Integrating Recurrent Neural Networks with Convolutional Neural Networks for Enhanced Traffic Light Detection and Tracking
TRAITEMENT DU SIGNAL(2023)
摘要
Detecting and tracking traffic lights within an urban landscape, from a camera affixed to a moving vehicle, poses a substantial challenge. It necessitates the development of a reliable traffic light detection and tracking system that strikes an optimal balance between precision and real-time processing capabilities. Driven by this imperative, this study puts forward a novel traffic light detection methodology that harnesses the synergistic power of convolutional neural networks (CNN) and recurrent neural networks (RNN). The CNN, renowned for its self-learning capability, is employed for effective feature extraction, while the RNN is leveraged to retain information from video frames, thereby facilitating more reliable predictions. These two types of neural networks are amalgamated into a singular, expansive neural network, working in unison. The input video is initially processed through the CNN, leading to the extraction of spatial features. Subsequently, it is fed into the RNN for the extraction of temporal features. The final determination of the traffic light state is based on the combined usage of these extracted features. The addition of temporal features significantly bolsters overall performance without escalating computational complexity. The proposed methodology was trained and evaluated using two distinct datasets: the DriveU Traffic Light Dataset and the Bosch Small Traffic Lights Dataset, both of which include video frames captured by a camera mounted on a moving vehicle. Demonstrating high detection precision while operating in real-time, the proposed approach exhibits significant potential for practical application.
更多查看译文
关键词
convolutional neural network,recurrent neural network,traffic light detection and tracking,mobile systems
AI 理解论文
溯源树
样例
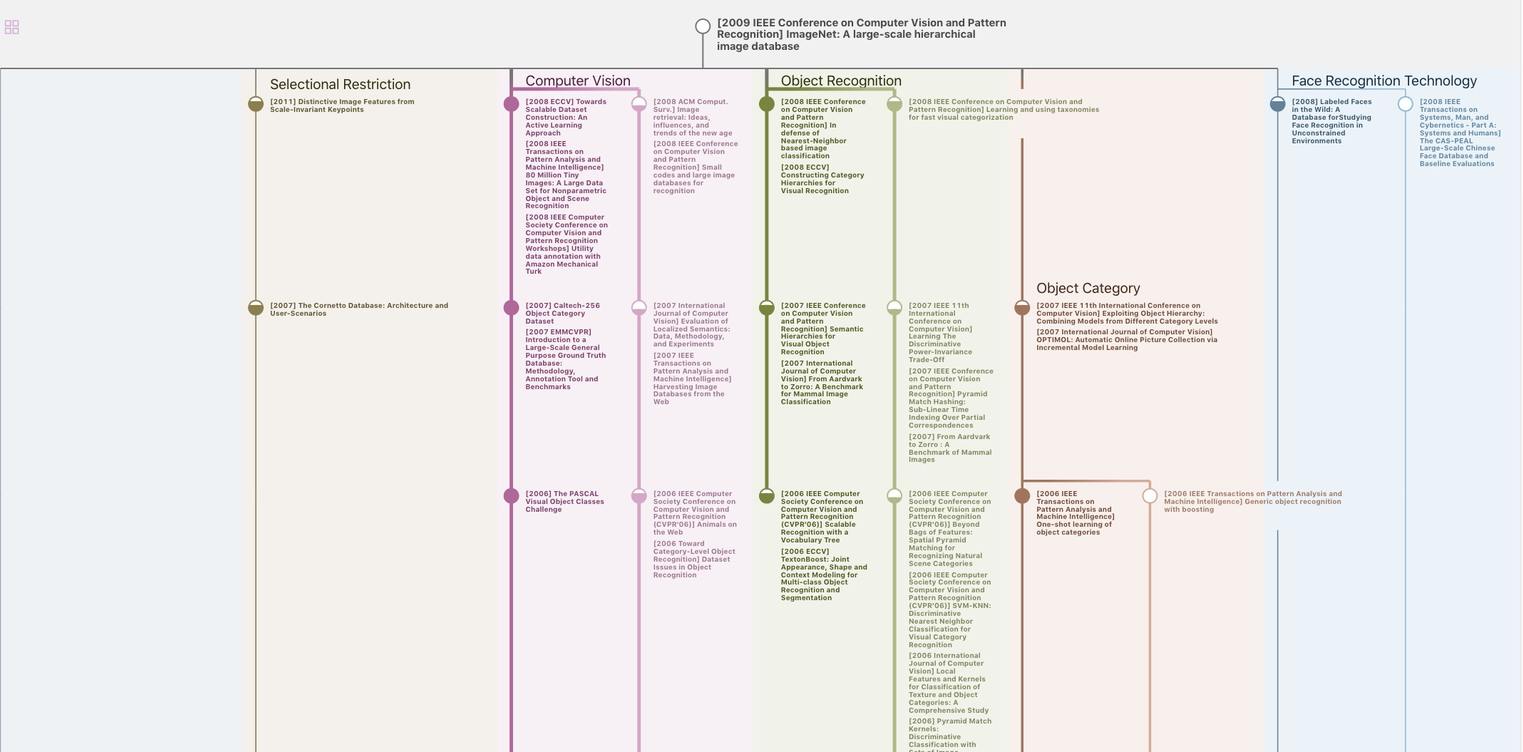
生成溯源树,研究论文发展脉络
Chat Paper
正在生成论文摘要