Variable Speed Limit Control for Mixed Traffic Flow on Highways Based on Deep-Reinforcement Learning
JOURNAL OF TRANSPORTATION ENGINEERING PART A-SYSTEMS(2024)
摘要
With the development of autonomous driving, a mixed traffic flow state composed of connected automated vehicles (CAVs) and human-driven vehicles (HVs) will last for an extended period. The abundant computing resources and CAVs with high compliance in the intelligent connected environment provide a good situation for variable speed limit control on highways, which helps even the traffic flow and improves traffic efficiency and safety. In this paper, we propose a variable speed limit control method for mixed traffic flow based on deep-reinforcement learning. First, the variable speed limit control problem is abstracted into a Markov decision process and the factors of real-time CAV penetration rates and predictions are considered in the state description. Different from variable message signs (VMS), CAVs are taken as the executive objects of the controller so that the variable speed limit control for mixed traffic flow is realized indirectly through the interaction with HVs. Next, double deep Q network (DDQN) is introduced to calculate the optimal speed limit in different states. Finally, the empirical study on US101-S proves the effectiveness of the proposed model. The results show that the variable speed limit control model based on the DDQN algorithm can effectively improve the efficiency and environmental benefits of mixed traffic flow. Moreover, the multi-objective reward function can achieve a better control effect than the single objective. Besides, the proposed model outperforms other models in this paper and predictive factors can further improve proactive control performance. In addition, with the increasing penetration of CAV, the proposed model achieves a better control effect.
更多查看译文
关键词
Variable speed limit (VSL),Connected and automated vehicles (CAVs),Reinforcement learning,Mixed traffic flow
AI 理解论文
溯源树
样例
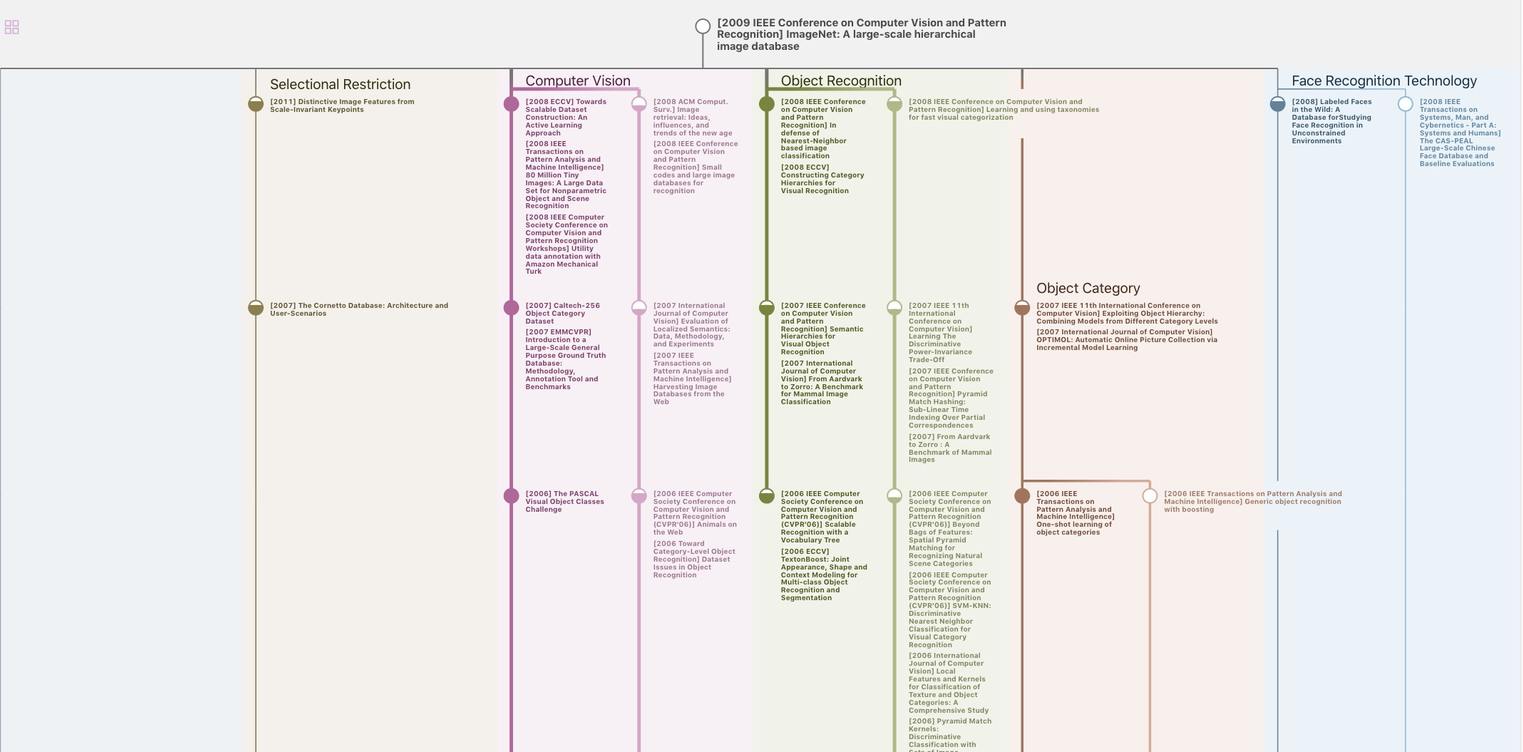
生成溯源树,研究论文发展脉络
Chat Paper
正在生成论文摘要