Joint torque prediction of industrial robots based on PSO-LSTM deep learning
INDUSTRIAL ROBOT-THE INTERNATIONAL JOURNAL OF ROBOTICS RESEARCH AND APPLICATION(2024)
摘要
PurposeBecause of the key role of joint torque in industrial robots (IRs) motion performance control and energy consumption calculation and efficiency optimization, the purpose of this paper is to propose a deep learning torque prediction method based on long short-term memory (LSTM) recurrent neural networks optimized by particle swarm optimization (PSO), which can accurately predict the the joint torque.Design/methodology/approachThe proposed model optimized the LSTM with PSO algorithm to accurately predict the IRs joint torque. The authors design an excitation trajectory for ABB 1600-10/145 experimental robot and collect its relative dynamic data. The LSTM model was trained with the experimental data, and PSO was used to find optimal number of LSTM nodes and learning rate, then a torque prediction model is established based on PSO-LSTM deep learning method. The novel model is used to predict the robot's six joint torque and the root mean error squares of the predicted data together with least squares (LS) method were comparably studied.FindingsThe predicted joint torque value by PSO-LSTM deep learning approach is highly overlapped with those from real experiment robot, and the error is quite small. The average square error between the predicted joint torque data and experiment data is 2.31 N.m smaller than that with the LS method. The accuracy of the novel PSO-LSTM learning method for joint torque prediction of IR is proved.Originality/valuePSO and LSTM model are deeply integrated for the first time to predict the joint torque of IR and the prediction accuracy is verified.
更多查看译文
关键词
Industrial robots,Particle swarm algorithm,Long short-term memory,Deep learning
AI 理解论文
溯源树
样例
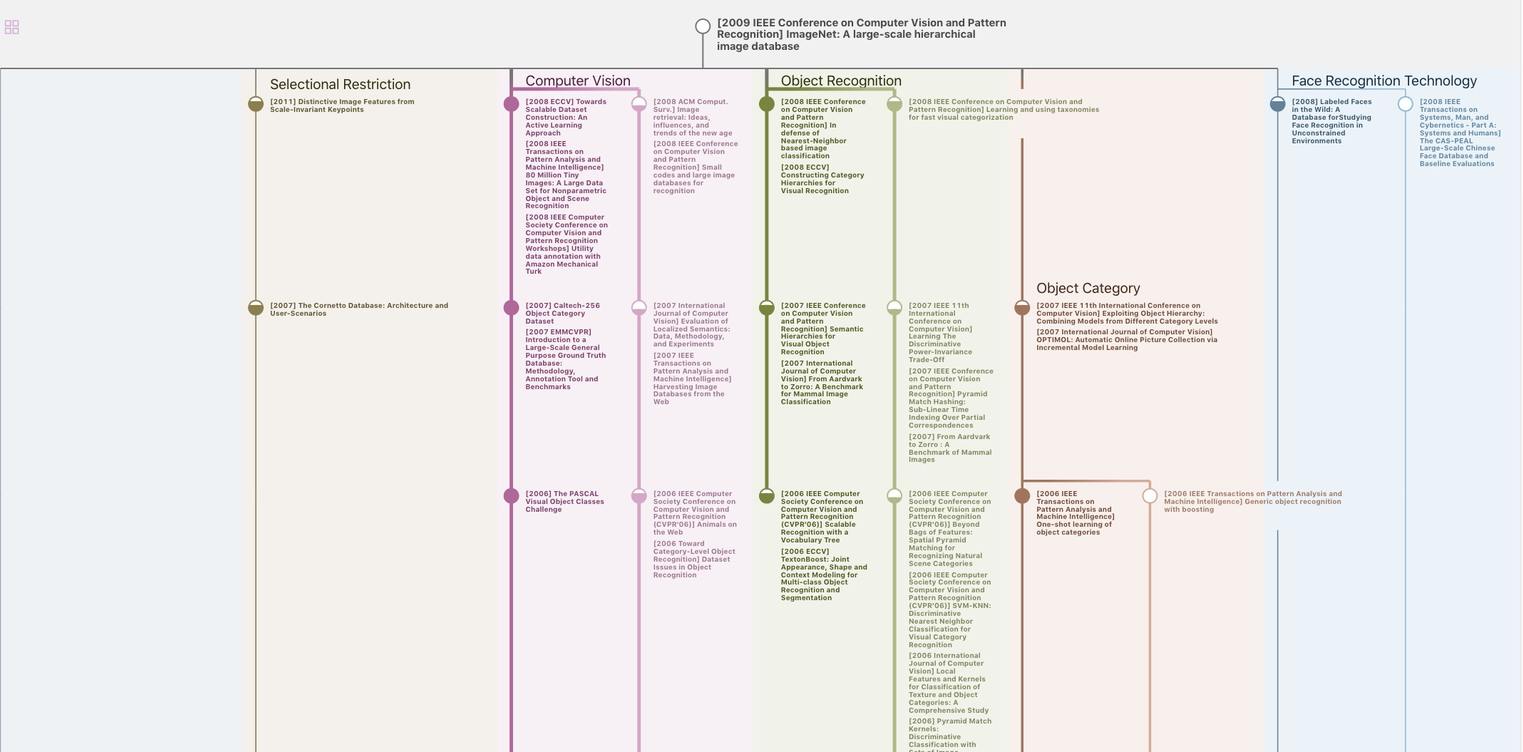
生成溯源树,研究论文发展脉络
Chat Paper
正在生成论文摘要