AML-Net: Attention-based Multi-Scale Lightweight Model for Brain Tumour Segmentation in Internet of Medical Things
CAAI TRANSACTIONS ON INTELLIGENCE TECHNOLOGY(2024)
摘要
Brain tumour segmentation employing MRI images is important for disease diagnosis, monitoring, and treatment planning. Till now, many encoder-decoder architectures have been developed for this purpose, with U-Net being the most extensively utilised. However, these architectures require a lot of parameters to train and have a semantic gap. Some work tried to make a lightweight model and do channel pruning that made a small receptive field which compromised the accuracy. The authors propose an attention-based multi-scale lightweight model called AML-Net in Internet of Medical Things to overcome the above issues. This model consists of three small encoder-decoder architectures that are trained with different scale input images along with previously learned features to diminish the loss. Moreover, the authors designed an attention module which replaced the traditional skip connection. For the attention module, six different experiments were conducted, from which dilated convolution with spatial attention performed well. This attention module has three dilated convolutions which make a relatively large receptive field followed by spatial attention to extract global context from encoder low-level features. Then these fine features are combined with the decoder's same layer of high-level features. The authors perform the experiment on a low-grade-glioma dataset provided by the Cancer Genome Atlas which has at least Fluid-Attenuated Inversion Recovery modality. The proposed model has 1/43.4, 1/30.3, 1/28.5, 1/20.2 and 1/16.7 fewer parameters than Z-Net, U-Net, Double U-Net, BCDU-Net and CU-Net respectively. Moreover, the authors' model gives results with IoU = 0.834, F1-score = 0.909 and sensitivity = 0.939, which are greater than U-Net, CU-Net, RCA-IUnet and PMED-Net.
更多查看译文
关键词
artificial intelligence,machine learning,medical image processing,medical signal processing
AI 理解论文
溯源树
样例
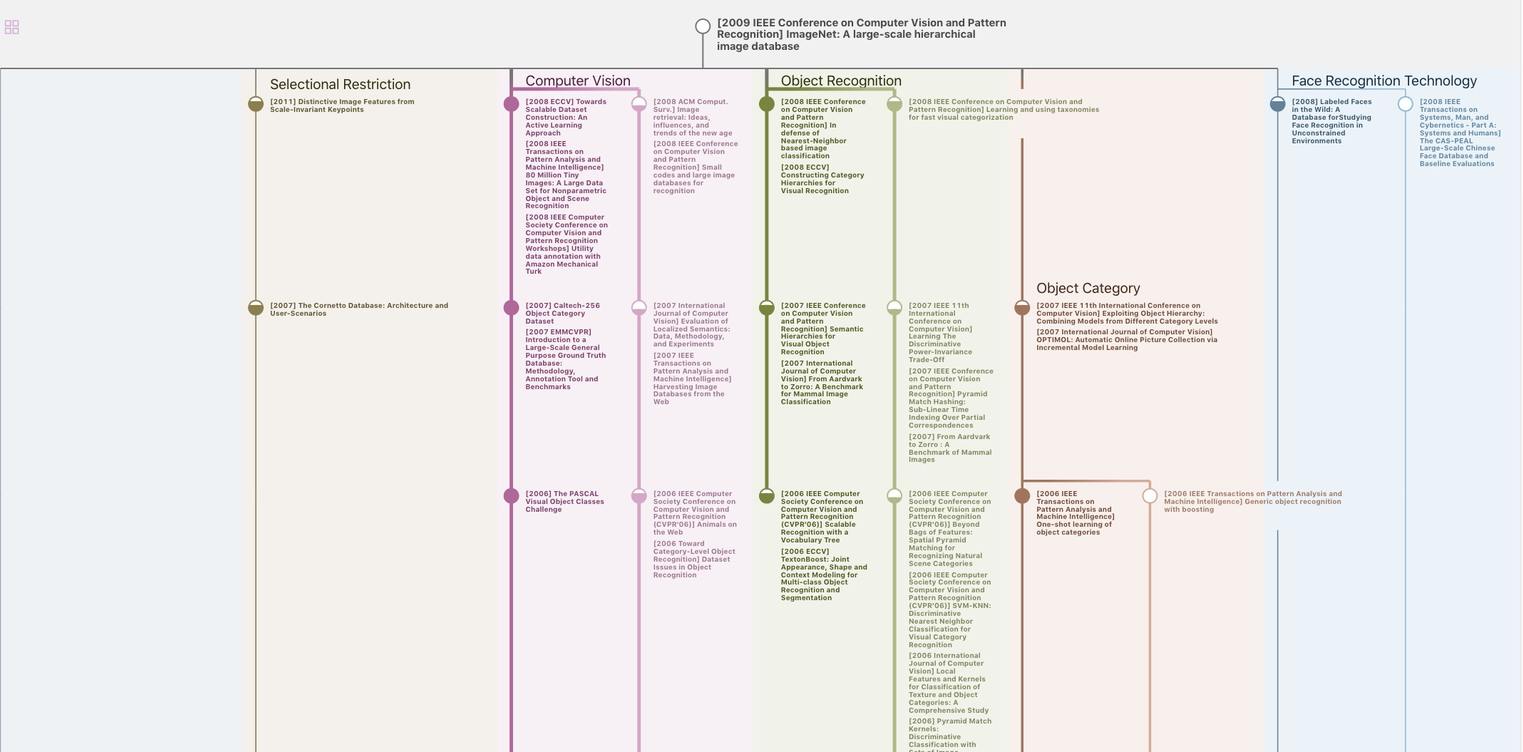
生成溯源树,研究论文发展脉络
Chat Paper
正在生成论文摘要