CombineDeepNet: A Deep Network for Multistep Prediction of Near-Surface PM2.5 Concentration
IEEE JOURNAL OF SELECTED TOPICS IN APPLIED EARTH OBSERVATIONS AND REMOTE SENSING(2024)
摘要
PM2.5 is a type of air pollutant that can cause respiratory and cardiovascular problems. Precise PM2.5 ( mu g/m(3) ) concentration prediction may help reduce health concerns and provide early warnings. To better understand air pollution, a number of approaches have been presented for predicting PM2.5 concentrations. Previous research used deep learning models for hourly predictions of air pollutants due to their success in pattern recognition, however, these models were unsuitable for multisite, long-term predictions, particularly in regard to the correlation between pollutants and meteorological data. This article proposes the combine deep network (CombineDeepNet), which combines multiple deep networks, including a bidirectional long short-term memory, bidirectional gated recurrent units, and a shallow model represented by fully connected layers, to create a hybrid forecasting system. It can effectively capture the complex relationships between air pollutants and various influencing factors to predict hourly PM2.5 concentrations in multiple monitoring sites based in China. The best root mean square error achieved was 22.0 mu g/m(3) (long-term) and 6.2 mu g/m(3) (short-term), with mean absolute error values of 3.4 mu g/m(3) (long-term) and 2.2 mu g/m(3) (short-term). In addition, the correlation coefficient (R-2 ) reached 0.96 (long-term) and 0.83 (short-term) across six monitoring sites. These results demonstrate that CombineDeepNet enhances prediction accuracy compared with popular deep learning methods. Therefore, CombineDeepNet proves to be a important framework for predicting PM2.5 concentration.
更多查看译文
关键词
Predictive models,Atmospheric modeling,Logic gates,Feature extraction,Deep learning,Air pollution,Weather forecasting,bidirectional gated recurrent units (BiGRU),bidirectional long short-term memory (BiLSTM),prediction
AI 理解论文
溯源树
样例
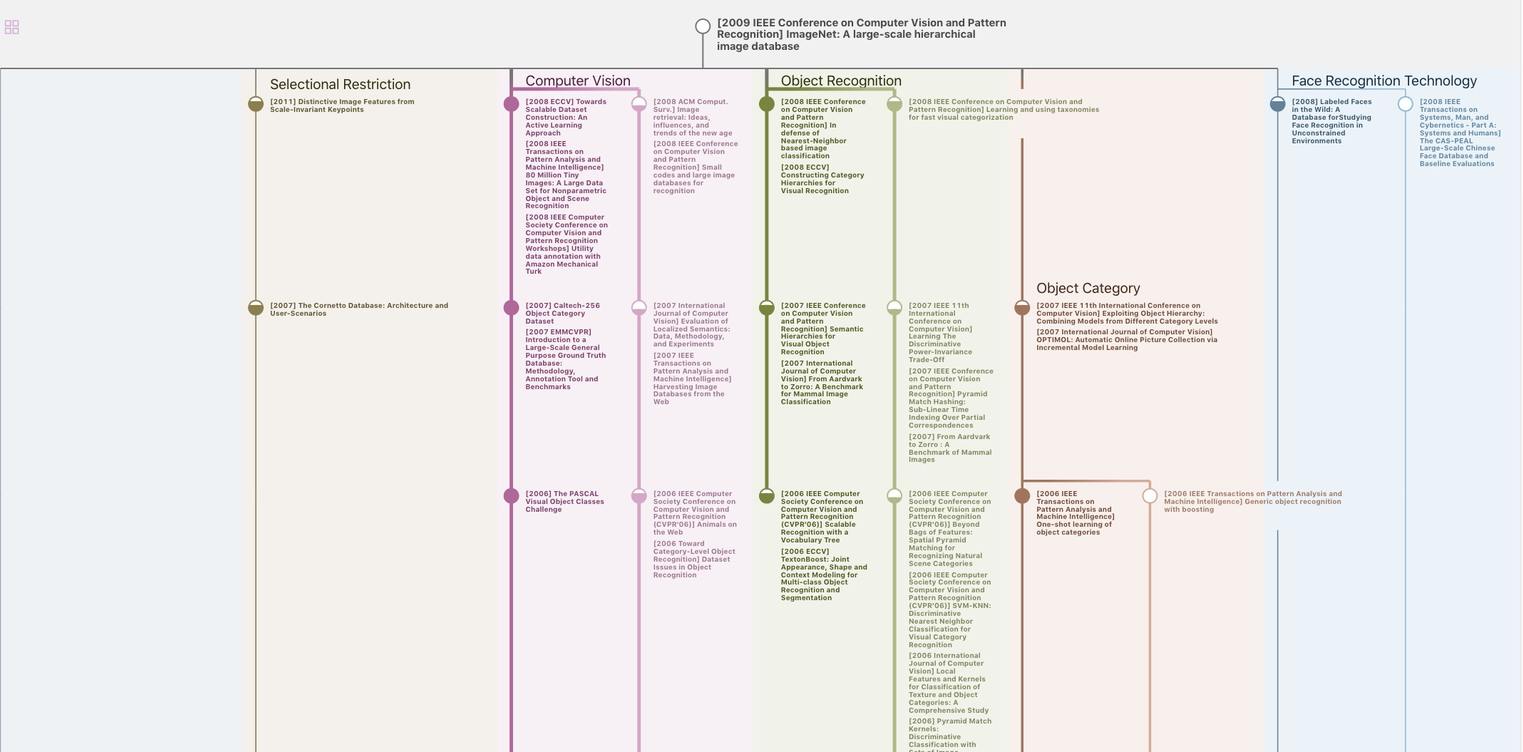
生成溯源树,研究论文发展脉络
Chat Paper
正在生成论文摘要