UANet: An Uncertainty-Aware Network for Building Extraction From Remote Sensing Images
IEEE TRANSACTIONS ON GEOSCIENCE AND REMOTE SENSING(2024)
摘要
Building extraction aims to segment building pixels from remote sensing (RS) images and plays an essential role in many applications, such as city planning and urban dynamic monitoring. Over the past few years, deep learning methods with encoder-decoder architectures have achieved remarkable performance due to their powerful feature representation capability. Nevertheless, due to the varying scales and styles of buildings, conventional deep learning models always suffer from uncertain predictions and cannot accurately distinguish the complete footprints of the building from the complex distribution of ground objects, leading to a large degree of omission and commission. In this article, we realize the importance of uncertain prediction and propose a novel and straightforward uncertainty-aware network (UANet) to alleviate this problem. Specifically, we first apply a general encoder-decoder network to obtain a building extraction map with relatively high uncertainty. Second, in order to aggregate the useful information in the highest-level features, we design a prior information guide module (PIGM) to guide the highest-level features in learning the prior information from the conventional extraction map. Third, based on the uncertain extraction map, we introduce an uncertainty rank algorithm (URA) to measure the uncertainty level of each pixel belonging to the foreground and the background. We further combine this algorithm with the proposed uncertainty-aware fusion module (UAFM) to facilitate level-by-level feature refinement and obtain the final refined extraction map with low uncertainty. To verify the performance of our proposed UANet, we conduct extensive experiments on three public building datasets, including the WHU building dataset, the Massachusetts building dataset, and the Inria aerial image dataset. Results demonstrate that the proposed UANet outperforms other state-of-the-art (SOTA) algorithms by a large margin. The source code of the proposed UANet is available at https://github.com/Henryjiepanli/Uncertainty-aware-Network.
更多查看译文
关键词
Building extraction,remote sensing (RS),uncertainty-aware
AI 理解论文
溯源树
样例
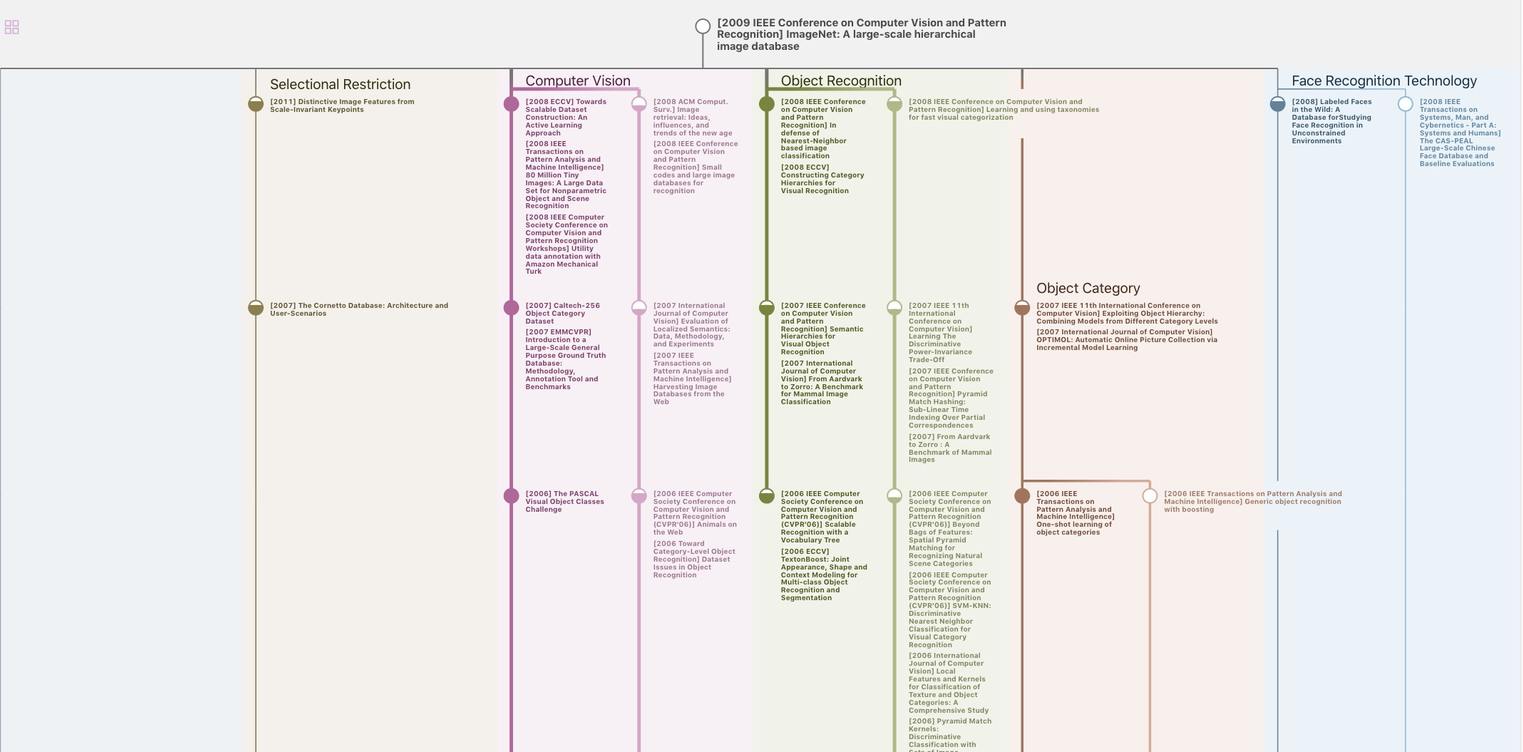
生成溯源树,研究论文发展脉络
Chat Paper
正在生成论文摘要