characteristics of the price series are examined. In the second stage, ensemble empirical mode decomposition (EEMD) and maximal overlap discrete wavelet transformation (MODWT) are used to decompose the original time series into two distinct sets of granular subseries. In the third stage, long- and short-term memory network (LSTM) and extreme gradient boosting (XGB) are applied to the decomposed subseries to estimate the initial forecasts. Lastly, sequential quadratic programming (SQP) is used to fetch the forecast by combining the initial forecasts.FindingsRigorous performance assessment and the outcome of the Diebold-Mariano's pairwise statistical test demonstrate the efficacy of the suggested predictive framework. The framework yields commendable predictive performance during the COVID-19 pandemic timeline explicitly as well. Future trends of BTC and ETH are found to be relatively easier to predict, while USDT is relatively difficult to predict.Originality/valueThe robustness of the proposed framework can be leveraged for practical trading and managing investment in crypto market. Empirical properties of the temporal dynamics of chosen cryptocurrencies provide deeper insights.
更多查看译文
关键词
Analytics,Forecasting,Cryptocurrency,Long and short-term memory network,Sequential quadratic programming
AI 理解论文
溯源树
样例
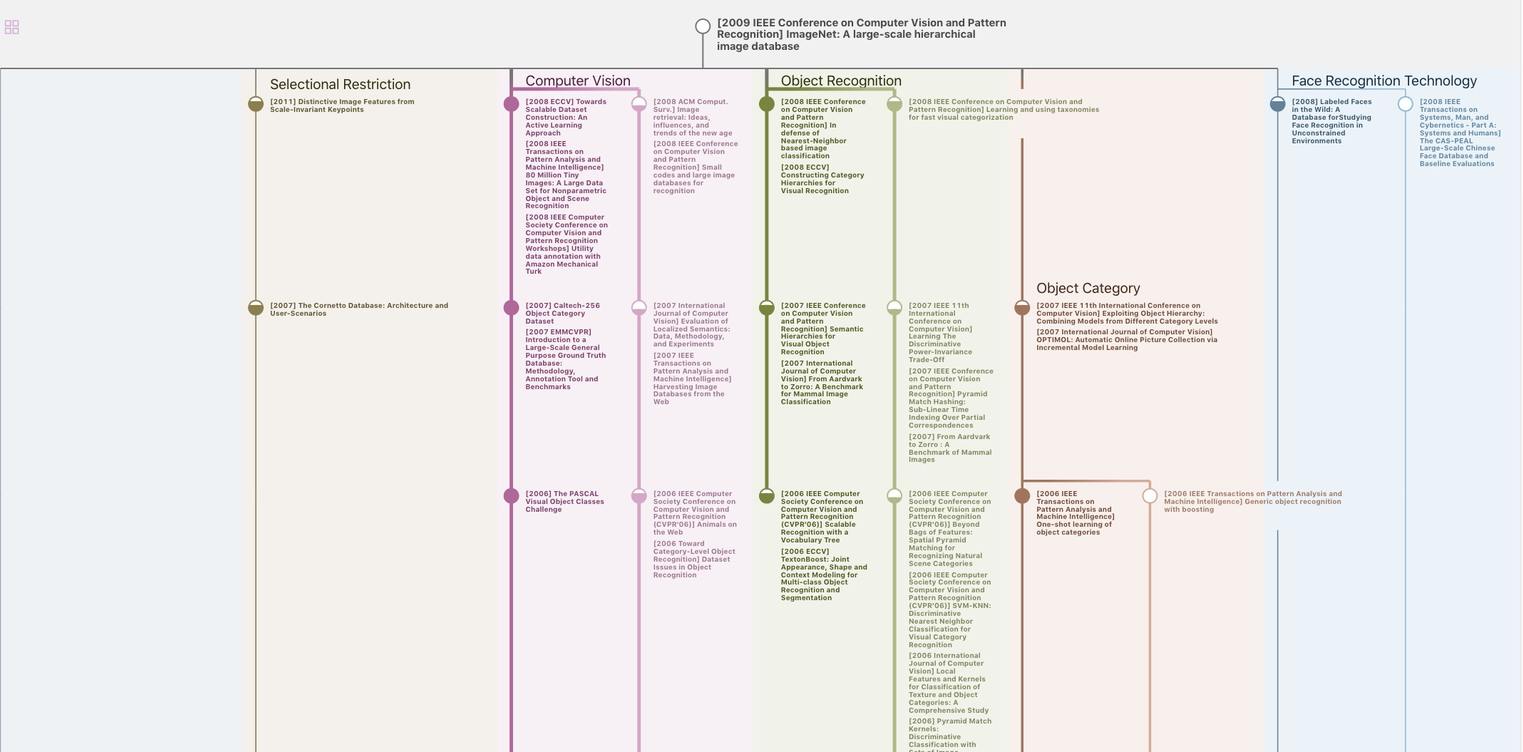
生成溯源树,研究论文发展脉络
Chat Paper
正在生成论文摘要